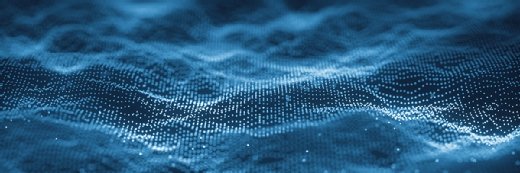
Nabugu - stock.adobe.com
AI skills for network professionals
Networking professionals must develop basic networking skills and AI to thrive in a complex landscape. Learn how AI's integration into network management will change the field.
It's essential for every networking professional, whether a novice fresh out of boot camp or a seasoned expert with decades of experience, to have a thorough understanding of basic networking skills. This remains true even as network management becomes more intricate and complex. In light of this complexity, organizations have considered using AI to manage networks.
The predicted AI boom could also spur a potential paradigm shift in networking, one in which basic network management intertwines with AI capabilities. AI could become a major imperative for network operations. In addition to basic network management skills, a solid understanding of AI will be a prerequisite for all current and prospective network professionals in the future.
Presentations during ONUG's AI Networking Summit Fall 2024 conference in New York City largely focused on AI's influence in network operations and management. In one panel discussion, experts discussed essential AI skills network professionals must hone to use AI within their network infrastructures.
How network pros use AI
Despite mounting fears and trepidation over AI, the technology isn't new to networking. Networking professionals have been implementing and enabling AI for IT operations (AIOps) to pinpoint and remediate issues within their infrastructures for nearly a decade.
Sherif Meshriky, principal network architect at The Cigna Group, described AIOps as the first phase of AI in networking. The next step, he said, is to use AI to consolidate automation scripts into a single comprehensive network infrastructure automation tool.
Many network professionals might dislike this second phase, fearing automation could replace them. But rather than replace network professionals, automation helps them be more productive, said Subbiah Muthukumaraswamy, head of cloud governance and infrastructure technology strategy at Memorial Sloan Kettering Cancer Center.
Meshriky agreed with Muthukumaraswamy and said he sees AI as more as augmented intelligence rather than artificial intelligence. "It's hand in hand with human intelligence -- its ability to enhance their capabilities, perform better and make more informative decisions," he said.
Data retention is another use case for AI, Muthukumaraswamy said. Some organizations -- such as those in the healthcare industry -- have large amounts of data from a variety of sources. AI can help professionals understand, organize and coordinate data through a taxonomy. Once an organization has a data model in place, it can figure out how to consume the data.
According to Susie Wee, co-founder and CEO of DevAI, organizations can also use AI in customer-facing businesses. Some organizations have built AI in their products; others use it to improve operations and extract intelligence from an organization's data. With all these various use cases, many organizations have formed task forces to figure out their AI strategy, Wee said.
"Bringing it all the way back down to operations, it's really [about] how can [AI] help people do operations better," she said.
Senad Palislamovic, head of technology at Nokia Enterprise, added that AI could enrich network professionals. But, to quell fears about AI, Palislamovic said organizations should educate network professionals on AI and machine learning (ML). Network professionals should understand various applications of AI, such as statistical analytics and prediction tools, GenAI, large language models and more.
AI skills for network engineers
For enterprises to enable these use cases within their networks, the professionals who manage the architectures must have a developed set of AI skills, including the following:
- Understand AI and ML. Because of the volume of AI tools available on the market, it's important for network professionals to have technical knowledge of AI systems. For example, if professionals decide to use an AI tool like ChatGPT to help with network management, they need to have a solid understanding of how it works, as well as the potential threats and business priorities of the tool. Additionally, network engineers can use prompt engineering, the process of training AI models to generate a specific response, to improve the performance of an LLM.
- Train AI systems. Network engineers should train AI systems to address the myriad use cases they could have in their network. For example, an AI system trained on the network can properly troubleshoot an error in the configuration. Troubleshooting is one of many potential use cases network administrators can train their AI to understand.
- Data management. In addition to understanding systems, network professionals must also understand the data that provides insight into the AI system. Network professionals must recognize where data originates and structure it properly for use cases. A data repository lets network professionals program AI tools successfully and build digital twins to monitor network infrastructures.
- Cross-team collaboration. As part of data management, network professionals must collaborate with members of other teams across the organization with their own datasets. This enables them to assimilate the data together to form a unified data structure to implement into their AI models.
- Coding. Coding might seem unrelated to network operations, but it can be essential to network professionals. When network engineers are proficient at coding, they can automate manual repetitive tasks. This can improve efficiency and streamline operations as network professionals can complete day-to-day tasks quicker.
The new network professional
Networking and AI might seem like disparate technology fields, but the two will continue to merge as AI permeates through every facet of IT. AI won't just be supplemental, but fundamental, to network operations. Furthermore, the role of a network professional has also changed in response to the new requirements of modern enterprise networks.
"A network engineer isn't [just] a network engineer," Palislamovic said. "They're really a computer scientist who understands vertical and horizontal [technology stacks] across the board."
Network professionals don't just need a solid understanding of networking; they now need knowledge in various subjects outside the field. For example, Meshriky said he recommends that prospective network engineer students study statistics to develop a solid grasp of AI data modeling.
It's not just the responsibility of network professionals to gain an understanding of AI. The panelists also encouraged enterprises to provide network professionals with the education, training and support to learn about AI and ease any concerns about the technology.
Furthermore, AI provides additional benefits beyond improved efficiency. Not only could gaining AI skills empower network professionals in their roles, but they also provide an opportunity for network professionals to upskill themselves in a competitive job market.
Deanna Darah is site editor for TechTarget's Networking site. She began editing and writing at TechTarget after graduating from the University of Massachusetts Lowell in 2021.