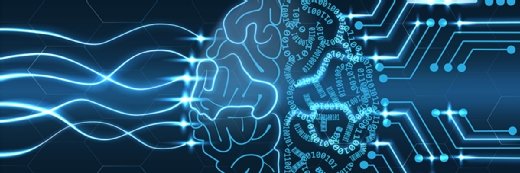
Laurent - stock.adobe.com
The benefits and challenges of AI network monitoring
The benefits include simplified network monitoring and automation capabilities. The challenges include data quality questions and integration complexities.
Artificial intelligence as part of network infrastructure monitoring has been a popular topic for several years. But only recently has the development of AI network monitoring made it practical to deploy in production networks on a broader scale.
With AI network monitoring, the main objectives are to sustain optimal service levels, gain accurate insight into potential infrastructure issues and get that data before business and network operations are affected.
To help with this process, machine learning -- a type of AI -- applies algorithms to telemetry and other data streams to gauge a baseline for normal operations. Once the AI network monitoring service establishes that baseline, it can then look for deviations that might indicate a potential infrastructure problem. Conceptually, AI monitoring can expedite troubleshooting and minimize costly network downtime.
AI monitoring is becoming a key element in AIOps, the marriage of AI and IT operations, in which machine learning is applied to big data to troubleshoot problems and automate formerly manual processes. AIOps encompasses a spectrum of network management, including event correlation, anomaly detection and root cause analysis.
Tapping into AI monitoring -- and, more broadly, AIOps -- to support network operations can be particularly important as organizations deploy more connected devices across their environments. Tracking both traditional IT infrastructure and industrial devices in a consolidated fashion should increase operational efficiencies and potentially reduce costs.
The benefits of AI network monitoring
Artificial intelligence has progressed as a key element in non-IT management use cases, such as autonomous driving and natural language processing for smart speakers. Now, trust and interest in AI to support IT operations are increasing.
AI network monitoring offers several potential advantages that create a more finely tuned, reliable and high-performing enterprise environment. The benefits of AI network monitoring include the following:
- Improved and simplified IT monitoring. Networks continue to evolve in terms of density of connected components and gear and in their virtualized nature. Monitoring these environments has become an involved and often inefficient process. Identifying issues before operations are negatively affected can be a steep challenge. Often, IT looks across disparate monitoring tools to isolate the sources of service-level degradation. AI network monitoring, however, promises to eliminate the fog associated with tracking network activity by providing a clear view on all elements in the infrastructure as they interoperate, streamlining IT operations. AI monitoring can also expedite and improve root cause analysis to get to the source of a problem quickly.
- Security insights. AI monitoring can also shed light on potential security issues by rapidly and accurately identifying threats and driving faster remediation. This also reduces instances of false positive alerts that flood security analysts and helps organizations improve their overall security postures.
- Driving automation. AI can inform the systems that can automate what were previously manual processes. While some of these processes may be iterative ongoing maintenance support elements, some organizations are exploring the use of automation for higher-level tasks. For example, AI can be used to trigger automated mitigation and remediation responses.
The challenges of AI network monitoring
As advantageous as AI network monitoring can be in a production environment, some challenges could impede a successful implementation. Fundamentally, IT operations teams need to overcome a learning curve to use AI monitoring effectively. In addition, at the outset of an AI network monitoring deployment, network operations teams should also consider the following challenges:
- Data quality. When discerning between harmless anomalies and actual network problems, AI is only as effective as the data it sources. If some real-time data isn't captured or there's an issue with data integrity, AI network monitoring can be inaccurate. Many organizations use synthetic data, which is created to simulate activity on a production network, as an alternative to real-time data feeds. Although synthetic data is similar to real network activity, it's not identical and may miss an actual issue or flag a problem that isn't one.
- Integration. As is the case when any new technology is deployed into a production environment with existing monitoring and management tools, integration can be tricky. If the IT organization cannot sync its AI network monitoring tools with other management and security services, then the output may be subpar.
- Ethical issues. As AI tools get smarter, some concerning ethical issues could emerge in terms of misuse and operational integrity. This is no different in network monitoring where AI technology analyzes vast amounts of data. Governments are trying to regulate how AI uses data, but it's a difficult and evolving task.