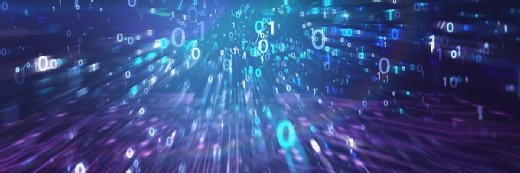
Getty Images
How can SAP's predictive analytics improve SCM?
SAP's predictive analytics could help users with their supply chain management as supply chain problems persist. Learn more about how these capabilities could benefit users.
Big data is driving a greater number of operational decisions than ever before. SAP software's predictive analytics could help users take advantage of its potential and improve their supply chain.
Potential predictive analytics uses for the supply chain include risk mitigation and inventory optimization.
Here's a look at predictive analytics and how SAP's predictive analytics capabilities can help with supply chain management (SCM).
Predictive analytics today
Predictive analytics can help drive business value in a wide range of scenarios, including SCM.
Supply chain managers have been using statistical models for years, including forecasting demand and refining predictive models based on different variables. Now contemporary systems' computational power marks a significant leap forward. Current analytics software can crunch more numbers and factor in more variables to discover new causal relationships.
SAP users gained new predictive analytics capabilities when the company's Integrated Business Planning (IBP) tool debuted in 2014. IBP takes advantage of SAP's HANA in-memory database and enables faster and more comprehensive real-time analytics than IBP's predecessors.
In addition, IBP's Supply Chain Control Tower provides insights into particular metrics and alerts users when supply chain conditions fall outside predetermined parameters. This enables a "management by exception" approach in which SCM professionals can quickly pinpoint potential problems and adjust course if necessary.
SAP predictive analytics uses
Certain SCM areas can particularly benefit from predictive analytics.
Here are some ways SAP software's predictive analytics can help users improve their SCM:
- Demand forecasting. Machine learning applies algorithms to identify correlations and trends as well as anomalies that would otherwise distort the data. Demand forecasting can include factors like a product's current selling price, weather conditions and marketing budget.
- Inventory optimization. Algorithms determine the right on-hand stock levels, which minimizes excess inventory while potentially avoiding shortages.
- Risk mitigation. Predictive analytics can help identify potential supply chain disruptions and alert managers about potential weather-related bottlenecks and labor stoppages, among other factors.
- Route planning. Predictive analytics can help determine the fastest delivery routes and account for distance from various supply sources, traffic and weather.
- Dynamic pricing. With dynamic pricing, managers can adjust for market conditions and revenue optimization, balancing demand with the available product supply.