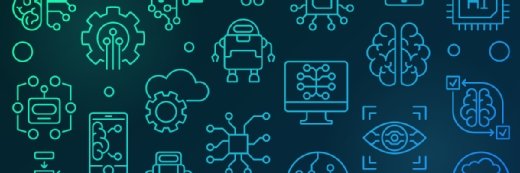
Weka adds multi-cloud support for AI, ML apps
With Weka 4.0, the parallel file system provider will support four major public cloud vendors while consolidating high performance workloads and adding a data reduction feature.
Weka has released a platform update for multi-cloud customers with AI and machine learning workloads that addresses a need for consistent data performance regardless of the data's location.
The update, Weka 4.0, adds features such as a similarity-based data reduction, new cost-effective options and simplicity. The Weka platform is a parallel file system that lets customers store data across multiple networked servers.
As more enterprises run AI and machine learning (ML) workloads, they end up with huge data sets, said Eric Burgener, analyst at IDC, a market research firm in Framingham, Mass. Feeding more data to AI and ML generates better results. If customers run something on one of the major public clouds, it is to any vendor's advantage to offer native support on all the major cloud providers, since so many enterprises are multi-cloud environments, Burgener said.
IDC pegs the storage market for AI and ML workloads at about $5.4 billion by 2024 with a five-year compound annual growth rate of 18.6%. AI is the fastest-growing segment, Burgener said.
Moving to multi-cloud
Weka, formerly WekaIO, began on AWS and could run locally on commodity server-based storage. With this update, Weka advances the number of platforms it will support to include Oracle, Google and Microsoft.
"[Weka now has] a native file system offering on the three most important public clouds to the North American market," Burgener said.

Enterprises frequently use different cloud providers because not every provider supports workloads in quite the same way, due to differences in performance, scale or cost, said Scott Sinclair, senior analyst at Enterprise Strategy Group, a division of TechTarget. Weka reports that its Weka 4.0 release can process 17 million IOPS and 2 TBps as top-end numbers.
"To get high-level performance across a multi-cloud environment when you're dealing with lots of interconnections is really complex," Sinclair said.
Data that moves between clouds is where costs can spike, but this strategy is typical today, Sinclair said. Companies create new applications or workloads in AWS, for example, but the data might live in Azure, so it must be copied or moved, which consumes time and incurs egress fees.
Data reduction and consolidation
The latest Weka release also introduces data reduction, a feature that identifies and compresses similar data while keeping dissimilar data intact, much like one of its competitors, Vast Data. As Weka expands beyond its initial performance goals and looks to serve diverse workloads, it needs to add features such as data reduction to compete in new markets, IDC's Burgener said.
Consolidation means more than storing all data on a single system, as different storage systems address different needs, he said. The initial capture for AI workloads sometimes requires very high throughput from a parallel client.
Weka might have some edge over its competitors here, as the platform now lets customers put a single data set on the data store and run multiple applications using different access methods. By contrast, some competitors require customers to have multiple storage systems, Burgener said.
"[Customers won't] spend time migrating large data sets to different storage platforms," he said. "Just leave it on one that can provide all the performance, letting you run various applications simultaneously."