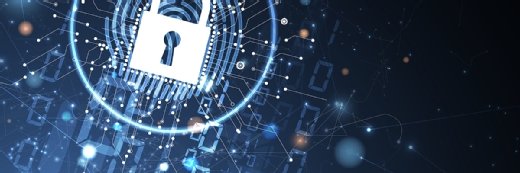
kras99 - stock.adobe.com
How AI is reshaping threat intelligence
As promising as AI technology is for threat intelligence, organizations grapple with a long learning curve and other challenges that could impede successful adoption.
Cybersecurity is no stranger to AI. Many organizations have harnessed the powerful technology to accelerate and improve threat detection, mitigation efforts and incident response across an increasingly difficult threat landscape.
Progress in generative, synthetic and other types of AI is becoming especially instrumental in gathering and discerning threat intelligence data, as evidenced by the following list.
There's a catch, however -- actually, a few catches. AI might not be the silver-bullet solution to all things threat intelligence and security.
Let's examine how AI improves threat intelligence and then discuss some cautions to using the technology.
How AI helps threat intelligence
AI is reshaping how security operations teams collect, analyze and use threat intelligence in the following ways:
- Reduced false positives. Machine learning technology, a discipline of AI, has been used for threat intelligence processes for some time. It can accurately discern real cybersecurity threats from harmless anomalies, reducing the number of false positive alerts that flood security systems.
- Expedited threat identification. Automated tools can parse data faster than humans can, providing real-time alerts to security events. This enables teams to make informed decisions and respond more quickly, minimizing operational disruptions and losses.
- Feed correlation. AI can compare and analyze data across multiple threat intelligence feeds to identify patterns and provide content from a variety and large amount of data.
- Tracked TTPs. Natural language processing (NLP) is a type of machine learning technology that understands human language. Customized NLP algorithms can correlate threat intelligence data across feeds to continuously track threat actor tactics, techniques and procedures.
- Improved phishing detection. Systems that employ NLP can detect malware, ransomware and other harmful email content, blocking it before it reaches end users.
- Improved customer experience. AI can improve customer trust and satisfaction. Financial institutions, for example, can use AI algorithms to track transactions. Applying learned pattern behavior with AI helps flag fraudulent activity to curb losses and improve clients' experiences.
- Insider threat detection. Applying AI in conjunction with user and entity behavior analytics (UEBA) enables security analysts to spot potentially damaging end-user behavior.
In addition to elevating threat intelligence, AI can help with other cybersecurity controls. Take identity and access management, for example. Using a mix of biometrics, AI and UEBA, organizations can analyze end-user activity in context to shore up authentication and block unauthorized access. This also helps strengthen policy compliance.
Is AI ready for threat intelligence?
As appealing as AI might be as a way to improve threat intelligence, challenges remain:
- Threat actors use AI too. One major concern is that threat actors might benefit more from implementing AI than the security practitioners using it to protect their organizations. Cybercriminals are notoriously creative and advanced, and they are willing to quickly adopt new technologies and methodologies to get ahead of their victims' defenses. For example, AI can help threat actors improve phishing attacks as well as conduct data poisoning or prompt injection attacks to manipulate AI models.
- Limited staff expertise. AI can be difficult to deploy and manage, let alone secure. Staff working with AI models need the proper training and skills to effectively input data and train models, manage and operate tools, and analyze output while also creating secure code and protecting the systems from cyberattacks and vulnerabilities.
- Data quality. AI models need to be fed a lot of high-quality data to accurately detect indicators of compromise and potential threats. Without the proper data or validation, models can return incorrect information or introduce security vulnerabilities. This can result in false positives and false negatives as well as hallucinations. AI models have also been known to introduce biases, another challenge to be aware of when validating data.
- Privacy and compliance. AI and LLMs face privacy issues, including deciding who owns the data and what data can be derived from LLM outputs as well as ensuring trustworthiness of data output. AI-powered tools and processes must have the proper privacy measures in place to ensure the data is safe. This also relates to compliance. Existing and future regulations include AI data guidance, which must be properly navigated and complied with.
- Human augmentation, not replacement. No AI conversation is complete without the question of whether it will replace humans. While it is an extremely useful tool in helping teams understand security vulnerabilities and how to address those shortfalls through policies, best practices and new investments, security teams and organizational leaders must remember that AI threat intelligence supplements, not replaces, skilled personnel. To get the most out of the technology, organizations and their teams must carefully assess the best way to use AI for their business needs. A collaborative balance between humans and AI is key to getting the most out of the information AI-driven threat intelligence provides.
Amy Larsen DeCarlo has covered the IT industry for more than 30 years, as a journalist, editor and analyst. As a principal analyst at GlobalData, she covers managed security and cloud services.
Sharon Shea is executive editor of TechTarget Security.