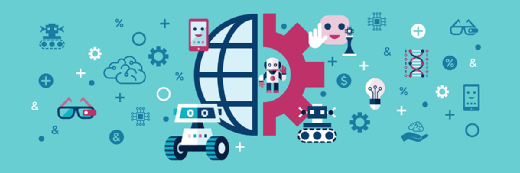
Guidelines to implement storage for AI workloads
Organizations with AI-enabled projects need an effective way to store, retrieve and share massive amounts of complex data. These tips will help meet AI data storage challenges.
Despite increasing enterprise adoption, AI technology remains an evolving and challenging concept. For most organizations, there's still much to learn about AI and its storage requirements.
Fortunately, there are certain guidelines and best practices IT teams can follow to ensure efficient storage for AI workloads.
The need for AI storage
As AI applications expand across industries, the technology will become more pervasive, said Adrian Zidaritz, author of AIbluedot.com, a website dedicated to AI topics, and the former head of data science at Collective[i], an AI consulting firm.
"Therefore, it's essential for enterprises to match their AI-based projects with a compatible and efficient storage technology," Zidaritz said.
Storage for AI makes better use of AI-based workload data and enhances the user experience through easily shared data, said Anthony Ciarlo, AI leader, ecosystems and alliances, at business and technology advisory firm Deloitte Consulting.
"Enterprise organizations with the right data strategy have faster reporting times, increased agility and a broader depth of the data they can analyze compared to traditional environments," Ciarlo said.
Key benefits include:
- The ability to analyze and use large quantities of data at a fraction of the time of traditional reporting.
- Enhanced security measures and access protocols. Storage for AI enables organizations to put rules in place that determine who has access and when. This provides an easy data exchange and ensures a more secure data set, which reduces both operating and compliance costs.
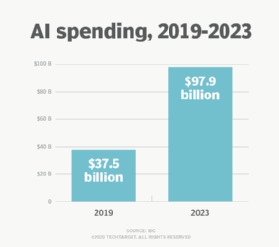
A mature data engineering infrastructure enables users to find the information they need faster, said John Langton, director of applied data science at management advisory firm Wolters Kluwer.
"It also enables the use of ... data for different purposes, from reporting to analytics to more advanced AI projects," Langton said.
Effective storage and data models -- as well as extract, transform and load processes -- will help users streamline activities so organizations can focus on analytics, rather than transforming data.
Deploy storage for AI
Most AI projects use a combination of block and object storage, said Goutham Belliappa, vice president for AI engineering at business and IT consulting firm Capgemini.
"A block store works best for direct user interaction, like a file system, a database or any application that requires high-performing reads and writes," Belliappa said. "Object storage, on the other hand, offers durability and flexibility where applications -- like a graph store, video player or some other mechanism that can understand the index and locations -- can access data that's written once and read many times."
Match the storage type to the use, Belliappa said.
"Avoid abstractions that, for instance, give you a block storage-like structure on top of object storage, where you end up getting the worst of both," he said.
Work to limit file sizes in complex environments, such as those that use Spark for parallel processing and parquet files. The way files are partitioned will also affect performance dramatically and lead to faster data retrieval.
Consider the cloud
The cloud -- more specifically, the public cloud -- should be top of mind during an evaluation of AI storage strategies, Ciarlo said.
"Here, you will find the latest innovations and capabilities that are only available natively in the cloud," he said.
Object storage, for instance, is oriented for the cloud, and the cloud enables data sharing and exchange.
"Without the advancements of cloud technologies, AI storage would not be an efficient or complete strategy," Ciarlo said.