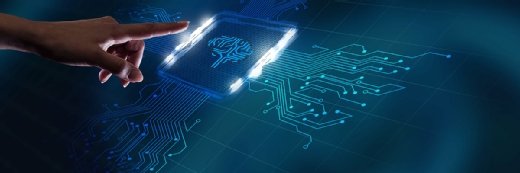
putilov_denis - stock.adobe.com
GenAI search vs. traditional search engines: How they differ
Search engines are the original way to browse the internet. GenAI search is a new method to explore and get answers to queries. Learn how it compares to traditional searches.
In the early days of the internet, it became clear that some form of technology was needed to help users navigate and find information as traffic began to surge. This led to the emergence of the first search engines, which helped users find content on the growing internet.
For most of the history of the internet, search has been predicated on the same basic process: Content is indexed to match keyword queries. With the advent of generative AI, the search landscape began to shift in a meaningful way for the first time in decades. GenAI search enables users to get results that include more and are not limited to keyword relevance. With GenAI search, large language models (LLMs) provide answers instead of only a page of results.
Multiple providers have entered the GenAI search market in recent years. Startups such as Perplexity AI have come into the space with entirely new GenAI search services. OpenAI, the vendor behind ChatGPT, entered the space with its ChatGPT search. Existing search vendors, including Google and Microsoft, have also offered AI options. Google has its AI Overviews technology, and Microsoft Bing has also integrated GenAI augmented search. Meta is also reportedly exploring GenAI search for its social media platforms.
What are GenAI search engines?
GenAI search engines are an evolution of search engine technology.
The primary defining innovation and characteristic of GenAI search engines is the use of LLMs to deliver a different type of experience than a traditional search engine. A GenAI search engine uses the LLM to understand, retrieve and generate responses to user queries. A regular LLM generates answers to user queries based on the knowledge available up to its training cutoff date, limiting its ability to provide information beyond that point.
In contrast, a GenAI search engine has no such knowledge cutoff and can use up-to-date information, similar to a regular search engine, to generate responses. GenAI search engines combine machine learning and natural language processing to understand user queries semantically.
The use of an LLM provides a series of different benefits and capabilities, including the following:
- Interprets user intent from natural language queries with more accuracy.
- Provides direct answers to queries, instead of only links to resources.
- Offers detailed explanations and clarifications.
- Combines and integrates information from multiple sources to deliver comprehensive responses.
- Engages in follow-up conversations with options for related topics and queries.
How do GenAI searches work?
GenAI searches work similarly to how an LLM normally works but with some notable distinctions -- most significantly, the incorporation of updated information.
The basic procedures involved in GenAI searches include the following:
- Query input. The user enters a query in natural language. The system processes the query by performing tasks such as tokenization and key phrase identification.
- Intent and understanding. The GenAI search technology not only looks at the user query in terms of the words used, but it also attempts to understand the user's intent for the query. The intent is to understand what the user wants to do with the query. For example, is the intent of the query informational, navigational or transactional?
- Information retrieval. The GenAI search system uses its knowledge base data set to answer the user's query. That knowledge base includes the pretrained LLM and updated real-time web crawling.
- Response generation. A response is generated that best matches the user query and intent. The generated response is refined for accuracy, relevance and coherence. The AI search engine structures its response in a coherent and readable format. It may include relevant citations or links to source material. Some systems also include expandable sections or follow-up question suggestions.
- Feedback and continuous learning. Many GenAI search engines incorporate feedback mechanisms to improve performance over time. That feedback can be implicit or explicit from the user on the quality of the search results.
How do traditional search engines work?
Traditional search engines, such as Google's core search index, operate on a different principle compared to GenAI search engines. Traditional search engines help users find the best answers to their queries by offering links to relevant sources.
The basic process behind how traditional search engines work includes the following:
- Crawling. The first stage involves automated web crawlers, or bots, that scan the internet for web content. These crawlers systematically browse the web to discover new and updated pages.
- Parsing and analysis. Once a crawler discovers web content, it analyzes the structural elements of the page, such as title tags, meta descriptions, headings and body text. This analysis helps the crawler identify and extract links to other pages.
- Indexing. The discovered pages are analyzed and categorized based on their content, keywords and other parsed data.
- Ranking. The search engine uses complex algorithms to rank the indexed pages based on the relevance to the query and the quality. One example of a ranking algorithm is Google's PageRank, which has undergone numerous updates and changes over the last two decades.
- Search engine results page (SERP). The relevant results of the user query are ranked and presented on a SERP. Each result typically includes a title, URL, and brief description or snippet.
Key differences between GenAI searches vs. traditional search engines
There are numerous key differences between GenAI search and traditional search engine approaches.
Aspect | GenAI search engines | Traditional search engines |
Response | Direct, conversational responses | List of links with snippets |
Content generation | Can create original content on the fly | Only retrieve existing information |
Query understanding | Advanced understanding of natural language and user intent | Primarily keyword-based with some semantic understanding |
Contextual awareness | Maintain context throughout a conversation | Has limited context awareness -- each query treated separately |
Information synthesis | Can combine information from multiple sources | Present separate results from different sources |
Update frequency | Can incorporate recent information | Depend on web crawling and indexing cycles |
Personalization | Can tailor responses based on conversation history | Personalize based on user data and search history |
AI's impact on SEO
For nearly as long as search engines have existed, individuals and organizations have focused on search engine optimization. SEO attempts to improve a webpage and its content to achieve a higher ranking in search results.
The rise of AI-powered search engines is impacting SEO strategies in significant ways, including the following:
- Keywords aren't enough. SEO strategies that are focused solely on keyword optimization may become less effective as search engines provide more conversational and context-aware results. With AI's ability to understand context and intent, the focus shifts even more toward creating high-quality, informative content that directly addresses user needs.
- Natural language needs to be optimized. SEO strategies now need to consider natural language patterns and conversational queries.
- Driving traffic is more challenging. With traditional search engines, SEO was often seen as a straight line to getting better ranking, leading to clicks and traffic. With GenAI search, that's no longer guaranteed. With summarized answers, simple queries may not lead to clicks or publisher traffic. What's needed is more detailed information than a simple summary can provide. SEO and traffic optimization need to do more to be successful.
The future of search and AI
Traditional search engines have been on the internet for over two decades. Over that time frame, the traditional search engines have iterated many times to keep delivering the best results for users. The path for search and AI will likely be the same with continued evolution and iteration.
As these technologies continue to evolve, the market should expect to see the following:
- More personalized results. AI will likely provide increasingly highly customized search experiences based on user preferences and behaviors. That goes beyond the current versions that understand basic context and user intent.
- Enhanced multimodal search. Much like LLMs are on a multimodal path, future AI search engines will likely better integrate text, image, voice and video search capabilities.
- Improved accuracy. As AI models are refined and training data expands, the accuracy and reliability of AI-generated responses will likely improve.
- Publisher engagement. With traditional AI search engines, publishers typically welcome web crawlers as they help to drive traffic. With GenAI search, where clicks and web traffic are not guaranteed, it becomes increasingly important for publishers and GenAI search technologies to strike content agreements. Perplexity AI is starting to engage with some publishers, though others -- such as The New York Times, which filed a lawsuit against the GenAI search vendor -- are not welcoming collaboration.
Sean Michael Kerner is an IT consultant, technology enthusiast and tinkerer. He has pulled Token Ring, configured NetWare and been known to compile his own Linux kernel. He consults with industry and media organizations on technology issues.