Tackling business problems with data science
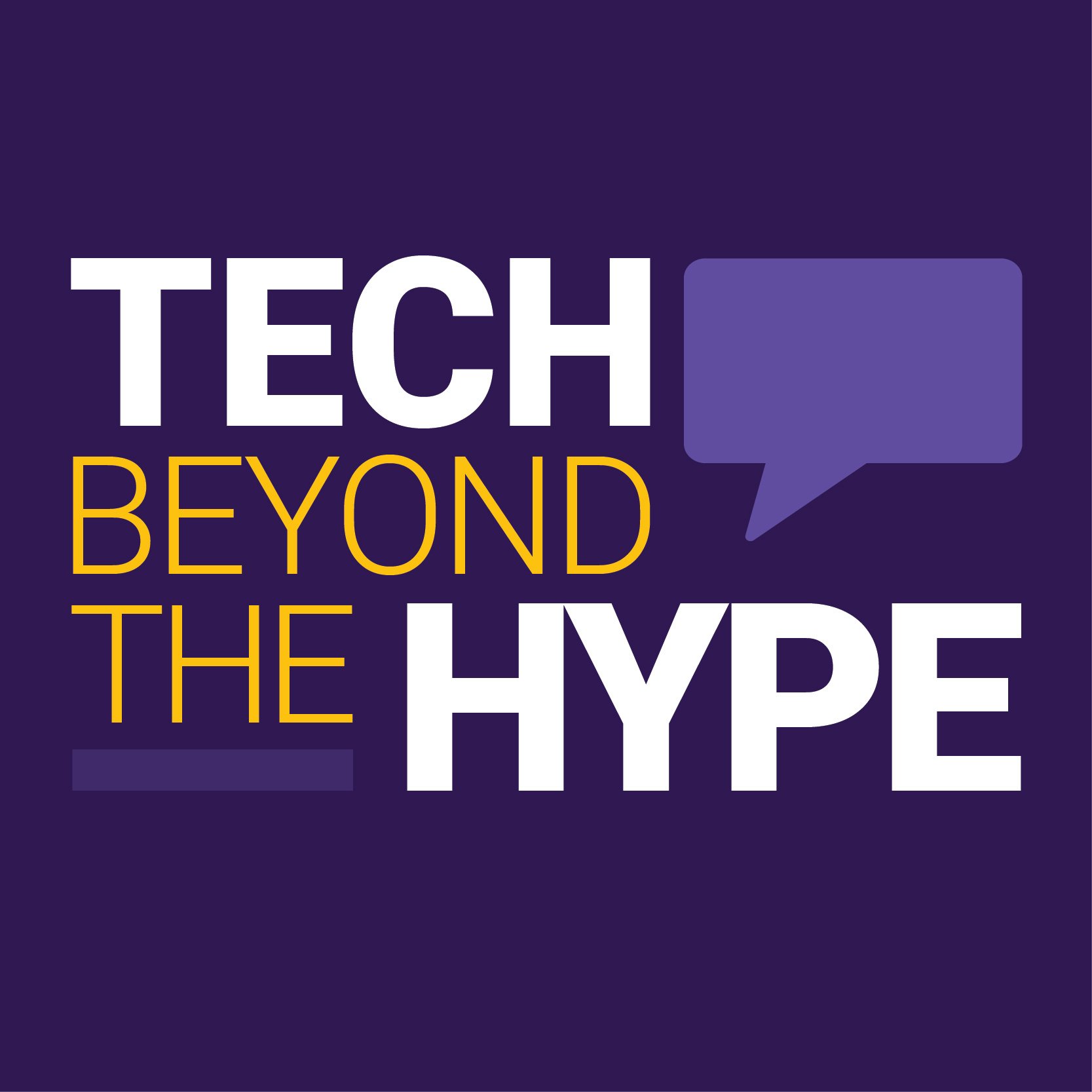
Where's data science heading? Hear how companies are using it after the pandemic, what organizations can do to foster a more diverse data science team and future trends.
In our latest original podcast series, Tech Beyond the Hype, host Ana Salom Boira is on a mission to make sense of all the latest business and tech hypes. Join her every month as she sits down with experts and leaders from across business and tech to find out how emerging technologies and new ways of working are shaping the future of how work gets done.
In the first episode of Tech Beyond the Hype, Ana talks to Sandi Ganguly, principal data science group manager at Microsoft, about the data science role in the digital future of work.
The exponential growth of data, estimated to reach 175 zettabytes by 2025, highlights the importance of data literacy and data management for the success of AI projects and data science initiatives. Despite the rapid growth of data, many leaders outside the data teams lack the necessary data literacy skills to take an active role in the design and deployment of AI initiatives, putting data science teams under intense pressure to deliver on an ever-increasing list of objectives.
In this episode, Sandi discusses how the influx of data is causing a proliferation in data-related roles and why business leaders need to encourage data literacy across all functions to improve data management and get valuable, real-time insights that drive the business toward the future.
Tune in to the full episode of Tech Beyond the Hype to learn more about the role of data science in the digital future of work and gain valuable insights on how to use data to drive business success. Subscribe to the series wherever you get your podcasts to stay up to date with the latest trends and innovations in business and tech.
Ana: [00:00:00] This is Tech Beyond the Hype, a TechTarget original podcast about the future that looks at how today's business trends impact the future of work. My name is Ana Salom Boira and I'm on a mission to make sense of how different sectors are evolving with and adapting to the proliferation of advanced technologies like artificial intelligence and blockchain. This episode is all about data science.
Ana: Businesses today are harvesting more data than ever before, and developing new processes and technologies that allow them to use that data to offer better products and services to their end users. With the right leaders, talent and know-how in place, businesses can leverage their data to solve real-world problems in real time, ultimately, creating new opportunities for innovation and better ways to serve their end customers.
Ana: On the flip side, many consumers have lost trust in big data with cases like the Cambridge Analytica scandal, making them [00:01:00] fearful of and resistant to businesses using their data. Safe to say, there's been a lot of negative hype about data science in the past, and a lot of it with good reason, but it's because of this very duality that I wanted to make this episode.
Ana: After years creating content about tech and big data, I realized that I still didn't truly understand how businesses are actually using my data with respect to the kinds of problems they're looking to solve when they're actually looking at the data that I've created. Before I hit play on the interview, let me tell you a bit about my guest, Sandi Ganguli.
Ana: He began his career at the Office of Naval Research in design optimization, looking at how to make improvements across supply chains for large pieces of manufacturing like ships or airplanes. After discovering his passion for improving operations and processes, he spent his PhD years developing a model that used algorithms to design optimized manufacturing. [00:02:00]
Ana: He's been working in data science since 2004 where -- as you'll hear now -- he started working at Expedia. Since then, and this is something that I really think comes across a lot throughout the interview, Sandy has become truly passionate about data science, seeing his work as key to developing digital environments that help people work more productively in the hybrid and remote working.
Ana: Prepare yourself for a jam-packed episode with lots of really great insights about the future of data science. Sandi does a great job explaining the fundamentals of data science going into the reasons why data science and AI have become such massive hypes in the business world. And then he also shares some awesome insights on the future of the practice.
Ana: I want to give a massive thanks to Sandi who's been a fantastic guest throughout the production process, sharing his experiences and opinions openly, and also helping to demystify what I think is a very complex and really interesting world. That is the world of data science. I hope you enjoy the [00:03:00] episode.
Sandi: In Expedia, it was 2004. The world was just getting out of the first dotcom burst, so people were starting to get back the trust on, on internet and travel was pretty hot back then. Online travel. So we worked on some very cool projects at Expedia on what's the best way to select, what's the best way to select the flight you want to get, what's the best way to select the right hotel, so it was the first real-world application of data that's coming from the internet where we came up with these awesome algorithms to give you the best experience that not only helps you decide where to travel and how to travel, but also make sure that the Expedia as a company was doing better in the bottom.
Sandi: I joined Microsoft within about four years after that. And the thing that fascinated me at that time was cloud. Because that was early stages of when cloud was being formed. And I was like this basically is the holy grail of computing where you're not doing your [00:04:00] own data science in your own box, but actually using a giant data center to do whatever you like, and store infinite amounts of data. You can compute really fast, so that's exactly where I wanted to be.
Sandi: So, I joined this new wave of search engine competition between Google and Microsoft in that era, and then figured out how to use massive amounts of data and build algorithms for many things like fraud detection, predictive maintenance of data center hardware. And then once I did that, my natural transition from there was hey can I get a little bit towards more of the customer-oriented problems. And that's what made me move from Azure and Cloud services and all of those, into Office -- where I have been working for the last eight years. That said, whatever I'm saying today is essentially my personal experience. I do not represent Microsoft in any way for [00:05:00] this conversation, and I will just be talking about what I've learned in the past 16 years of my data science experience.
Ana: So you're representing yourself and I love that. Thank you so much for telling us all your background. That sounds awesome. It sounds like -- I love what you were saying about the marine engineering and the parallels between marine engineering and data science as kind of looking at a massive problem and trying to understand even at the most micro scale, how things need to fit together for the massive whatever it is that we're talking to work. So that is, that's really awesome. I never thought about it that way. Is that the purpose of, of data science to look at the micro and the macro?
Sandi: Absolutely Ana, I, I think you've kind of hit the point there. When you're looking at something from a meta picture, looking at it from the 30,000-foot level of what you're trying to solve at a macro level, you need to understand how to unravel that, decompose that into little [00:06:00] problems. And again, how do you aggregate all the solutions into one single problem? So that's exactly what data science organization does within any company and enterprise right now. Is figure out all the tiny problems to solve that accumulates to making the company better in terms of bottom line.
Ana: Right. And does it always have to be about the bottom line or are there other metrics that you could be using to look at the kind of success of the data science?
Sandi: I think that is layered. So there are several layers to the kinds of problems that data science tries to solve. It could be as granular as -- hey, find me the most important bugs in a set of code. Or it could be as high-level as -- tell me the next two set of investments you're trying to do in the next two quarters. So they're all data science problems. It just depends on how you want to solve them and what is the granularity you want to look at.
Sandi: In general, what I've seen is it boils down to how well you can [00:07:00] decompose the problem, into an actual mathematical formulation and how you advocate and story-tell the solution to your management.
Sandi: It's a great way to think of data science as solving or answering questions at various level using sound principles of mathematics and statistics, and then making sure that the decisions that are based upon it are also founded on solid science.
Ana: Ok. And then so to put that into a bit more context -- say for example in your role at the moment at Microsoft -- what kind of data science questions are you looking at, and what are the overarching objectives of your team in looking at those questions?
Sandi: We look at various kinds of problems. It starts as granular as there is an issue that the customer is facing. Can you identify that? Or a customer has reached out and say, here is my problem. Can you identify that in actual data streams?
Sandi: And [00:08:00] then that's one of the granular problems we're looking at. And then we also look at things like, ok, what are the things that we want to invest in going forward given the current trend of productivity, given the current trend of what people want to do. For example, people are now working hybrid, what does it for us?
Sandi: And then how do you make sure that we are positioned in the best way to understand the needs of customers and provide products that make customers more productive.
Ana: And so – since you mentioned the start of hybrid working -- over the past two years alone, we've created 90% of our data. What does that influx of data look like for a data science team and what kind of adaptations have you had to make in order to be able to still have the same outputs as you were beforehand?
Sandi: I'm going to start by giving you a little bit of historical perspective of how the data volume has exploded. Ok. [00:09:00] If you take anything before, you can literally neglect it in terms of volume -- not enough data at that time. So there was an estimate that I got that says, if in 2010, the total volume of data in the world that was being created amounts to 2 zetabytes, right now, the trend is by 2025, it's going to be 181 zetabytes.
Sandi: That's how much data we are currently producing and trying to consume. For data scientists, there has been a transformation, and I'm going to talk about this from the perspective of two very pivotal articles that have come out and published and are very popular. Back in 2012, Tom Davenport and DJ Patil published an article saying 'Data Scientist: The Sexiest Job in the 21st Century,' and that was literally the point where a lot of enterprises recognised this as a discipline and [00:10:00] understood that -- hey, we've been getting statisticians and quant analysts as a one-off, but we need to have this program.
Sandi: Fast forward to like 2022, they publish another article – 'Is Data Still Sexiest Job of the 21st Century?' Since they published the first article, I'm sure they'd say yes it is one of the most in-demand jobs right now. But there have been some changes, and this is related to the question you asked, Ana -- is what has the data explosion, the way we work changed, how has that changed the profession?
Sandi: I think the biggest change that has come is that data science discipline has actually been decomposed into several different roles. Now that enterprises and people realise that this is functional unit that needs to be part of a bigger organisation to do anything. So now they've kind of split it up into various specialized roles -- for example, a data analyst looks at high level business questions, a data engineer looks at how to best structure the data and democratise it across the [00:11:00] organization. The actual data scientist that looks at the data, manipulates it, does explorations with the data and answers some difficult questions that is not really available through aggregated metrics.
Sandi: There are some other distinct roles that have come out like AI scientists and research scientists. AI scientists are people who are actually working to figure out how scenarios within the enterprise can now be automated, and AI can be implemented at various levels within the product.
Sandi: Research science on other hand basically work on the algorithmic changes and development of new algorithms that data scientists and AI analysts and AI scientists can all use. So there's a spectrum of opportunities from what we used to call 'data scientist' now that have really come up.
Sandi: Explosion of data working hybrid – working hybrid essentially means that we now collect more [00:12:00] data than we used to. And then the importance of network collaboration and virality is way more than what it was five years ago.
Sandi: So digital customers, users of the product, networking and vitality is now front and center. It's not a 'nice to have' anymore.
Ana: Hold on, I'm going to jump in, because you've used a couple of words which I think -- so I'm trying to think about people listening to this podcast who might not be fully in the tech world. When you talk about virality and connectivity, what exactly are you talking about?
Sandi: Networking and virality essentially boils down to how people work together in a digital environment without actually physically being together. If you take productivity efficiency of work, that is one of the objectives of enterprises you'll see that has now gone through a paradigm shift and people are now [00:13:00] trying to be as productive and as efficient as possible through remote locations. What virality and networking would generally mean is that – how are we measuring the effect of working in groups in a digital environment? We never used to measure that. People went to work, brainstormed, get stuff done. Right now, it's different. Right now, it's like people from different geolocations come together in a, in a software environment and get stuff done. How do we measure fact productivity has improved or hasn't changed anything. That is some of the ways that enterprises are trying to move forward. Is bringing together various groups of people and trying to quantify what is the effect of networking in this case. For example, you and I worked on a document and we both shared content. If you and I worked in silos and we went back and forth between us on figuring what is best content to add, would it take less time or more time, or would the content be as high-quality as it is? [00:14:00]
Sandi: Abstract it out to any industry. In any industry, what we're trying to do is understand the effect of collectively working on certain objectives and measuring that. That is basically what has really come out as a fascinating change after the pandemic. So that's where essentially we see why hybrid is so important.
Ana: And then -- since you mentioned AI, and how it's being used to automate certain functions. What's the interaction between AI and data science, and will automation mean that the role of data scientist becomes no longer?
Sandi: Excellent question and this is exactly why we're going to break out of the hype and talk about what it is in reality.
Sandi: So AI is definitely something that we are awaiting its proliferation and democratization going forward. But data is a foundation for all of that.
Sandi: [00:15:00] Let's define AI. The way I see AI is the application of algorithms using a computer system on large data sets to simulate human intelligence. So, you're looking at a picture saying, 'Hey, this one looks like a flower.' Because you have, your memory, has a whole bunch of flowers that you've looked at before. That is a data set in your head. So we simulate that in various ways.
Sandi: And as soon as you talk about data sets, and robust data and big data, you cannot do anything unless you are correct. You have to apply solid scientific principles on that data to actually understand what sense you want to make out of it.
Sandi: That is why I still think data science is the foundation of all AI. AI to me is the culmination of various data science methodologies and [00:16:00] applying a lot of computer science and machine learning on top of that to get outcomes that can be used to simulate human intelligence. To me, data science is still the building blocks of AI.
Ana: Do you think that we'll ever reach a point where, I can't remember the word, but there's a word for when artificial intelligence is so intelligent that it's capable of the same level of intelligence as a human being.
Sandi: I don't know that I know a word for that, but I think it's what my daughter says -- when will the robots take over our world?
Ana: Yeah, are they taking over?
Sandi: I'll think we're a long way off. One example that I'm seeing is self-driving cars. It is an example where I think we are still, I think, decades off from where we can truly hand over our lives to an algorithm and let them take care of it in morning traffic. So we're inching there.
Sandi: I think what I would paint a realistic picture that we will get a lot more proliferations of the mundane jobs being taken over [00:17:00] by AI making us more productive on a daily basis. For example, today we're overloaded with information: You get up in the morning, you flip your phone, and then you see, ok, I've got like 900 messages from different chat groups doing this. The real next step would be extracting a summary of all of those and giving you a rundown of what happened last night when you were sleeping. Here is a summary. Ok. So that's one example, which I think is much more realistic than robots taking over the world.
Sandi: It's easy to understand science fiction as something of a fantasy but if you take AI, AI is something that is actually programmed by humans right now. So it'll only get as much as you program it. At some point in time, it will start to develop where algorithms are so self-learning that you don't need to program anything new, but I don't see that within the next two decades.
Ana: So, 20 years everyone. You've heard that here first. Twenty years until we get [00:18:00] run over by the robots. And then, so one of the big things that a lot of businesses are struggling with is a digital talent gap, which obviously makes it all the more challenging to build a data-driven team or digital team. Is that the same for a company like Microsoft that has so much clout and brand awareness? What does the talent gap challenge look like? Is it the same or different?
Sandi: I'm going to generically speak about the whole discipline as a whole and what I'm, when I'm looking at several industries, and Microsoft is just one of them, so it's generically applied to Microsoft as well. You can break up 'talent gap' into two pieces.
Sandi: One is talent gap of the discipline itself. So are we generating data scientists at the pace the world needs? I think that it's come a long way there, so like 10 years ago we used to have a couple of programs and mostly applied statistics on operations research and [00:19:00] all of those that a few graduates and even lower, in the masters and the PhD program, and a lot of them went into academia.
Sandi: Right now we have a lot of universities doing data science programs as a master degree or a certification course, which you can basically take while you're working. So the pipeline for talent has significantly changed over the last 10 years.
Sandi: You're seeing a lot more resumes than we used to see 10 years ago. There are still some gaps within the data science discipline itself. These are the foundations of data science and statistics. Computer scientists are those being those being supported? And are people coming out of these programs actually getting the training that they need?
Sandi: And I would say partially, yes, they're getting a lot of theoretical training, but still when they come to the industry, they're faced with a real-world problem, and it's totally different in terms of the type of data they [00:20:00] see, the type of problem they're solving, the ambiguity of dealing with real life is so different. So there is a gap between real-world problem-solving and the training they are receiving currently in the universities. That gap has reduced, but it's still [there].
Sandi: The other talent gap that I'm seeing is people who are in the periphery working with the data scientists, their knowledge of data science as a discipline. Their knowledge of data systems is lower than where you would expect it to be. And this is for all industries. Let's say data scientists working with marketing folks, sales folk, engineering folks, product managers. There is the real potential of using data and data scientists as basically with these peripheral disciplines, actually know exactly what they want to do with the data. That is something that is, I would say is a little bit more reactive. Like, hey, the business needs X based on this, let's go find and this and into some data to actual innovation.[00:21:00]
Sandi: So we're thinking of data science innovation as a very research-oriented project, but I think the innovation can be magnified a lot more when these peripheral disciplines have the required training of what to do more with data. So that's where the talent gap currently lies.
Sandi: And most organizations are now driving data-driven cultures where the other disciplines are understanding the value of the data. Also figuring out how to best utilize not only the data science talent, but also to make sure that how they can contribute to some of the data-driven workload that the data science team is currently doing.
Ana: When it comes to the duality of the talent you mentioned, what would you say to someone who's looking to establish a data science team within their own organization. What are some key attributes?
Sandi: So, this is a tough one to answer because I would start it by saying, it depends. If you're looking for what is the right data science team [00:22:00] organization structure, in any enterprise, there are various ways to approach the problem or approach your need, essentially. So let's start with the two extreme cases.
Sandi: One is you have a completely centralized data science team, and people go to that particular team to ask questions and get their problem solved. The other extreme is a completely decentralized data science team, where there are a couple of data scientist that are embedded in a whole bunch of teams. They both have pros and cons and the real answer is somewhere in between. Some of the pros of having a centralized data science team is that it's good for career growth or mobility of talent. It is good for applicability and reuse of several data science techniques.
Sandi: Whereas some of the pros of a decentralized data science team is that there is much more domain knowledge within the data scientists in each of these teams. The efficiency of working within each of these teams is much higher, but there's also lack of career growth, and people [00:23:00] get bored doing the same things too often. Where you are within these two extremes depends on a couple of things. One is how mature is your organization in terms of data-driven thinking. Going back to the talent gap of the disciplines that interact with data science, how mature are they thinking in terms of using data? If they are really mature, you're heading more toward a decentralized model. If they are starting afresh and the size of the organization is smaller, then you're heading more toward a centralized data science team. Where you place depends on the type of questions you're asking, the data maturity of the disciplines that are interacting with data science and also, your organization's size.
Sandi: You get to the right point by trial and error, and then you keep shifting depending on where your organization goes.
Ana: Sure. So it's a very iterative approach to building out a team.
Sandi: Absolutely. Yeah.
Ana: And would you [00:24:00] say that's something that echoes also in the culture of data science as a practice in itself?
Ana: For example, you have other digital ways of working that are very collaborative and agile, and as we become more digital, it's obviously more necessary for more teams. Is that something that has always been there in data science or is it a newer way of doing things in terms of the collaboration?
Sandi: Yes. The two aspects of what you said, and this brings a whole lot of ideas to talk about right now, is the iterative nature of solving anything pre data science discipline, in let's say the early 2000s, the whole mentality of solving a customer solution was let's do it, be done, one, and be done.
Sandi: As we've seen a shift there and organizations, especially mature large organizations, are now taking an approach where they have to iteratively solve the problem because it's just too complex right now. There are so many unknowns. Even with [00:25:00] so much data, we really don't know that much about a complex creature like human beings.
Sandi: We really don't know a lot about what are the macroeconomic trends that affect us. So we make incremental changes and then we learn. One of the culture changes that a data scientist does as a job is to basically work with these folks, collaborate with them and express the importance of an iterative culture. Iterate more, fail fast and fix. That's basically what the whole profession is trying to drive through and bring it down to an algorithmic level.
Sandi: Iterate until the time the errors come to zero. So that's basically what we're replicating in an organization. Keep inventing, keep doing the innovation you want until the time you succeed. You will fail a whole bunch of times before that.
Ana: I love that because it seems so often in the business world that the concept of failure is almost taboo, in that it's either we get it right the first time or we failed. Whereas I like that vision of success as being [00:26:00] a collection of failures that lead you to a success rather than it being just a success in and of itself.
Sandi: Very nicely put. That's, that's perfect.
Ana: So, moving forward a little bit, I wanted to talk about diversity and inclusion. Obviously stem careers generally have this problem -- lack of diversity. What challenges does that create for data science, that there's a lack of diversity?
Sandi: Wow Ana, this is a very relevant question and I want to start by saying that there is an implicit assumption that the data science discipline requires highly qualified people. I've seen that to be a successful data scientist, you don't need a Masters and three PhDs. You don't need -- I mean those are required in some cases, but that's not a requirement.
Sandi: But there are some fundamental education basics that you have to complete when you're making decisions that decide the fate of the company in the next few [00:27:00] quarters, or what is really wrong with the product that the customers are expressing their desat on. You need to be able to actually dissect the problem and have a very credible and realiable way of solving or storytelling it. That requires a basic level of education.
Sandi: What has really turned out in terms of diversity and inclusion is a bunch of college degrees, which we basically take as milestones for this education has been a blocker for a big section of the society. So the underprivileged and the folks who don't get a chance to go to college and do graduate programs or PHDs do not end up getting employed in this discipline as a result of this.
Sandi: So how do we tackle this? So what we've done, and you don't have to be a giant corporation to do this -- any data science leader should be able to do this themselves. [00:28:00] You can employ people who are data savvy, who have passion in this area and who want to learn more and educate themselves while they're on the job. Like I mentioned before, the data science discipline has a wide spectrum of work.
Sandi: It can be cleaning data, manipulating data, moving data, extracting data building algorithms and whatnot. If you have people who have not crossed those milestones of college degrees, but they show the passion, bring them in, let them do parts of the job, and then give opportunity to train themselves through college degrees, through master program, through certification programs, and see them grow within the discipline. This is something that I actively make sure happens within my team is that we bring in folks who have not had the opportunity to get advanced degrees, but want to do so on the job. And from what I've seen in my organization is that [00:29:00] they are brilliant managers as well. So it just takes them, some opportunity and once you put them there, they just work wonders.
Sandi: So that's that. The other part that I wanted to really talk about is diversity and inclusion in terms of the output. That is many times skipped so we have the data science team, which in terms of gender and racial distribution, is something that we're working towards and those are the solutions that I kind of touched on.
Sandi: The other part of this was, when you're designing the solution for, let's say, an AI algorithm or a personalization or recommendation algorithm, what we should keep in mind is that we always look at the end product or the end metric as what gives the biggest ROI. What happens in the process is that we fail to include a large section of the society that may not fall into what you define as ROI.
Sandi: [00:30:00] For example, accessibilities. So you have this excellent speech recognition algorithm, or you're doing something that generally benefits a whole number of people and you look at your metrics and say 'Look, we're doing awesome.' But you're actually excluding a whole lot of people who may not fall in the same category. This is something we definitely keep in mind when you're providing a solution. Is that responsible enough? Is that including people who have accessibility issues? So these are the things that I have worked on around D&I as a whole in these areas.
Sandi: The opportunity that we can present as a discipline is diversity in all kinds of economic strata. So I think there are folks that, uh, don't have the opportunity to get access to large-scale computing. They don't have the opportunity to basically take courses that are relevant to data science.
Sandi: We're trying to overcome some of those [00:31:00] by bringing in people who are not trained in the profession. And the ability or the passion to kind of train themselves during the job. So this generally covers all kinds of individuals that are there, who have extreme potential but just get the milestones that we talked about.
Sandi: So, so, uh, it's the inclusion of different communities and people in different economic strata is basically our first line of defense, in the sense of making this a very very non-diverse discipline.
Ana: How would you identify a passion for data in people? Because I guess not everyone has the marine engineering story that you told us earlier, and that kind of fit so perfectly into data science, the way that you analogized it. How do you see that in other people that come to the role?
Sandi: We have a bunch of ways to kind of find our talent. So we have conferences that we go to, there are hidden nuggets. There are others where [00:32:00] people show interest, and where their qualification is not a bar or a standard for them to go. So there are well-established conferences. There are hackathons we have in developing countries. There are a whole bunch of opportunities we give to schools and others. Our goal is to catch them young when they're underprivileged. So we have a lot of programs where we can present a data-related problem and see how students are doing. Bring them in for high school internships, or the same for college.
Sandi: There are college dropouts that basically are very good at the job. But then they cannot continue because they don't have the financial background to do that. So it takes effort. It takes time to actually go and solve this. For the data science leaders listening to this: it is worth it to kind of cordon off some time and do this outreach to schools and colleges [00:33:00] and find the people that have the real passion in it.
Ana: On the subject of outreach, there's a lot of public criticism or mistrust of big data. Does that have to factor into your outreach and communications? Like with customers, for example, how do you tackle the mistrust?
Sandi: Excellent question Ana again. I think customers in general are in a quandry right now. They face the dilemma of how much should I trust a product and how much harm is it doing to me? As you've seen the past 10 years or so, customers life has improved in general by how enterprises have used their data. For example, a search engine is still dependent on what you search, a recommendation engine is dependent on what you're looking for, and how personalized is what the product is servicing to you.
Sandi: However, users are also looking at news [00:34:00] and then figuring out all these evil effects of sharing data. There is data breach, there is, you know, foul ways of utilizing customer data. And then I think there is a lack of understanding of what are the positive effects of using the data as opposed to what is really happening in the not so positive way.
Sandi: The events of the past years have not really helped over here. So people have a tendency to mistrust any time they are asked to share data. And that's with good reason because you're seeing a lot of evil effects of sharing the data where the customers are generally impacted by this. But at the same time, we need to have a way to make it visible to the customers how their lives are getting improved by data.
Sandi: The strategy we're trying to take over here is that we need to build trust. Everything we do relies on trust. To build trust, the first thing we want to do [00:35:00] is give customers control over their own data: where they want to share and where they don't want to share. We basically make boundaries of what we never use in any of our products. So, let's say content, something that we never use. That's a great way to build trust.
Sandi: We also want to make sure that we make visible to the customer what we do with the data we collect. Here's why you're getting a bunch of recommended solutions -- because you've shown interest in X. Here's why you are getting this offer.
Sandi: So these are things that we have to get back to build customer trust. These are also ways where we can say that we're reducing the overhead of you searching more to get what you need, making your life easier. So, so the balance right now is on us, the products kind of make sure that we rebuild the trust by showing the benefits of using your data and making sure you have complete transparency of how we're using the [00:36:00] data. So extend that to what's happening in the regulatory world, like in EU, in parts of California, these are actually ways in which the customers have expressed the fact that we do not trust a lot of this data. That's fair.
Sandi: What I would say is that we can still make products better by making sure that we're compliant with all the regulations that are currently in place and data science needs to come up with different ways of making sure that users' privacy, users' security is maintained while we still provide the value of collecting your data or one area I'm totally fascinated by and it's good to talk about it in this context is, 'How do we simulate real data when we don't want to get the actual data from the users?'
Sandi: There's an entire area which I think is ripe for innovation, where we basically say, there's a sample set [00:37:00] of data coming from other folks that are OK with sharing the data, and then we kind of project -- what would the general customer base look like had they released all this data? So there's a wide margin of error, but still you have an idea of what to do and data science should start innovating in an area where they're actually not looking at your particular set of data, but can get it from a much more aggregated, more simulated way. Those are ways in which you keep the trust on and make sure we continue innovating.
Ana: It sounds like data science and security are almost converging in that your priorities at least, are aligning with each other a lot more. Is that a trend that's going to continue?
Sandi: Yes, it's definitely a trend that is going to continue. There are various areas that are currently ripe for innovation over here.
Sandi: Security does not always mean that we shut down every data stream coming from users or we make sure that you have nine different levels of authentication coming from you. That just makes a [00:38:00] user's life very difficult. We want to make you secure also, make it easy for you to access your information, to give you a better experience of using the product. And part of why we really want to pursue this area is to make sure that we have enough checks and balances that we're providing you as a user -- give yourself control on what you want to do with your data. That's number one.
Sandi: Number two is we want to use technology to ensure that your data is secure. If you were to provide data and we have a foolproof way of completely wrapping it in an environment that cannot be leaked, that is trust for the customer. We can do stuff within that environment, and there are various ways to describe a trustworthy environment.
Sandi: We're also looking at things like [running] eyes-off algorithms to data that you don't want us to see. So there, there are ways to do that if you have content data you want to get ads on, or you have content data and you want to basically get some suggestions on, we [00:39:00] can do that -- and we want you to look at it.
Sandi: So, security and data science are coming together to give users a better experience of the product while maintaining trust.
Ana: Right. So it's kind of a more personalized service, but at the same time, one that has a sense of privacy in that you know that the stuff you're doing is not going to be being looked at. Is that right?
Sandi: Exactly.
Ana: Thank you. We've reached the end of the questions that I wanted to run through with you today. Just one final question, which I'm going to be asking everyone in the series, is what does the future hold for data science? What's the ideal scenario? What do you think that people can expect to see from data science in the next 10 to 20?
Sandi: Oh, that's a big one, and it'll definitely be biased by what I want to do going forward. The future of data science just exploded again. This time, not just for the volume of data, but also for commoditized AI models. Whether it's large language models or robotics, AI will drive a bunch of customer needs. [00:40:00] Like I mentioned before, the ability to customize AI for the user is actually a data science job. Gathering the right data, working with the right researchers, data scientists, will actually make AI useful for humanity and at scale.
Sandi: Second, I think the internet of things -- currently an unsung hero, like neural nets back in the 80s -- will emerge out stronger. The need to connect devices that talk to each other will only grow, and their applicability for smart homes and enterprises is going to get stronger. Last, and probably the most important part where I really want to keep my focus on, is as climate change will continuously threaten our way of life, we will invest in smart energy solutions that will include using AI to reduce consumption. Currently, humans are consuming everything at a rate that is [00:41:00] unsustainable by the planet. Where we don't have alternative solutions right now, the only way to reduce or actually minimize wastage over here is by using data and algorithms that inform such wastage.
Ana: Right. That's so interesting. I'd never thought about how important data science will be for the environment and for trying to come up with solutions to these huge, big-world problems that we are facing at the moment. Thank you so much for your time today, Sandi. It's been an absolute pleasure having you on and hearing all about data science. Thank you very much.
Sandi: Alright, thank you Ana and, thanks for having me. It's been a pleasure too.
Ana: That's all for today's episode, folks, at a time when AI-based technologies like Chat GPT are proliferating and access to data-driven insights is becoming more easily accessible for everyone, maintaining quality and talent within data science will be essential to [00:42:00] ensuring that the insights that we're getting are both reliable and based in solid science.
Ana: As we move toward an increasingly connected and digital world, businesses that value data science will undoubtedly be best positioned to adapt with and thrive in the face of continued technological innovation.
Ana: I hope you found Sandi's insights as enlightening as I did. Thank you all for listening, and if you did enjoy today's episode and want to hear me interview more business leaders about the latest hypes in the business and tech world. Please make sure to like and subscribe wherever you get your podcasts. Tech Beyond the Hype is a TechTarget original podcast.