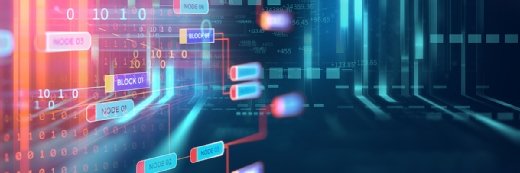
Getty Images/iStockphoto
Mobile Devices, Geolocation Data Help Predict COVID-19 Outbreaks
Geolocation data collected from mobile devices helped researchers predict COVID-19 outbreaks by determining if people practiced social distancing or not, a study revealed.
Researchers used mobile devices to capture geolocation data and monitor close contact between people to predict COVID-19 outbreaks across the state of Connecticut, according to a study published in Science Advances.
Close contact is the primary way that an individual can get infected with the coronavirus. At the beginning of the pandemic, the Centers for Disease Control and Prevention (CDC) recommended that people keep at least six feet of distance between them and others while in public settings.
Researchers from the Yale School of Public Health, the Connecticut Department of Public Health, the CDC, and Whitespace Ltd., a spatial data analytics firm, leveraged mobile devices to track whether people were social distancing.
Study participants consented to using a third-party application that collected their geolocation data. The frequency of data was partly dependent on a mobile device’s operating system and how often users interacted with the app.
The study results are based on geolocation data gathered from a total of 788,842 unique device IDs between February 2020 and January 2021.
“We measured close interpersonal contact within a six-foot radius everywhere in Connecticut using mobile device geolocation data over the course of an entire year,” Forrest Crawford, PhD, an associate professor of biostatistics at the Yale School of Public Health and lead author of the study, stated in a press release. “This effort gave Connecticut epidemiologists and policymakers insight to people’s social distancing behavior statewide.”
An algorithm used the geolocation data to determine when mobile devices were within six feet of each other, thus confirming when individuals had close contact with others.
Researchers incorporated that data into a standard COVID-19 transmission model, which predicted COVID-19 case levels in the towns and cities where the close contact occurred.
According to the study, researchers predicted an initial wave of COVID-19 cases in Connecticut from March to April 2020 by using geolocation data. They also predicted the drop in statewide cases from June to August and localized outbreaks in August and September.
By monitoring close contacts and predicting COVID-19 cases, individuals can be notified in a timelier manner and public health officials can act accordingly. For example, officials could take steps to improve social distancing measures in communities that had high contact rates. They could also work to ensure mask-wearing, adequate ventilation, and cleaning protocols in these locations.
Further, public health officials could use the close contact results to increase testing in certain areas in order to achieve earlier and more complete detection of cases, which may help stop future outbreaks.
Past studies have used mobility metrics to track social distancing and possible COVID-19 transmission, but they may not always produce the most accurate measures, according to the Yale researchers.
“Mobility metrics often measure distance traveled or time spent away from a location, such as your home,” Crawford said. “But we all know it’s possible to move around a lot and still not get very close to other people. So, mobility metrics are not a great proxy for transmission risk. We feel close contact predicts infections and local outbreaks better.”
Additionally, health officials tend to use metrics like surveillance data and cases, hospitalization, and death counts to monitor the spread of the coronavirus — processes that may lag disease transmission by days or weeks.
Using mobile devices that collect geolocation data and note close contacts can lead to a timelier prediction of cases, especially if COVID-19 test availability is low, researchers said.
“The contact rate we developed in this study can reveal high-contact conditions likely to spawn local outbreaks and areas where residents are at high transmission risk days or weeks before the resulting cases are detected through testing, traditional case investigations, and contact tracing,” Crawford concluded.