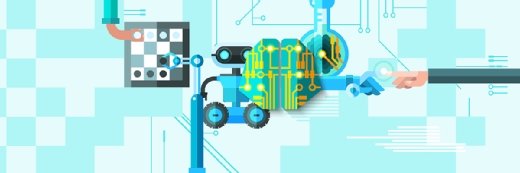
stock.adobe.com
Why the future of AI storage may have to exclude flash
Discover why large, high-node count and hard disk-only scale-out storage architectures address the ever-growing capacity demands and challenges of AI architectures and workloads.
Many organizations will invest in AI over the next few years. At a minimum, these investments will be for IT operations, where IT will use AI to process the mounds of telemetry data continually generated by systems within the data center. More than likely, though, most enterprises will start to invest in AI applications that will help them run their business better.
The storage infrastructure that supports these initiatives is critical to the success of the project, however. If enterprises don't deploy adequate AI storage to support their AI workloads, these investments will result in more buck and less bang.
Why is storage so critical to AI?
Most AI workloads become "intelligent" by processing mountains of information so they can learn the behaviors of the environments they will later manage. AI architectures tend to follow a conventional design. Most are a cluster of compute nodes with some or all of those nodes containing GPUs. Each GPU delivers the performance of up to 100 CPUs, but these GPUs are also more expensive than off-the-shelf CPUs.
An essential role of the AI storage infrastructure is to ensure these GPUs are fed continuously with data so they are never idle. The data they process is typically millions, if not billions, of relatively small files, often created by a sensor or IoT devices. As a result, AI workloads often have an I/O pattern that is a mix of sequential and random reads.
Another challenge is storage requirements. For AI to become more sophisticated -- smarter -- it needs to process more and more data. These high storage capacity demands mean AI projects that start at 100 TB can quickly scale to 100 petabytes (PB) within a few years. AI capacities in the 300 PB to 400 PB range are becoming increasingly common.
In its early stages, many AI projects counted on a shared all-flash array to deliver the performance required to keep expensive GPUs busy. Today, many AI projects can take full advantage of the high performance and low latency of NVMe-oF systems that offer high performance, even over the network. As these environments scale and attempt to reach high levels of autonomy, they need ever more compute and GPU resources and significantly more storage space.
Next-generation AI storage infrastructure needs to scale to meet the capacity demands of higher-level autonomy. It also needs to scale to meet the performance demands of scale-out compute clusters. As an organization scales its AI workloads and IT adds more GPU-powered nodes to these clusters, I/O patterns become more parallel.
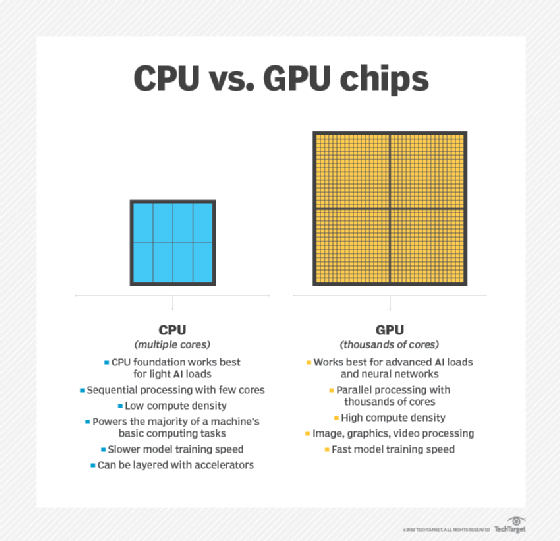
Scale-out AI storage
A scale-out storage architecture solves the capacity challenges created by next-generation AI workloads. Additionally, a scale-out architecture, if it enables direct access to specific storage nodes within the storage cluster, can meet the parallel I/O demand of advanced AI workloads. However, parallel access requires a new kind of file system so the storage cluster doesn't bottleneck with a few nodes managing access.
Given that most AI workloads will demand dozens, if not hundreds of petabytes of capacity, it is unlikely storage planners can continue to use all-flash as the only storage tier within the AI storage infrastructure. While the price of flash has come down significantly, high-capacity HDDs are still far less expensive. The modern AI architecture, at least for now, needs to manage both flash and disk and move data transparently between those tiers. However, management of those tiers needs to be automated.
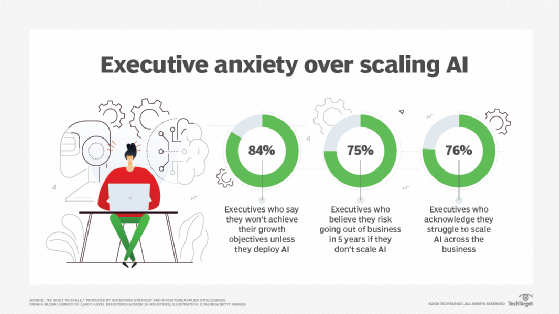
In some cases, given the size of the workload's data set, an organization may be better off building a large, high node count and hard disk-only cluster. That's because these workloads are so large they completely overrun any cache tier. Also, tiering data adds overhead to the storage software, slowing it down. A high-node count, hard disk-only storage cluster may provide enough parallel performance to continue to deliver data to GPUs at speeds it can process.
AI requires HPC-level storage
As AI workloads mature, AI storage infrastructures will look more like high-performance computing (HPC) storage systems than traditional enterprise storage. These infrastructures will almost certainly be scale-out in design and -- because of massive capacity demands -- contain HDDs and potentially tape, depending on the workload profile.
Meanwhile, flash, because of cost and how quickly AI and machine learning can consume it, might soon be rendered useless as a storage medium for these systems. At minimum, flash may end up playing only a small role in AI storage architectures as these environments transition into becoming more a combination of RAM, hard disks and tape.