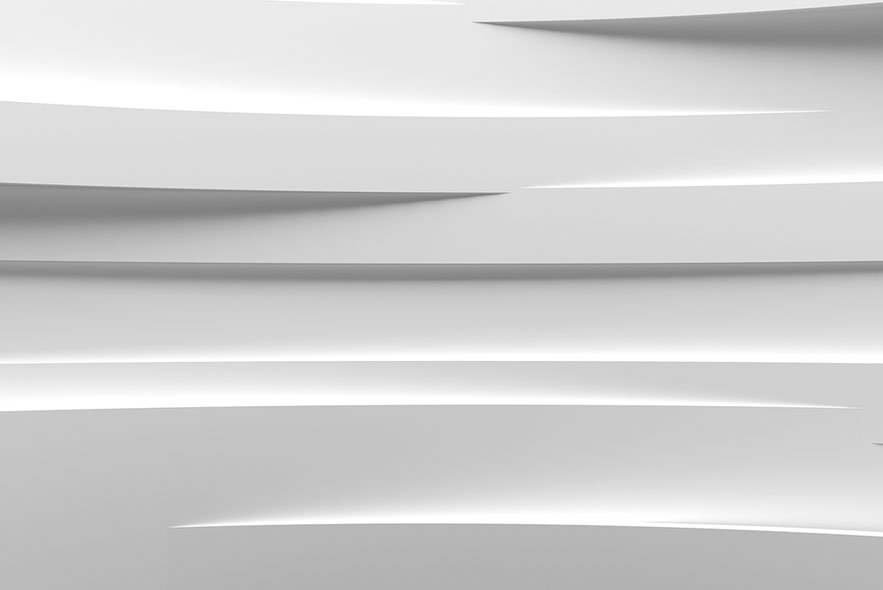
What is augmented analytics?
Augmented analytics is the use of machine learning (ML) and natural language processing (NLP) to enhance data analytics, data sharing and business intelligence.
Data analytics software can integrate augmented analytics tools to handle large data sets. Organizations can enter raw data source information into these platforms that scrub, parse and return key data for analysis. The use of ML and NLP enables augmented analytics tools to understand and interact with data organically as well as identify valuable or unusual trends.
The data analytics field is complex and generally requires a data scientist to extract any value from big data. This complexity is in part due to the fact that data must be gathered from several disparate sources, such as web analytics, marketing releases and social media posts. Collecting the data is just the first step; it must also be prepared for analysis by being organized and refined before the analyst or data scientist can glean useful insights. The results are then communicated to the organization along with action plans to capitalize on these insights.
Due to the manual effort required for these tasks, data scientists are currently in high demand, and their services can be impractically expensive for some businesses. It's estimated that a data scientist can spend as much as 80% of their time gathering, preparing and cleaning up data. This is where augmented analytics can be implemented. With the addition of machine learning to data analytics, many of the time-consuming tasks of data collection and data preparation can be done quickly, automatically and with fewer errors. As a result, data scientists can spend more time searching for actionable insights.
How does augmented analytics work?
Gartner introduced the concept of augmented intelligence, an overarching concept to augmented analytics, in its 2017 "Hype Cycle for Emerging Technologies." Put simply, augmented analytics amplifies human intelligence; it brings out the best in human thinking by filling in gaps and stimulating additional insights. This is accomplished through artificial intelligence -- using ML to deeply examine large data sets, seeking out subtle patterns in the data, and using statistical analysis to surface trends and relationships in the patterns.
Features of augmented analytics
A look at the key features of augmented analytics, including the following, helps to clarify its capabilities and deepens the understanding of its functionality:
- Smart data preparation. Machine learning requires that large data sets be cleaned -- with outliers and unreliable data items removed -- and transformed for uniformity. These processes are generally automated and are portable to other enterprise operations, raising data quality standards across the board.
- Automatic data identification. AI-driven identification of data attributes enables users to extract relevant data from multiple sources, both structured and unstructured, enhancing the variety of data available for analysis.
- Data visualization. Augmented analytics typically uses highly versatile data visualization tools as its primary outputs. Platforms like Tableau and Microsoft Power BI offer tool sets with a broad range of user-interactive graphs and plots, enabling ad hoc drill down and analysis of the numbers.
- NLP and natural language interaction. Machine learning has enabled the proliferation of natural language processing, which, in turn, has made augmented analytics accessible to many nonexpert business users.
- Statistical techniques. Augmented analytics platforms offer users statistical tools and processes for analyses of all kinds, combined with unprecedented ease of use.
- Recommendations. Also called prescriptive analytics, the trend analysis functions of machine learning systems can produce best options from a range of possible outcomes, offering strong recommendations for decision support.
Benefits of augmented analytics
The list of benefits offered by augmented analytics, in both automated and human-driven tasks, is considerable. The following are some of the most essential advantages:
- Better insights. Augmented analytics platforms can bring more and better data together in a unified picture, using multiple sources, to clarify the how and why of what and when -- enabling higher-quality insights into market events and performance issues.
- Faster insights. The process of acquiring insights is faster, given the user-friendliness and convenience of most augmented analytics platforms, which are built with utility and flexibility in mind.
- A more complete picture. Augmented analytics can fill in the gaps in understanding of events and issues, enabling a better grasp of the big picture -- another important decision support feature.
- Automated operational tasks. Many augmented analytics processes can be automated and embedded in hands-free business operations.
- Insight into customer behaviors. Using augmented analytics to deep-dive customer profiles, history and shopping patterns can provide a unified window into customers and the choices they make.
- More accurate forecasting and prediction. More complex data, along with higher-quality analysis processes, leads to greater predictive power.
Challenges of augmented analytics
Just as augmented analytics has numerous benefits, it also has its challenges. Knowing and understanding the following challenges upfront can help an organization better prepare for implementation:
- Data quality issues. The quality of augmented analytics insights is tied to the quality of the data used to inform them. Garbage in, garbage out means that the data sources feeding augmented analytics systems must be as reliable as possible to begin with; cleaning data improves its utility, not its quality.
- Complexity issues. Augmented analytics simplifies the lives of business analysts and other users, but augmented analytics platforms themselves aren't simple at all; implementing and maintaining them requires the ability to grapple with their complexity.
- Data literacy. Just as the platforms require expertise, so do the handling and interpretation of analytics themselves. It doesn't take a data scientist to make effective use of analytics, but a firm understanding of the data, its proper use and its lineage is essential.
- Data governance. Effective data governance is no simple task even in an organization that doesn't use augmented analytics capabilities, but in one that does, it's even more essential. Proper curation and governance of in-house data sources and the import of external data is a major key to success.
Augmented analytics vs. automation
Digital technology has automated many processes to free up human hands for other tasks. This occurs in augmented analytics platforms as well to prepare data for machine learning. Augmented analytics, in turn, can be used to automate decision-making processes. The distinctions are that some automated processes in the enterprise don't involve analytics; some decision-making processes can be automated if augmented analytics is embedded in them; and augmented analytics itself requires the use of automation to make ML practical in the first place.
Augmented analytics key use cases
Augmented analytics can be used across various departments, including from the boardroom to the mail room. The following are examples of use cases:
- It can be used for live data exploration during board meetings and other presentations at the C-suite level.
- IT departments and manufacturing operations can use it to detect impending bottlenecks and performance issues.
- Sales teams can use it for deep trend data analysis.
- Marketing groups can analyze campaign effectiveness and customer behaviors.
- Supply chain managers can perform efficiency analysis on delivery and troubleshoot supply chain performance issues.
- Hotels, resorts and other hospitality industry providers can employ it to personalize travel experience and cultivate appealing customer offers.
Data collection is vital in manufacturing to resolve bottlenecks and reduce costs. Learn what data collection technologies companies can use to improve their production line operations.