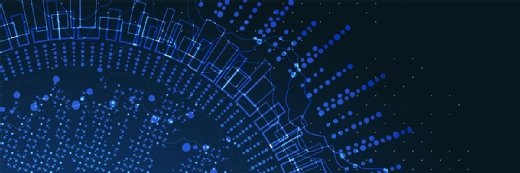
KOHb - Getty Images
How to evaluate AI for Windows Server environments
Organizations tasked with assessing AI workloads on Windows Server must weigh all the variables to determine the best approach to adopt this budding technology.
The rise of AI has captured the imagination of business leaders, but for IT managers who work in Windows Server environments, turning the hype into reality is a challenge.
Implementing AI requires examining the operational and business considerations to determine if running these deployments in the data center makes more sense than putting these workloads in the cloud. The upcoming Windows Server 2025 release and its feature improvements in AI could spark more interest in using existing infrastructure to roll out AI-based projects to execute organizations' strategic goals. This article touches on the possibilities of AI with a closer look at the process many organizations must undergo to determine the optimal way to take advantage of this technology.
Why should a business consider implementing AI?
AI has no shortage of evangelists who tout its ability to solve a range of problems, such as carrying out predictive maintenance to avoid unexpected downtime, monitoring applications and analyzing data.
Incorporating AI could transform businesses in several ways, but organizations must understand where AI brings value and where human intelligence is still necessary. AI outperforms humans when processing large amounts of data, identifying patterns and automating repetitive tasks. But when it comes to creativity, nuanced decision-making or human-driven tasks, AI has its limitations.
Where AI can be useful and where it is lacking
Analytics. AI-driven analytics tools can process large data sets faster than any human could, turning raw data into actionable insights. In business scenarios, AI can analyze customer behavior and supply chain data to optimize pricing strategies, forecast inventory needs and personalize marketing campaigns. With AI's help to crunch numbers, business managers can make smarter, data-driven decisions to boost profitability and operational efficiency.
IT operations. AI can identify and automate labor-intensive IT jobs, such as managing patches, running maintenance scripts or automating an action in response to declining system health. For example, if an IT performance metric exceeds the limit set by the administrator, then AI can automatically correct the problem without human intervention. For an IT team that manages hundreds or thousands of systems, this can be a tremendous benefit. Similarly, AI-powered chatbots can manage common help desk requests to let IT teams focus on more strategic tasks.
Where AI falls short. While AI is excellent for repetitive tasks and handling data, it's not always the right fit. In situations that are not static, such as negotiating agreements or managing complex stakeholder relationships, AI lacks the human intuition that can be necessary to address unique issues. Similarly, AI struggles with regulatory and compliance environments that need specialized knowledge or ethical considerations. Creativity and strategic development -- areas that call for fresh thinking and innovative problem-solving -- can be beyond AI's scope.
Cost-benefit analysis and ROI
Analyzing the financial implications and potential return on investment (ROI) is crucial for any IT project and AI is no different. Measuring ROI for AI projects typically involves assessing time savings, efficiency gains and the ability to shift staff toward higher-value tasks. But how do you measure these effectively?
For instance, deploying AI to automate customer service with a large language model (LLM) can deliver quick ROI by significantly reducing the workload for support teams. However, specialized AI applications, such as those used in niche manufacturing tasks, may require more substantial upfront investment and provide mixed value due to shifting workloads, changing service demands or evolving project requirements.
A clear cost-benefit analysis starts with defining tangible outcomes, setting measurable metrics linked to business goals and comparing these to the overall investment in AI technology, infrastructure and training. While ROI is often measured financially -- such as determining the time it takes for cost savings to exceed the project's investment -- it can also be evaluated in terms of time-to-value. This could mean improvements in work production, higher sales or increased customer satisfaction.
Microsoft offers several resources to assist with calculating ROI for AI deployments. The company commissioned Forrester to produce Total Economic Impact (TEI) studies and developed its AI Business School provide frameworks to assess AI's financial impact, focusing on automation, productivity gains and cost reductions. With Microsoft tools such as Cost Management and Cloud Cost Optimization services for Azure, IT managers can analyze potential cost savings, particularly related to cloud infrastructure, resource optimization and scaling. These resources include customizable templates and case studies to help IT leaders build business cases and project scopes tailored to their needs.
Key elements that factor in the cost-benefit and ROI of AI deployments include deployment models, model selection and complexity. For instance, the decision between cloud vs. on-premises deployments can significantly affect costs. Cloud-based AI services provide scalability and flexible pay-as-you-go pricing, making them more adaptable and cost-efficient for handling dynamic workloads. On-premises AI might have higher upfront costs due to hardware investments but lower long-term expenses for large-scale deployments with stable workloads.
Similarly, the choice between custom-built AI models vs. prebuilt models influences ROI. Custom-built models require higher investments in development, training and data collection but may deliver more tailored insights. Prebuilt models, while quicker to implement and more cost-effective initially, may lack the deep business-specific insights needed for certain tasks. The type of AI model -- whether a basic LLM or a specialized data analytics model -- also plays a role in determining financial return based on the complexity of the business case.
Proof of concept or pilot programs are effective methods to evaluate AI's value before committing to full-scale deployment. These controlled tests measure performance, optimize configurations and assess AI's effect on real-world operations without the financial risk of a large-scale rollout.
The upcoming Windows Server 2025 will usher in new features designed to optimize AI deployments and reduce costs. With tighter integration with Azure, enhanced virtualization capabilities, and better support for AI workloads, it offers several cost-saving benefits. Features such as improved resource allocation and advanced containerization options can help reduce the infrastructure footprint to maximize efficiency in both on-premises and hybrid environments. AI-powered automation and monitoring tools in Windows Server 2025 will assist with server management with the potential to reduce manual intervention and optimize resource use throughout AI operations.
What are the operational considerations for deploying AI?
Once a business commits to adopting AI, IT teams must address several operational considerations for a successful deployment. For teams managing Windows Server environments, the challenges are both technical and logistical, requiring careful planning and alignment with the organization's goals. Key considerations include upskilling, security protocols, environmental impact and supply chain dependencies.
Implications for IT teams
Skill sets and upskilling needs. AI deployments can introduce a range of new technical requirements IT teams must manage, such as machine learning frameworks, data processing tools and automation software. Upskilling existing staff through AI-specific training or certifications from Microsoft is crucial to ensure IT teams can integrate AI effectively with the existing infrastructure.
Security considerations. Security is a paramount concern when deploying AI, especially when sensitive data or intellectual property is involved. IT teams must implement a comprehensive set of security controls, both soft -- policies and training -- and hard -- tools and firewalls -- to protect data integrity. Data loss prevention and access management policies are essential for governing data flow and preventing unauthorized access. AI models trained on sensitive data need continuous monitoring to prevent vulnerabilities. Strict policies must also be enforced to avoid leaks of proprietary or confidential data through AI output.
Environmental impact. AI workloads are notoriously resource-intensive, which can have significant environmental consequences. However, Microsoft attempts to address this issue in Windows Server 2025 with energy-efficient AI processing capabilities designed to reduce power consumption. IT teams can use these sustainability features to optimize server performance and align with the company's broader environmental goals. An organization can use energy-efficient processors and enhanced resource management tools to lower its environmental footprint while still meeting the computational demands of modern AI workloads.
Supply chain dependencies. AI deployments -- especially those in on-premises environments -- often rely on specialized hardware, such as GPUs or AI-specific processing units. These components are subject to supply chain risks, including potential delays or shortages that could slow AI projects. As best they can, IT teams must prepare for these contingencies and explore their options, such as using cloud-based AI infrastructure as an interim measure.
Microsoft adds AI enhancements to Windows Server 2025
Windows Server 2025, due out in late 2024, introduces a range of features designed to optimize AI workloads, making it an attractive option for IT managers who want to scale AI initiatives efficiently.
One standout feature is the update to GPU partitioning (GPU-P) through which multiple VMs share a single physical GPU. This capability maximizes resources to run several AI workloads concurrently without needing multiple dedicated GPUs. Additionally, VMs using GPU partitions can automatically reboot on another node during system migrations or when hardware issues arise to avoid disruption of critical AI tasks.
Another key enhancement in Windows Server 2025 is the live migration for GPU-P to let IT teams balance AI workloads and perform hardware or software maintenance without interrupting running VMs. These features, coupled with Nvidia's advanced GPU support, make Windows Server 2025 well-suited for resource-intensive AI tasks, such as training and inferencing. With centralized management tools available via Windows Admin Center, IT managers can effectively manage and optimize GPU resources across on-premises or hybrid environments to keep AI deployments scalable and cost-efficient.
Should AI workloads run on-premises or in the cloud?
One key decision for most IT projects is whether to deploy on-premises or in the cloud. Both options have pros and cons, which need to be evaluated based on available resources, scalability requirements and risk management strategies. On-premises deployments provide full infrastructure control but come with higher upfront costs and limited scalability. A cloud-based deployment gives flexibility, capacity scaling and cost efficiency but may introduce concerns with data security and long-term costs.
Understanding these operational considerations will help IT teams navigate the complexities of AI deployment on Windows Server to determine what meets the organization's technical and strategic needs. The following table compares the pros and cons for deploying AI in on-premises and cloud-based environments.
Factors | On-premises deployment | Cloud deployment | ||||
Resources |
|
|
||||
Budget |
|
|
||||
Scalability |
|
|
||||
Risk |
|
|
Deploying AI in Windows Server environments has the potential to improve efficiency, security and data-driven decision-making. However, understanding the business objectives and operational considerations -- from cost-benefit analysis to skill set requirements and deployment models -- is essential to make this deployment a strategic asset that drives long-term value.
Dwayne Rendell is a senior technical customer success manager for an Australian cybersecurity MSP. He has more than 15 years of experience in IT and specializes in service delivery, value realization and operations management of digital service portfolios. Dwayne has experience in multiple sectors, including health and government. He holds an MBA from the Australian Institute of Business.