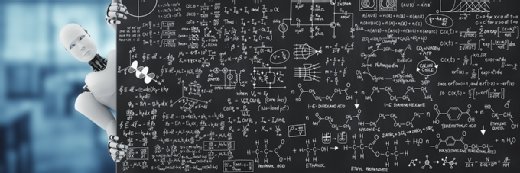
Blue Planet Studio - stock.adobe
How AI can strengthen ESG reporting
Gathering data across an ecosystem of stakeholders is a slog. But with the right tools, it can be easier.
Sustainability reporting is a complex undertaking. AI tools have a potential role in making this easier.
ESG reporting -- also referred to as sustainability reporting -- has become a cornerstone of corporate accountability and regulatory compliance. Traditional methods of reporting that rely heavily on manual data collection from siloed systems are unable to handle the growing complexity of ESG data standards and reporting requirements.
Despite enterprise leaders' best efforts, persistent sustainability challenges exist. These include fragmented data sources, manual reporting inefficiencies, unreliable external inputs and greenwashing risks. In short, challenges exist throughout the lifecycle -- from data collection and reporting to validation and assurance.
AI tools can help automate ESG data collection and aggregation, and improve data integrity by detecting inconsistencies and streamlining the compliance process.
Here's more on the challenges of ESG reporting, traditional AI and generative AI (GenAI) use cases, and best practices for C-suite, IT and sustainability leaders.
ESG reporting issues AI can help address
Sustainability reporting is a complex endeavor for many reasons. To name just a few: Many organizational leaders are starting to understand the concept of greenhouse gas emissions or what falls into different emission scopes. In addition, multiple ESG reporting frameworks exist, and the area is rapidly evolving. On top of that, collecting ESG data is riddled with obstacles. It's in this latter area that AI tools can be especially helpful.
Here's a look at some sustainability data collection challenges.
Data silos and gaps
Accurate sustainability reporting depends on access to correct data. However, in most organizations, data is fragmented or missing, or spread across multiple business units, tech debt from legacy IT systems, and multiple geographies.
While data in other domains -- for example, customer data or financial reporting data -- has also been fragmented, enterprise and IT leaders have made efforts to integrate or establish centralized reporting systems due to regulatory requirements or business needs.
In contrast, ESG reporting remains a voluntary effort in many countries. Even in regions where there are reporting mandates, sustainability reporting initiatives are relatively new, so enterprises are still working to improve their efforts, including selecting the right tech.
Unreliable external data sources and greenwashing
ESG data consists of not just internal enterprise data but also includes data external to the enterprise, such as supplier data and disclosures, and third-party reports. Just as with internal data, the quality of external data can be unreliable, inconsistent or even missing. Greenwashing, or exaggerated sustainability claims, by partners or suppliers is also a possibility.
Manual reporting and errors
ESG reports are often compiled manually. This method is not only error-prone but also takes much longer in comparison to automated methods.
Resource constraints and bottlenecks
Although ESG teams are often small, they are charged with compiling complex reports for multiple stakeholders and might have to deal with a variety of bottlenecks.
AI in ESG reporting: Use cases
AI's strengths and capabilities are well-matched to address the challenges of ESG reporting. Both traditional AI and generative AI can help support ESG efforts, although it's important to balance that with environmental costs.
Traditional AI use cases
Traditional AI focuses on pattern recognition, automation and predictive analytics. Here are four use cases for sustainability reporting:
- Data cleansing and standardization. Identifying and correcting inconsistencies in ESG data.
- Data collection automation. Extracting ESG metrics from internal databases and external reports.
- Predictive insights. Forecasting compliance gaps and risks.
- Fraud and greenwashing detection. Scanning reports for inconsistencies and flagging misleading claims.
Generative AI use cases
GenAI extends traditional AI by creating human-like text, summarizing insights and generating reports. In ESG, GenAI use cases include the following:
- Narrative generation. Producing ESG reports based on structured data.
- Contextual analysis. Summarizing complex documents and extracting key risks.
- Regulatory alignment. Generating ESG disclosures tailored to evolving regulations and requirements of key stakeholders.
In short, traditional AI enhances data processing, data quality, and analytics, while GenAI enables automated report generation and can be used for contextual and personalized insights.
Using AI in ESG reporting: Best practices
Like any technology, AI's usefulness in the realm of sustainability reporting requires forethought and strategy. These five strategies can help IT and sustainability teams use AI tools in a way that supports ESG reporting.
1. Define a unified ESG data architecture.
An important step is implementing an ESG data lake to consolidate information across different business units and systems. AI and automated data ingestion tools can extract ESG metrics from various data sources.
2. Enhance data standardization to ensure data quality.
AI can harmonize and align metrics across different ESG frameworks. AI anomaly detection models can identify errors and any inconsistencies.
3. Deploy AI for fraud detection and identifying risks.
IT can develop models that identify discrepancies in both corporate and supplier disclosures and detect ESG violations prior to public filings.
4. Automate compliance and reporting.
When the foundations -- architecture, standardization and detection models -- are in place, there is room for more automated reporting. Teams can use GenAI to generate ESG disclosures aligned to regulations as they continue to evolve. Humans need to verify the output of GenAI, as it can hallucinate. But even with this quality check in place, AI has the potential to boost productivity. For example, AI can help monitor reporting consistency across geographies.
5. Integrate insights into business decision-making.
Beyond reporting, with higher quality ESG data now available, AI can execute scenario analyses related to climate risks and supply chain disruptions and serve as a valuable input for CXO decision-making.
Kashyap Kompella is an industry analyst, author, educator and AI adviser to leading companies and startups across the U.S., Europe and the Asia-Pacific regions. Currently, he is CEO of RPA2AI Research, a global technology industry analyst firm.