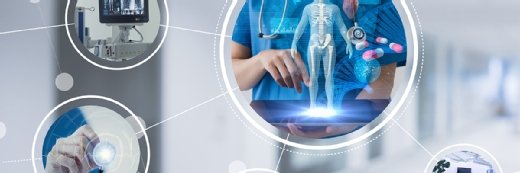
Getty Images/iStockphoto
Healthcare strategies: Machine learning for treatment planning
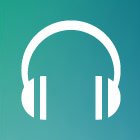
Machine learning can use digital twins and real-time feedback to improve patient care.
This week on the Healthcare Strategies podcast, Shania Kennedy, assistant editor at TechTarget's Xtelligent Healthcare Media, speaks with Amanda Randles, assistant professor of biomedical sciences at Duke University, about the uses of machine learning and artificial intelligence in medical research and clinical care.
Listen to the full podcast to hear more, and be sure to subscribe to Healthcare Strategies on iTunes, Spotify or Google Podcasts.
Machine learning can help researchers learn about disease through biomedical simulation and high-performance computing.
"A lot of the projects that we're focused on really look at blood flow modeling. We're trying to understand how different treatment options may affect the hemodynamics at a specific patient. So the crux is creating almost a digital twin. Those blood flow simulations are incredibly computationally intense, they take a lot of compute resources. They can take anywhere from a few minutes to 12 hours on large scale resources," Randles explained.
"We're starting to use machine learning to augment that need for the large-scale computation. We were identifying the minimal number of distinct simulations you had to run for each patient to then be able to train the machine learning model and predict the rest of the states without having to individually run them. Instead of just trying one treatment option, can we predict the results of 10, 15 different treatment options, or even five treatment options, before the [doctor] ever go[es] into the operating room?"
Being able to generate a breadth of treatment options improves patient experience as well, by providing noninvasive alternatives for gathering patient data.
"What we're seeing now is more of the acceptance of providing a noninvasive way of getting at that measure by creating this 3D model of the patient's anatomy, whether that's from CT scans, MRI scans and then running a blood flow simulation," Randles said. "In this digital vascular twin, you then can measure the pressure gradient in a noninvasive manner and determine if that patient needs a stent or not."
Machine learning tools also have a positive impact on health equity, especially lighter-weight models.
"If you're trying to run a simulation of around several million hours for compute power, it needs to be a hospital that has this large compute access," Randles said. "If you have a real-time, much lighter weight machine learning-based model, you could deploy that on a tablet, you could deploy that in the cloud. You're allowing easier access for people to access this type of technology. Aside from being able to use techniques like transfer learning and other options to actually apply to different types of population, really tune it to those patients and those subsets of the population."