What are data silos and what problems do they cause?
A data silo is a repository of data that's controlled by one department or business unit and isolated from the rest of an organization, much like grass and grain in a farm silo are closed off from outside elements. Siloed data typically is stored in a standalone system and often is incompatible with other data sets. That makes it hard for users in other parts of the organization to access and use company data. And when an organization aspires to be data-driven, siloed data can be a huge obstacle.
Data silos can have technical, organizational or cultural roots. They tend to arise naturally in large companies because separate business units often operate independently and have their own goals, priorities and IT budgets. But any organization can end up with data silos if it doesn't have a well-planned data management strategy.
Why are data silos a problem?
Data silos hinder business operations and the data analytics initiatives that support them. Silos limit executives' ability to use data to manage business processes and make informed business decisions. They also prevent call center agents, sales reps and other operational workers from accessing relevant data about customers, products and supply chains. This is a problem for organizations implementing customer relationship management. CRM is increasingly essential to enhancing customer experience.
The specific ways that data silos can harm an organization include the following:
- Incomplete data sets. Data silos lock data away in separate data sources from users who can't access it. As a result, business strategies and decisions aren't based on all the available data, which can lead to flawed decision-making. Silos can also derail efforts to build data warehouses and data lakes that integrate different data sets for business intelligence (BI) and analytics applications.
- Inconsistent data. Many data silos aren't consistent with other data sources. For example, a marketing team might format customer data differently than other departments. Data errors by a sales team might not be identified and fixed. Data updates in other systems don't get made in a siloed customer service system. Such inconsistencies create data quality, accuracy and integrity issues that affect end users in both operational and analytics applications. They are especially problematic when external users, customers and partner companies are accessing one siloed data source via an application programming interface or online app, and data in other internal sources differs.
- Duplicate data platforms and processes. Data silos add to IT costs by increasing the number of servers and data storage devices an organization must buy. In many cases, those systems are also deployed and managed separately by departments instead of an organization's data management team. That further increases spending and inefficient use of IT resources.
- Less collaboration between end users. Isolated data sources in silos reduce the opportunities for data sharing and collaboration between users in different departments. It's harder to work together effectively when different teams don't have visibility into siloed data.
- A silo mentality in departments. Data silos contribute to organizational silos: departments and business units that guard their data closely and are reluctant to share it with others. They might also resist data governance programs that aim to break down data silos and ensure that company data is consistent and correct across all an organization's systems.
- Data security and regulatory compliance issues. Some data silos are stored by individual users in Excel spreadsheets or online business tools like Google Drive, often on mobile devices. That increases data security and privacy risks for organizations if they don't have suitable controls. Silos also complicate efforts to comply with data privacy and protection laws.
How data silos occur
A department or end user might go rogue and create a data silo even in an organization that has solid data management processes. More often, though, data silos are a consequence of how organizations are structured and managed as a whole, including their IT operations. The following factors commonly cause silos to occur:
- IT strategy and technology deployments. Some organizations have decentralized IT buying decisions and allow departments and business units to purchase technologies on their own. This often leads to the deployment of databases and business applications that aren't compatible with or connected to other systems. The same thing can happen when corporate IT teams are involved in purchasing decisions if a department needs a particular technology. The variety of data platforms now available also helps drive data silos. In addition to mainstream relational databases, organizations can deploy big data platforms, NoSQL databases, cloud object storage services and special-purpose databases to meet different business needs.
- Organizational structure and management. Data silos regularly occur when business units are fully decentralized and managed as separate entities. That's most common in large organizations with different subsidiaries and operating companies, but it can happen in smaller organizations with a similar structure and management approach.
- Corporate culture and principles. Even when IT and business operations are managed in a more unified way, company culture can spur the creation of data silos. There are fewer incentives to avoid them if data sharing isn't a cultural norm and an organization doesn't have common goals and principles for managing data. Departments might also view their data as an asset they own and control, further encouraging data silo development.
- Business growth and acquisitions. Growing organizations are prone to data silos. As a company expands, new business needs must be addressed quickly and additional business units can be created. Both of those situations are natural data silo incubators. Mergers and acquisitions also bring silos into an organization, some known and some that are hidden.
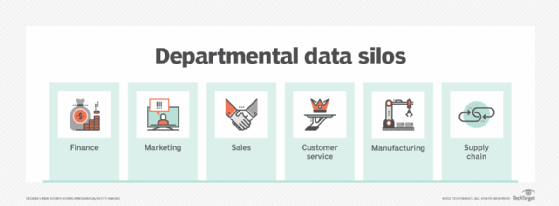
How do you identify data silos?
Because of their disconnected nature, data silos can be hard to detect. Ideally, IT and data management teams will create an inventory of the systems in their organizations and regularly update it to add new ones. Doing so should help identify and document data silos. But finding them all can be a challenge, especially in large organizations with business units that operate autonomously.
Evidence of data silos might come to light, though. Signs that point to them include the following:
- Different departments reporting inconsistent data.
- BI and data science teams not being able to find or access relevant data.
- Executives complaining about a lack of data from some business operations.
- End users discovering that data sets are incomplete or out of date.
- Unexpected, out-of-budget IT costs suddenly materializing.
How do you break down data silos?
Breaking down data silos lets an organization manage and use data more effectively. It often also helps lower technology and data management costs. The following approaches can be used separately or in tandem to remove silos and connect data assets to better support business operations:
- Data integration. Integrating data with other systems is the most straightforward method for breaking down silos. The most popular form of data integration is extract, transform and load (ETL), which extracts data from source systems, consolidates it and loads it into a target system or application. Other data integration techniques that can be used against silos include real-time integration, data virtualization and extract, load and transform, a variation of ETL.
- Data warehouses and data lakes. The most common target system in data integration jobs is a data warehouse, which stores structured transaction data for BI, analytics and reporting applications. Increasingly, organizations also build data lakes to hold sets of big data, which can include large volumes of structured, unstructured and semi-structured data used in data science applications. Those two types of platforms provide centralized repositories for data from different systems, making them a natural way to address silos.
- Enterprise data management and governance. Ultimately, it's best to not only eliminate existing data silos but also prevent new ones from being created. A more comprehensive data management strategy helps achieve both of those goals. For example, data architecture design documents data assets, maps data flows and creates a blueprint for data platform deployments. An enterprise data strategy better aligns the data management process with business operations. And a strong data governance program can directly reduce the number of data silos in an organization and promote common data standards and policies.
- Culture change. To really put a stop to data silos, it might be necessary to change an organization's culture. Efforts to do so can be part of the data strategy development process or a data governance initiative. In some cases, a change management program might be needed to implement the cultural changes and ensure that departments and business units adopt them.
What are the business costs of data silos?
According to IDC Market Research, incorrect or siloed data can cost a company up to 30% of its annual revenue. An organization can measure these inefficiencies by how many silos it has, how successful efforts to eliminate them are, and whether they continue to proliferate. In general, increased IT and data management expenses are the most tangible cost. But data silos also have the following intangible costs:
- Reduced productivity.
- Less effective business management.
- Missed business opportunities.
- Lower-quality customer service.
- A lack of trust in data that limits its use and business benefits.
The terms data silo and information silo are sometimes used as synonyms. More often, though, information silos are considered a cultural problem caused by departments or individual workers who don't want to share information. In addition to cultural change, one way to address the latter problem is to create an information architecture along with a data architecture.
On-premises and cloud-based ETL tools
Increasingly, organizations are moving their digital assets into cloud-based data storage. However, moving data around in any domain -- on premises or in the cloud -- requires tools for ETL to do the actual moving and to modify the data as needed in transit.
On-premises ETL tools can be automated, simplifying the process of consolidating and cleaning up disparate siloed data sources for centralized access. These tools are generally platform-specific and must be purchased, although most organizations with significant database or data warehouse assets already possess them.
Cloud-based platforms generally include built-in ETL tools to facilitate migration, and these can be used in much the same way -- to integrate data as it's migrated out of siloed data sources, transforming it as needed along the way.
Managing unstructured data can be expensive and time-consuming. Learn what strategies and tools organizations can use to manage this data cost-effectively.