How AI could change threat detection
AI is changing technology as we know it. Discover how it's already improving organizations' ability to detect cybersecurity threats and how its benefits could grow as AI matures.
AI is now a critical tool in an organization's cybersecurity defense strategy, with expert expectations that AI will boost the enterprise security posture even further as the technology matures.
Although AI is widely considered to be in its infancy, it is already delivering transformational benefits in cybersecurity.
Research confirms that take on AI in cybersecurity. A February 2024 report on AI in cybersecurity from ISC2, a training and certification organization for security professionals, found that 82% of the ISC2 members surveyed said they believe AI will improve job efficiency for them as cybersecurity professionals.
Detecting and blocking threats is among the top five areas where they said AI -- including machine learning (ML) and generative AI (GenAI) -- is supporting their work. The other four areas that AI benefits are user behavior analysis, automation of repetitive tasks, monitoring network traffic/network malware detection and the prediction of weak spots where breaches might occur.
Security team investments in AI aren't surprising, given the impact AI has on enterprise defenses, said Adnan Masood, chief AI architect with UST, a company focused on digital transformation products. "It's very well established that AI in frontline defense is a force multiplier."
This article is part of
What is threat detection and response (TDR)? Complete guide
History of threat detection
Threat detection and response (TDR) is a collection of processes that security teams use to identify and defuse threats -- such as malware, botnets and DDOS attacks -- before they affect their organizations.
Technology teams developed this practice in the late 20th century as they connected more computers to early versions of the internet and discovered that they were vulnerable to viruses and worms.
Early threat detection practices mostly involved identifying "something bad on a device by detecting that it matched a known signature," explained Kayne McGladrey, a senior member of IEEE, a nonprofit professional association, and field CISO at Hyperproof.
This signature-based detection was, and still is, a key part of threat detection, but other rules-based detection practices -- where computer activities are analyzed to determine if they follow set rules -- have become foundational components of threat detection over the years, too.
Enterprise IT and security teams also saw the evolution of technologies that supported their threat detection work. For example, anomaly detection systems emerged around 2000 and added not only new capabilities but more automation, too, thereby eliminating some manual monitoring.
Technologies emerged to monitor threats on not just endpoints but within network traffic and other parts of the IT environment. These include security information and event management (SIEM) systems; security orchestration, automation, and response (SOAR) capabilities; and endpoint, network, extended and managed detection and response tools.
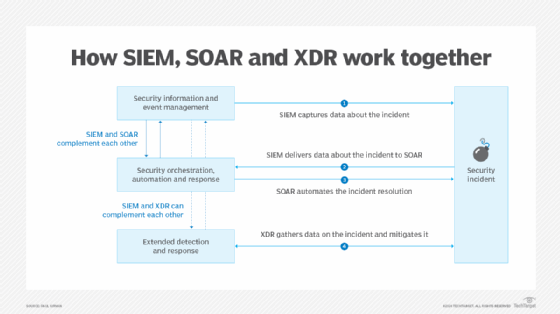
These tools have long used pattern matching and analytics to detect threats, plus automation to aid in response. Now, these tools use AI as well.
"There has been automation in threat detection for a number of years, but we're also seeing more AI in general. We're seeing the large models and machine learning being applied at scale," said Josh Schmidt, partner in charge of the cybersecurity assessment services team at BPM, a professional services firm.
How AI is used in threat detection
AI uses advanced algorithms to shift through and analyze vast amounts of data and does so in near real time. As such, it instantaneously can identify patterns and anomalies that indicate potential threats. This is done at a pace and scale that human analysts cannot match.
ML is a prevalent type of AI used in threat detection, although other forms of AI are used.
"AI is looking [at the IT environment] and asking 'Does this seem like something an attacker would do or something a user wouldn't do?' and alerting on that," said Allie Mellen, principal analyst at research firm Forrester.
For example, user and entity behavior analytics products use AI algorithms and ML to find unusual behaviors that do not match a user or entity's typical pattern, which it does by analyzing vast amounts of data on when, where and how users and entities access and connect across the enterprise network, its software and its hardware.
AI also works to validate whether something that has been identified as a possible threat is truly a threat, Mellen explained, adding: "For detection validation, AI is asking, 'Is it a true positive or is it detecting on benign behavior?'"
An AI-based tool can use different analytical approaches to detect, identify, alert and even automatically remediate a threat, Mellen said. For example, it would use association rule learning, a type of unsupervised learning technique that examines the relationship of one step to others, which can detect an activity whose steps resemble a cyberattack.
Other tools find anomalies or outliers in activities, which could indicate a threat, she said. Still others use clustering, where they map users into different groups to determine what typical behaviors look like so they can find a threat that doesn't belong.
"So, there are layers in how we see ML used. And you can stack these and layer these different methods together to get a more accurate assessment of what's going on," Mellen added.
Additionally, AI is being used to support analysts and improve their work experience as they deal with threats, Mellen said. Most notably, AI is being used to give workers the best step to take next, where the AI alerts analysts to potential threats and then advises them on what actions to take in response, adjusting recommendations as needed.
Nearly all enterprise security teams get AI tools through the security software they buy from vendors. However, Mellen noted that some SIEM vendors are creating a "bring-your-own machine learning model, which allows for better or more targeted detection based on applications being used in the enterprise."
Furthermore, McGladrey said some very large organizations are developing their own AI capabilities. "They're putting their data in a data lake, and they're writing their own algorithms and using machine learning to detect threats," he said, explaining that these organizations believe they can create a more effective threat detection system based on their own environment's data and their own threat intelligence and at a price point better than those vendors offer. He said whether that's true has yet to be determined.
The importance of AI in threat detection
AI helps cybersecurity teams to keep pace better with the volume and velocity of threats coming at their organizations; that's the most prominent benefit of AI in threat detection, experts said. "People can't look through millions of entries to find outliers; that's where AI can shine," Masood noted.
AI also enables many threat detection and response tasks to be automated, which eases the workload that would otherwise fall on security analysts and security operations center (SOC) teams. Perhaps more importantly, AI and automation perform can perform lower-value tasks that might not be done otherwise, given the limited human resources that exist in any organization.
"This takes a huge load off the SOC to evaluate every threat that comes in," said Benjamin Luthy, program director of cybersecurity and adjunct professor for cybersecurity and information technology at Champlain College Online. That, in turn, lets security pros spend more time on higher-value and proactive security tasks, such as threat hunting.
The future of AI in threat detection
AI is expected to continue improving threat detection capabilities as AI matures as a technology. That can be seen with GenAI.
"There is a lot of potential with generative AI," Mellen said, as it excels at understanding how events are sequenced in very complex sequences. This could help advance user behavior analyses or identify threat actor behaviors, she added.
Masood said GenAI also could transform the security analyst's experience by explaining, in everyday language, both the attack pattern the threat detection tools found and response requirements. Similarly, analysts could use GenAI to query the threat detection tools in natural language and also use GenAI to understand unstructured data, thereby giving the analysts better access to information that could aid threat detection and response efforts.
"It won't just raise flags, but it will tell you what specifically is happening. That explainability comes naturally to generative AI," Masood said, adding that the technology and the security world's use of it is not yet mature enough to do all that.
Moreover, AI could advance enough to not only react to threats but perhaps even anticipate them, thereby helping move security teams from a reactive to proactive strategy, Masood added.
Meanwhile, Schmidt said he also believes the continuing evolution of AI will shake up the vendor market as "truly revolutionary players will emerge, and they'll get more investments to help them accelerate technical improvements and advancements."
He said he further expects that -- as AI-related regulations emerge and as such regulations give companies guardrails for when and what data they can share -- companies will more readily share data that can be used to train AI tools to be even more precise in their threat detection and response jobs.
"In the future, as the technology improves and gets more refined, the default capabilities out of the box will be more capable, and that will mean higher rates of threat detection and will help decrease the time to detection for a breach," he explained.
Challenges and limits to using AI in enterprise security
Although the use of AI in threat detection is well established and its use is expected to significantly mature and expand in the future, security leaders cautioned against the tendency to see AI as a savior for enterprise security programs.
Some organizations still struggle to fine-tune the AI capabilities they deploy to their unique IT environments, Mellen and others said, which limits the AI tools' ability to detect as much as it possibly could. Some organizations lack the skills and knowledge to make full use of AI tools, Masood added.
As a result of those organizational limits, security teams sometimes fail to optimize the AI tools they have.
At the same time, some are overestimating the capabilities that AI can or likely will have any time soon, McGladrey, Mellen and others noted.
For example, McGladrey said some promote using AI for threat and risk assessments. He acknowledged that AI might, in fact, help with that task; however, it will produce results that are unlikely to uncover any novel or unique insights for the organization.
"It's not coming up with new thinking," he explained. He similarly questioned claims that AI, as it exists today, could be used to successfully identify zero-day attacks, explaining that the systems aren't built and trained to do that.
Mellen similarly picked up on that theme: "We don't have true intelligence in any meaningful way today." She said AI helps improve threat detection, but it hasn't been revolutionary.
"Has AI had a substantial impact, absolutely. But I'm hesitant to say that because it hasn't had the impact that was promised," Mellen said. "Yes, it is used for detection, which helps security teams, but the part that takes the longest by far is investigation. So, we need support in the investigation process. That's where machine learning and AI should be focused on improving the analyst experience."
At the same time, the bad actors are also harnessing AI to improve their attack capabilities -- a fact that has the security community worried. Indeed, an April 2024 Splunk report found that "while security teams recognize the many benefits of AI, so do threat actors that are unencumbered by laws and policies. When asked whether AI will tip the scales in favor of defenders or adversaries, respondents are almost evenly divided: 45% predict adversaries will benefit most, while 43% say defenders will come out on top."
Not all share that outlook. Schmidt, for one, has a more optimistic take: "I think AI will benefit the defense more than the threat actors."
Mary K. Pratt is an award-winning freelance journalist with a focus on covering enterprise IT and cybersecurity management.