Launching an AI project calls for careful scoping
AI implementation may benefit from a handful of steps and a common set of patterns, according to speakers at the AI World Government conference in Washington, D.C.
As more customers think about launching an AI project, more channel partners will find themselves nudged into an advisory role.
The good news is that approaches are emerging to help get AI deployments off the ground. Industry executives presenting at the AI World Government conference, held this week in Washington, D.C., outlined AI implementation steps and implementation patterns.
Arit Garg, emerging market and technology director at Cray Inc., a supercomputer manufacturer based in Seattle, said organizations looking to adopt AI may encounter an "overload of infrastructure" options. While AI implementation may appear to demand a long, winding trajectory, Garg suggested enterprises "think of AI as having a much simpler roadmap."
Steps for launching an AI project
Garg, who spoke during an AI World Government session on the AI vendor ecosystem, broke down the task of launching an AI project into three steps:
1. Defining the problem
Ninety percent of AI efforts fail and the lack of a problem statement is the main culprit, Garg noted. The key to success is having a clear, high-value problem you are trying to solve. During this step, an organization may find that the problem, as defined, is not a good fit for AI or that it needs to pursue a steppingstone project before it can tackle AI.
2. Defining data requirements
Data fuels AI deployments, but organizations may struggle with data management. "Once you do know what you are solving, think about the data you have … and the data that you need to solve the problem," Garg said.
3. Selecting vendors
Garg said the vendor landscape -- market research firm Cognilytica has classified nearly 3,000 AI vendors -- becomes much easier to navigate when organizations first define the problem they aim to address and the data they need to solve it. With that insight, organizations can narrow the list of vendors capable of addressing their requirements. "Most will fall away," Garg said.
Start small, find the right pattern
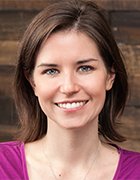
Kathleen Walch, managing partner and principal analyst at Cognilytica, based in Washington, D.C., recommended organizations take a limited first step into AI, but maintain a wider vision. "Start small, think big, iterate often," she advised, during an AI World Government session on AI adoption. A small project that doesn't take too long to complete and generates ROI can get AI adoption rolling.
Identifying which pattern a given AI project follows can also facilitate implementation. Cognilytica has identified seven core AI patterns, based on its analysis of hundreds of use cases: conversation and human interaction; predictive analytics and decisions; goal-driven systems, autonomous systems; patterns and anomalies; hyper-personalization; and recognition. The seven patterns help determine how to best implement and manage the project, according to Cognilytica.
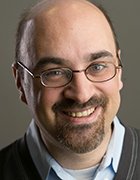
The patterns can also influence the order in which an organization takes on AI projects. Conversational AI systems, such as chatbots, can have great short-term ROI, noted Ronald Schmelzer, managing partner and principal analyst with Cognilytica.
On the other hand, the autonomous AI pattern may not prove a good starter project. Autonomous projects take the human out of the system and tend to have a higher degree of difficulty, Schmelzer noted.
"This is a hard problem," he said. "We don't recommend starting with an autonomous system."