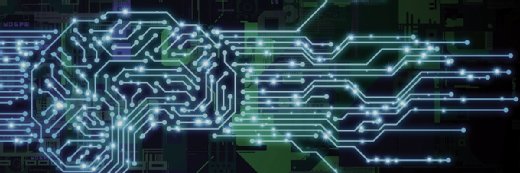
Getty Images/iStockphoto
The business case for AI-driven network orchestration
Organizations that are considering AI-driven network orchestration will find it has many business cases -- chief among them are reduced Opex and efficient resource use.
Now that many networking vendors have included AI functionality in their products, many organizations have implemented AI within their network infrastructures. Most of the remaining few that haven't are considering it.
This is the case at the University of California San Diego, the southernmost University of California campus. Established in 1960, UC San Diego has approximately 43,000 students and 42,000 employees. Tech leaders at UC San Diego are considering the use of AI-driven network orchestration to reduce operational costs and use resources more efficiently.
AI-driven network orchestration differs from network automation in the way it makes sense of large, heterogeneous data. For example, there might be 10 steps involved in an OS upgrade. Traditional network automation relies on limited checks between steps before moving on. Organizations still must verify the accuracy of AI-powered findings and recommendations.
"We would have to carefully monitor AI orchestration for its accuracy and keep close tabs on its error rate," said Vince Kellen, CIO at UC San Diego. Despite the shift to continual testing and evaluation, Kellen said he expects AI-driven network orchestration to save time for network engineers.
What is AI-driven network orchestration?
Network orchestration is a process by which administrators configure a centralized controller to create and manage network and security services. It's an evolution of network automation that integrates traditional automation processes with intent-based networking, which typically includes AI.
AI-driven network orchestration relies on AI's speed and power, which enable organizations to quickly sort through mountains of data collected over time. Before AI, it was difficult and time-consuming for organizations to sort through this level of data.
Once AI sorts through a collection of data, it can generate usable information to help with the following:
- Improving operations.
- Strategizing new products and services.
- Measuring performance from company and customer perspectives.
- Analyzing network traffic at a given time and using informed, automated decisions to adjust routing.
These capabilities reduce network outages and disruptions, which, in turn, improves customer satisfaction.
Reasons to adopt AI-driven network orchestration
Jonathan Forest, VP analyst at Gartner, cited the UC San Diego example -- the desire to reduce costs and better use resources -- as why many organizations should consider AI-driven network orchestration.
The decision to adopt AI-driven network orchestration depends on the business case, Forest said. According to Forest, factors that organizations should evaluate as they decide to adopt AI include the following:
- Potential time and cost savings.
- Improved UX.
- Reduced trouble tickets.
- Ability to address the skills gap.
- More efficient use of resources.
- Trust in AI outputs.
If organizations find they can realize these benefits with the adoption of AI-driven network orchestration, it might be worth it to implement the technology within their infrastructures.
"The goal is to operate more efficiently across the network operations lifecycle," Forest said. "This is the type of evaluation that organizations need to do to determine [AI's] worth."
Advantages and challenges of AI-driven network orchestration
Forest and Kellen both agreed that AI has several advantages and challenges. Organizations should thoroughly evaluate the pros and cons of AI-driven network orchestration to decide if it's worth the investment.
Advantages of AI-driven network orchestration
Advantages of AI-driven network orchestration include the following:
- Intelligent decision-making. AI-driven systems sense real-time environmental changes and optimize network performance accordingly across multiple services and domains.
- Complex, cross-domain environment management. AI enables analysis and decision-making in complicated cross-domain environments that might be too complex for programmatic network automation to manage.
- Improved predictive capabilities. AI anticipates and prepares for future events, enabling proactive network management. This helps prevent network failures before they occur.
- Enhanced optimization. AI-driven optimization enables network teams to balance competing goals, such as throughput, latency, cost and energy efficiency.
Challenges of AI-driven network orchestration
Challenges and tradeoffs of AI-driven network orchestration include the following:
- Requires investment in data infrastructure and machine learning. Organizations must invest in reliable data collection, storage and management systems across their network infrastructure.
- Requires retraining of staff and organizational changes. AI adoption in networking requires a workforce skilled in both networking and AI. This necessitates a culture of continuous learning and innovation.
- Raises explainability and trust concerns. Transparency in an AI algorithm's decision-making capabilities is crucial for trust and evolution from an advising copilot to a trusted automation system.
- Leads to potential data privacy and security concerns. AI systems require access to sensitive network data, so it's crucial to have reliable security measures and data privacy controls, especially with external AI services.
Top criteria for making the move
As UC San Diego ponders the move to AI-driven network orchestration, Kellen said the university uses some key criteria to help drive that decision. The university found it could reap the following benefits:
- Demonstrable ROI that lowers infrastructure costs.
- Reduced time to execute orchestrations.
- Improved response and agility to deploy network configurations.
"[Assuming AI meets these criteria], we feel that AI will quickly become the dominant form of application development in all layers of technology, including the network," Kellen said. "With any big shift like this, we expect there will be risks but more rewards over time."
UC San Diego uses these factors to evaluate AI-driven network orchestration, but other organizations should develop their own criteria to make the decision. This requires them to evaluate their use cases and weigh the potential risks and rewards of adoption. If the evaluation shows AI as worth the investment, businesses can move forward with AI-driven network orchestration to streamline network management.
David Weldon is a freelance writer in the Boston area who covers topics related to IT, data management, infosec, healthcare tech and workforce management.