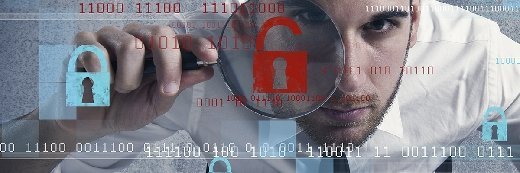
alphaspirit - Fotolia
Give your SIEM system a power boost with machine learning
The enterprise SIEM is still essential to IT defenses, but the addition of AI, in the form of machine learning capabilities, gives it even more potential power.
The hottest topic when it comes to the SIEM system today is the addition of artificial intelligence and machine learning technologies.
Machine learning is when computers can make decisions without needing a comprehensive set of preprogrammed instructions for doing so. The goal is for machine learning technologies to make decisions that would normally require human intelligence, hence the term artificial intelligence. Machine learning also enables computers to improve their decision-making over time, in terms of both accuracy and speed.
Today's SIEM machine learning technologies are relatively immature. This doesn't mean you can't make use of them today, but it does mean they have definite limitations. The following are some tips on how you can make the most of your SIEM machine learning capabilities.
Keep qualified people involved in monitoring and administering the SIEM system. Security in general is a tough field for machine learning because things change very quickly, and it's often unclear which events are benign and which are malicious in a particular environment. Context is often absolutely necessary to differentiate good from bad. Does a series of remote logins to servers indicate a system administrator who is working remotely or an attacker harvesting data? Humans can often review events and innately provide the context that SIEMs do not yet have available. Also, humans need to correct the SIEM's errors so it can learn from its mistakes. If the SIEM learns the wrong lessons, it's going to make bad decisions.
Ensure your SIEM system takes advantage of threat intelligence data. Threat intelligence provides insights into the likely intent of individual IP addresses, domains, websites and other logical entities on the internet. Some SIEM products and services include a particular threat intelligence feed, while others allow customers to add on third-party intelligence feeds of their choosing. Giving your SIEM continuous access to one or more threat intelligence feeds enables machine learning technologies to use the context the threat intelligence delivers. This improves the SIEM's decision-making, particularly in terms of accuracy.
Provide as much event data for the SIEM system as feasible. Machine learning generally works better on larger data sets than smaller ones, so taking advantage of machine learning may require switching from traditional relational databases to big data approaches. That enables the SIEM to collect and retain far more information on events, thereby improving the quality of machine learning. There is a caveat: Because big data is lossy, it may complicate compliance reporting. But this is a known problem with multiple workaround options available.
Carefully consider how confident you should be in allowing the SIEM system to automatically implement decisions it makes through machine learning without a human reviewing and approving those decisions first. Many SIEMs can direct other enterprise security controls to reconfigure themselves in response to a threat, such as blocking network traffic from a malicious IP address or preventing internal hosts from connecting to a particular external domain. Errors in decision-making could inadvertently disrupt benign activity, causing damage and financial loss to your organization.
Ideally, machine learning could make a SIEM system largely self-sufficient, able to identify incidents far better than humans could and able to minimize the need for human involvement. But for now, human supervision and intervention will remain essential.