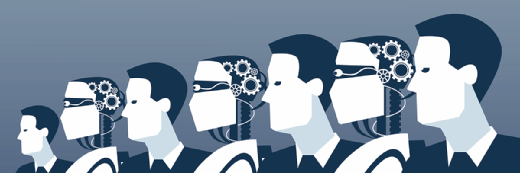
Prepare to train AI models for IT Ops non-stop
Algorithms have the potential to revolutionize IT organizations with carefully deployed models. Explore the learning approaches needed to successfully implement and maneuver these AI deployments.
More efficient IT, increased productivity and lower operational costs are some of the key gains of AI-fueled IT operations, or, more succinctly, AIOps. Technology innovations are making AIOps more accessible and affordable for organizations of all sizes. These improvements extend to wider use of GPUs, machine learning and data analytics.
IT leaders who take steps to adopt AI technology are on the path to highly automated, secure, self-healing data centers that require minimal hands-on involvement. However, this path also requires concerted effort to understand how to apply AI models to the correct use case, scale these efforts as needed and retrain the AI over time to ensure efficient performance. Let's explore current AI deployments, operational use cases and key elements in the training process to take AI to the next level.
AIOps in the data center: Goals and benefits
To achieve operational goals with AI depends on the aggregation of information from diverse IT sources, including systems monitoring data, performance benchmarks and job logs.
AIOps supports primary IT operations through the analysis of data points gathered from across an IT infrastructure. Alongside the combination of big data with machine learning to automate data center processes, an AIOps platform typically includes event correlation, anomaly detection and causality factors to improve equipment health, boost security and prevent downtimes.
The goal is smart automation that will proactively make improvements and automatically repair data center issues. Administrators can then rely on these insights to ensure that an infrastructure functions normally and responds quickly to alerts and possible system failures. For example, in addition to maintaining configurations and avoiding drift, IT teams can use AI to closely monitor hardware performance, extend usability or detect capacity losses and avoid service outages.
Beyond monitoring hardware, the most prevalent use case for AI in the data center is power management and more efficient energy use. AI deployments can optimize temperature controls, reduce electricity usage, improve sustainability and prevent costly power outages. IT teams can combine AI with predictive analysis to track distribution levels and identify potential defects in electrical systems -- benefits beyond good facility design.
Increasingly over time, IT leaders will adopt AI to optimize software deployments through workload automation and to ensure the best application performance. For example, a well-trained AI model could automatically balance cost and risk factors to help an organization decide whether to place workloads on premises or in the cloud.
Key considerations for training AI
Understanding AI models is fundamental to implement an effective training process. Models in AI are the outcomes of algorithms, which are computational instructions that deliver a desired response. Different data fed into the algorithm will deliver a different model. By processing pools of information and learning to detect patterns, a well-trained model within a data center can execute the same actions that an IT expert would.
It's difficult to understate the importance of data quality to effective training. AI requires massive amounts of information to achieve desired outcomes. And the training process typically includes steps to identify, aggregate, clean and annotate a known data set, as well as integrate data points from different silos. The goal is to sort out inconsistencies in advance to ensure the training feeds are rich and accurate.
IT teams dedicate a substantial amount of time to cleanse data and engineer features to accurately represent the signals within a data set. Once models are deployed, administrators can monitor them for drift and retrain as necessary. Careful upfront planning for AI deployments is critical for success and to understand future scalability requirements.
The three main approaches to training consist of supervised, unsupervised and reinforcement techniques. With supervised learning, IT personnel and data scientists supply the model and training data. Algorithms then become the vehicle for improving and fine-tuning the model and assimilating new data.
In unsupervised learning, AI algorithms independently identify patterns in unlabeled data and then take actions based on those insights, increasing accuracy through repetition and experience.
With reinforcement learning, it is crucial to provide feedback using rewards and penalties. Through constant feedback, models learn how to identify and produce optimal outcomes. These approaches incorporate precise, accurate information. Ultimately though, human knowledge of the data center is the key ingredient to train AI to make the right decisions and execute the appropriate actions.
AIOps and the future
Assessments for effective AI deployments should also address infrastructure. The goal is to have hardware and software that support flexible scale-out to accommodate AI-related growth. For example, networks should provide the low latencies and high bandwidth needed to meet new compute and data demands, and the fast message rates and smart offloads necessary to AI.
Multiple server GPUs are fundamental to read data at the high rates AI requires. Organizations can create a shared storage infrastructure to scale smoothly as AI use expands.
IT teams will also encounter a range of unique challenges around their AI deployments. Primary among these are communication challenges between the teams responsible for designing, building and operationalizing AI models. For example, engineering and IT teams sometimes struggle to understand and operationalize the AI models data scientists create.
For smaller organizations and startups, formulating ways to rapidly clean enormous amounts of data, perform feature engineering and train models efficiently can present key hurdles. However, it's clear that AI will become a dominant data center force for improved operations as the technology goes mainstream and adoptions grow.
The cloud will boost much of this expansion. An increasing number of AI cloud services offer data center options to host AI hardware or keep network AI models current with new data elements and patterns.
According to research from Gartner, the use of AIOps to monitor applications and infrastructure will rise from 5% in 2018 to 30% in 2023. In addition to boosting their data center efficiency, more organizations will rely on AI to keep up with other emerging technology trends. A hybrid approach to AIOps might help IT leaders move beyond AI deployments for basic resource monitoring, as well as maintaining hardware configurations and resiliency to more complex tasks.