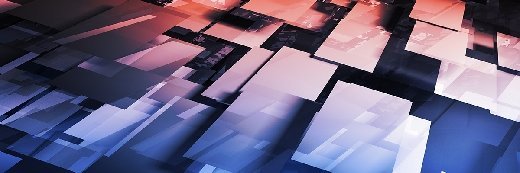
kentoh - Fotolia
You can now run a Nvidia vGPU on VMware infrastructure
VMware's partnership with Nvidia aims to make machine learning more accessible. By pairing Nvidia vGPUs with vSphere, companies can accelerate computing and maintain management.
Thanks to a new chapter in the partnership between VMware and Nvidia known as Project Monterey, organizations can now run compute-intensive applications such as AI and machine learning workloads on Nvidia vGPUs -- and use VMware vSphere to manage them.
AI, deep learning (DL) and machine learning (ML) workloads have traditionally been confined to the CPU, but the Nvidia Virtual Compute Server (vCS) enables IT administrators to shift those workloads to GPUs or virtual GPUs (vGPUs) and manage those workloads through vSphere. This strategy aims to improve GPU utilization, tighten security and simplify management.
"AI, DL [and] ML … are all very compute-intensive workloads and require a considerable amount of computing," said Raj Rao, senior director of product management at Nvidia in a session called "Best practices to run ML and compute workflows with Nvidia vGPU on vSphere." He added: "A general piece of hardware cannot just take on and deliver these requirements."
With Project Monterey, VMware aims to eventually ease development and delivery of machine learning in vSphere environments. For now, it seeks to simply accelerate computing for those environments with the help of vCS and vGPUs.
Nvidia GPUs feature tensor cores, which can activate the large matrix operations AI requires. Its GPUs also feature advanced compute cores for more general-purpose multitasking compute workloads. These GPUs are generally available in all popular OEM servers; organizations can deploy them on-premises or in the cloud. Virtualizing GPUs extracts functionality, performance and reliability from hardware GPUs.
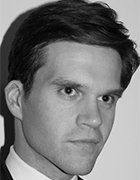
"This is part of a general trend toward hardware accelerators for virtualization," said Paul Delory, research director at Gartner, a research and advisory firm based in Stamford, Conn. "We're increasingly offloading specialty functionality to dedicated hardware that's purpose-built for one task."
Managing vGPUs with vSphere
With the newfound ability to manage vGPUs through vSphere, admins can enable diverse workloads, such as running Windows and Linux VMs on the same host. VMware customers increasingly use vGPUs in edge computing, and 5G GPU computing presents an emerging use case for vSphere-managed vGPUs.
Admins can also use vGPUs in vSphere to accelerate graphics workloads; encode and decode VMware Horizon workloads; run machine learning, deep learning and high-performance computing workloads; and develop augmented reality or virtual reality applications.
VSphere-managed vGPUs also add efficiency to processes such as vMotion for vGPU-enabled VMs. Admins can manage GPUs and vGPUs with vSphere, and then vMotion workloads using those GPUs and vGPUs in a more streamlined manner.
"Machine learning training or high-performance computing jobs can take days," said Uday Kurkure, staff engineer at VMware. "If you were to do server maintenance, you need to stop the jobs and bring the server down … bring up your server again and restart the job. But … instead of shutting down your jobs and shutting down your server, you could be vMotion-ing those jobs to another host … saving days of work."
To set up a Nvidia vGPU on vSphere, install a Nvidia GPU on a host. Install Nvidia vGPU Manager on the hypervisor, which runs atop the host, to virtualize the underlying GPU. Admins can then run multiple VMs -- with different OSes, such as Windows or Linux -- that access the same virtualized GPU. These hosts can then run high-performance computing or machine learning workloads with speed and efficiency.
Machine learning in vSphere and virtual environments
Using vGPUs can enable more efficient machine learning training for those with access to that technology. Admins can train their machine learning applications while running other workloads in the data center and drastically reduce the time it takes to train the machine learning applications. For example, a complex language modeling workload for word prediction can take up to 56 hours to train using only a CPU, but takes just eight hours with a vGPU, according to Kurkure. A vGPU also has a 4% overhead in training time compared to a native GPU. However, machine learning still might remain inaccessible and on the horizon for most organizations.
"The benefit of Project Monterey for AI or ML workloads is getting them access to GPUs," Delory said. "[But] right now, you either have to install GPUs in all your hosts -- which is expensive -- or dedicate hardware to AI or ML workloads -- which is complex and expensive."