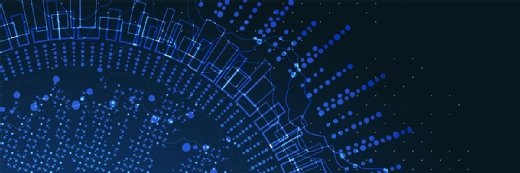
KOHb - Getty Images
How AI can automate IT patch management
AI-based patch automation can take pressure off the process of scanning, testing, deploying and producing a report. Is AI the right way to go for your organization?
Organizations can use AI tools to take advantage of the numerous benefits that automated patch management offers, but it comes with some limitations.
Patch management uses software devices and procedures to update digital assets such as servers, networking devices or any other digital appliance that run an OS. The goal of patch management is to secure computing devices against cyberthreats and ensure they use the latest drivers, software versions and firmware to run smoothly.
Using automated tools for patch management reduces IT support time, makes the attack surface smaller and helps organizations meet compliance requirements. Automated patch tools initiate their tasks automatically based on events, triggers or schedules that admins set to run at predetermined times. A typical manual patch management process involves the following phases:
- Scan all computing devices across an organization's IT environment to identify missing patches, outdated applications, firmware and drivers.
- Visit each vendor's website to download and install or update the required patch.
- Test critical patches, such as those installed on key servers, before applying them to all other devices so they don't stop any feature or function.
- Manually install patches on all devices.
Automated patch management lets admins execute the previous steps without human intervention. It involves the following phases:
- Scan connected devices across an organization's IT environment to identify which devices and applications need security patches or updates.
- Automatically connect to each vendor's website to download patches. For example, it can connect to the Cisco portal to patch a Cisco router or firewall.
- Test the patch on some devices before applying it to other devices. This is helpful for situations such as applying patches to critical systems that can't go offline.
- Produce a detailed report about the patching activities along with their dates, versions and affected systems. These reports are valuable because they act as proof of the business's technical competency and adherence to the best technical standards in protecting users' sensitive data. Later, these reports can be sent to compliance bodies.
How does AI-driven patch management facilitate patch management?
AI-powered patch management tools can significantly improve the patching process and make it more intelligent and context aware by performing the following tasks:
Automated patch identification
AI can automatically scan connected devices across an organization network and identify relevant vulnerabilities in real time. AI continually monitors patched systems and identifies any anomalies or suspicious behavior. AI then applies remediation actions such as employing additional patches or notifying IT admins to take further actions.
Patch prioritization
AI-powered patching tools can prioritize required patches based on factors such as the severity of the vulnerability and its impact on core business functions. This lets admins focus on the most critical vulnerabilities that can significantly affect the organization's IT infrastructure and data.
Better patch compatibility analysis
AI-powered patching tools use machine learning (ML) models to learn from past patching activities. This improves their ability to identify compatibility issues that might arise when patching systems, allowing them to act promptly and roll back patches or revert systems to their original state. This enhances patching efficiency and saves the time needed to test patches before deployment.
Limitations of AI-powered tools in patch management
Despite the numerous benefits of AI-powered patch management, organizations must consider the following limitations before using the technology:
- Compatibility issues. When admins apply patching automatically to complicated environments, it could lead to unexpected issues or system instability. For example, an organization's IT environment might contain a mix of Windows 10 and XP machines. An AI tool would struggle to automatically patch both systems because each one belongs to a different generation of OS.
- Lack of context awareness. Patching isn't merely dependent on understanding the technical issues of the target IT environment. Admins must consider other factors when they apply patches, such as regulatory compliance and business priorities. Human insight and guidelines are still required during the patch lifecycle.
- Training data quality. The effectiveness of AI-powered patching tools depends on the ML models on which they are trained. For instance, if the underlying training datasets are incomplete, poisoned or have low quality, the patch tool will provide inaccurate recommendations that can severely affect an organization's security posture.
- Vendor lock-in. Depending on a specific AI vendor to provide all of your organization's patch management services could result in a vendor lock-in situation. This makes shifting to another patch tool complex and costly.
- Cost. Many SMBs can't afford the costs to maintain an AI-based patch automation tool. This can lead to them outsourcing this function, and possibly falling victim to vendor lock-in issues.
Nihad A. Hassan is an independent cybersecurity consultant, an expert in digital forensics and cyber open source intelligence, and a blogger and book author. Hassan has been actively researching various areas of information security for more than 15 years and has developed numerous cybersecurity education courses and technical guides.