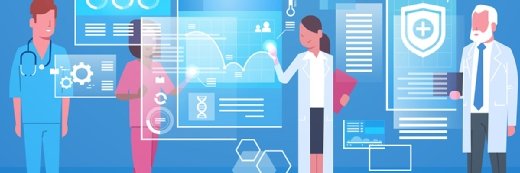
gmast3r/istock via Getty Images
How Epic is finding the “sweet spot” for EHR AI integration
As digital health transformation progresses, thoughtfully implementing EHR AI tools will require balancing efficiency, provider trust and scalable adoption.
While most physicians agree the EHR helps them deliver high-quality care, the digital system is cited as a top contributor to clinician burnout due to growing patient message volumes, increasing clinical documentation requirements and poor usability. As the AI buzz continues across the healthcare industry, organizations are looking to EHR AI integration to streamline workflows.
In this episode of Healthcare Strategies: Industry Perspectives, Sean McGunigal, director of artificial intelligence at Epic, shares insights on the EHR vendor's approach to responsible AI implementation. He also describes key use cases demonstrating value and how healthcare organizations can navigate AI adoption at scale.
Recorded at HIMSS25, the conversation explores the evolving role of AI in clinical documentation and what’s next for AI-powered EHR innovation.
TRANSCRIPT TEXT STARTS HERE.
Sean McGunigal: I am certainly a big proponent of AI, but I understand where AI can fall short, and it really, for us at Epic, comes down to being very thoughtful, not just with where we integrate AI, but how we integrate AI.
Kelsey Waddill: Hello, this is Healthcare Strategies: Industry Perspectives coming to you from HIMSS 2025 in Las Vegas. And I'm Kelsey Waddill, a podcast producer at Informa TechTarget. EHRs are one of the biggest pain points in every provider's day-to-day. Ask any of them. They will tell you this. Documentation takes up a tremendous amount of time and energy. Combing through the document to find answers, also time-consuming.
But hey, I'm at HIMSS25. Got to ask the question, can AI do something about this? Lucky for us, Sean McGunigal, director of artificial intelligence at Epic, took the time to meet up with me at HIMSS25 to share a little bit about how AI can make this unwieldy task a bit more manageable and where AI integration into EHRs is most powerful, or shall we say epic. Okay. Wait, wait, wait. I'm sorry. Don't go. I promise that was the only pun. Anyway, here's Sean.
All right. Well, Sean, thank you so much for coming on to Healthcare Strategies here at HIMSS25. Excited to get into the conversation today.
McGunigal: Yeah, thank you for having me.
Waddill: Great. First of all, before we dive into the nitty-gritty here, could you just share with our audience just your name and what you do?
McGunigal: Sure. So Sean McGunigal. I'm a software developer at Epic on our cognitive computing platform team, which is our AI infrastructure team. So I specialize and focus on artificial intelligence, machine learning and now generative AI. And I work with our application teams really across Epic software to find ways to embed those AI tools to help the users of our software, so to help providers, to help patients, to help health systems in general try to find every possible opportunity.
Waddill: Excellent. And how have you been experiencing HIMSS? How's your HIMSS been so far?
McGunigal: HIMSS has been great. It's-
Waddill: AI this year.
McGunigal: A lot of AI as we expected, so this is not my first HIMSS, thankfully. And it's great to see a lot of old familiar faces coming by our booth. We've had a lot of great conversations with a lot of health systems, both current Epic customers and not current Epic customers, and it's just really exciting to see all the buzz around AI again this year.
Waddill: Yeah, great. So get us started here. Let's hear a little bit about, we're surrounded by so much AI talk here at HIMSS, and I'd love to hear what you think the sort of sweet spot is for AI and EHR integration right now.
McGunigal: Yeah, so right now I would say the sweet spot is leveraging AI, particularly for what I would consider the easy tasks in the health system. So administrative burden, operational efficiency, those are the core areas that we're focusing on, and we think that those are really good opportunities to help improve the lives of providers in the health system, help improve some of the operations of the health system in a way that isn't relying on maybe the kind of clinical knowledge that some of the clinical use cases might have. It's not to say that the clinical use cases aren't there. It's just, especially for organizations that might be a little hesitant, starting with some of the operational or efficiency workflows, this tends to be a good starting point, a good way to get into the space and start to see value right out of the gates.
Waddill: Yeah, that's important for organizations to be able to see value right out of the gate if they're starting out this way because it can get very discouraging to go the other way around.
McGunigal: You don't want to spend a ton of time and see no value ultimately. Yeah.
Waddill: Absolutely. So when we talk about digital innovation, we talk about kind of how do you determine that value? How do you measure the success of these tools? When we look at AI integration into EHRs, and I'm sure this is different based on the use case, but how do the metrics differ from other digital health innovations or how are they the same?
McGunigal: Sure. Yeah, so I think particularly with generative AI, we've seen them differ, I would say pretty substantially, from the AI of the past where, especially for clinical decision support, you're looking at a prediction and you're trying to evaluate the performance of that prediction. And it's very rigid, it's very mathematical and it's very rigid, but with a lot of the generative AI tools, you're looking at trying to help users in a way where even if it's not perfect, it still helps folks. And so I think of an example, like one of the tools that we've had in our software for over a year now, which is called In-Basket ART. It's one of the patient messaging tools that a provider has to help suggest a response to a message that a patient sent a provider. And the tool has come a long way over the last year since its initial release, but one of the things that we had seen even in the early days was providers were finding value even if they had to rewrite the message.
And so to quantify that, to really quantify how much value or how useful is this tool, it's less about the kind of statistical performance and you're looking more at key performance indicators for the workflow.
So what you said, it does come down to the use case and the workflow. And for something like that In-Basket tool, we look at time saved. So we look at how long the provider is spending reviewing the chart, how long the provider is spending sending the message, and those are really indicative for us for how well this tool is performing. Sure, it helps that the providers don't have to make a lot of edits, and so getting a really high-quality response is really, really valuable. But the ultimate metric or measure of performance is going to be those times saved. And you'll see that really across the board with all the generative AI tools that we're building into our software, is you have both operational monitoring metrics that see how are the users doing as they're interacting with the tool as well as measures of more of the statistical side, so measures of the actual integration performance.
Waddill: So as we address different organizations that are trying to integrate (AI) into their EHR, we obviously have some, especially larger organizations that are trying to move this route. And they often, when it comes to AI, generally speaking, get stuck in a pilot fatigue phase where they are able to tackle on the smaller level, but then scalability becomes an issue. Is that something that you see in EHR integration as a potential challenge? Is that not really an issue for EHR integration? What do you think about scalability in this space?
McGunigal: Yeah, so you're talking to a platform engineer here, so when I think scalability, I immediately think scalability of the infrastructure, which was a limitation in the early days. There was simply too much demand for these generative AI tools than there was GPUs to run them. And so there was a scalability limitation of actually having the compute to run these at an enterprise scale. Thankfully, that's no longer true. So thankfully now we have the technical capabilities to scale out. We are still seeing what you were describing where there are organizations that are getting stuck in that pilot phase.
Generally, the guidance we would provide in that context is make sure you're going into the pilot with very clear measures of success. You need to know what you are measuring and what you're looking for out of a pilot so that you're not having a moving goalpost situation. You don't want to evaluate a tool, find that it performs well, some providers like it, some providers don't like it, and then you don't know what to do with that information. You don't know where to go from there. So generally what we would say is make sure you're approaching those pilots very methodically and that you have those very clear goalposts in mind so that you can say, "Yes, this looks good, let's roll it out," or "No, this doesn't look good, and let's wait until the tool is more mature," for example.
Waddill: That's great news that the infrastructure is no longer the barrier.
McGunigal: Yes.
Waddill: It's more the process, it sounds like, that we have to work through to ensure that works out well. Great. So I'm curious, even as AI is not new in healthcare, it's not new in general, but even in the last couple of years as the industry has been trying to see the possibilities of it and the capabilities of it, there are a lot of providers who are understandably a little bit nervous about the idea of potentially too much reliance on AI and potentially different AI tools replacing clinical judgment and the risks associated there. So I was just curious, as someone who sees the other side of these tools, what would you say to doctors who might have that kind of a reservation?
McGunigal: Yeah, I would say the concerns are certainly valid and concerns that we share, as well as I would say I'm certainly a big proponent of AI, but I understand where AI can fall short. And it really, for us at Epic, comes down to being very thoughtful, not just with where we integrate AI, but how we integrate AI. So we don't want to be replacing clinical judgment. Especially in the current state, you are going to find that these models are not perfect, they are not going to be able to do everything a provider is doing today. And so you need to integrate them in a way that they're assistive for a user and not replacing.
So I think of something like a summarization where the summary is just trying to tease out some of the key information in the chart, but for every line item in that summary, I need to be able to show a citation to that user so they can go in, they can read more information, they can confirm that the summary has the full picture, has the full context. And so we need to be really thoughtful with how we actually integrate a lot of these solutions into the software that our providers are using.
I would also say be a critic. Being a critic or being a skeptic also encourages that validation and monitoring around these AI tools that they really do need. You do need to have somebody making sure that they're working for your particular patients, for your particular health system. We at Epic try to help organizations be skeptical of these tools. And one of the ways that we try to help do that is with a suite of software that we've released last year called the Trust and Assurance Suite. And what that aims to do is basically arm each health system with the data science tooling in order to verify, ‘Yes, these AI tools are performing as we would expect on our populations with our providers.’ And it's something that these organizations can use ongoing. It can become a monitoring tool, and it's one that we would encourage as organizations are really starting to mature in terms of AI governance, they start to establish these toolkits where they can and should evaluate all of the AI tools in their system.
Waddill: I'm curious if there's any kind of sneak peek that you could give us into the stuff that Epic is excited about or working on. What are you working on right now?
McGunigal: So one of the things that we're pretty excited about, and we have been talking at the booth at HIMSS here, is some of the work we've been doing in building agents, and I think agents are a tough term to describe. But in effect, these are tools where I don't have to give to a generative or an AI tool the exact step-by-step of what I want it to do. Instead, I can give it a broad goal and I can give it access to some of the data in the chart, for example, and I can give it access to some actions to take. And I allow the agent to go through its workflow as it would reason, basically.
So one of the really key powerful tools that comes with this agent is it can do more than a single step. We can go from a place where we're not just suggesting a response to a patient's message. We can actually tee up a follow-up order if we think ... The patient's asking for a refill, great, let's tee that up. If we think the provider might want to schedule a follow-up based on what the patient's sending, let's tee that up.
And so by having the behind the scenes agent where it's not just doing one thing, it's reasoning for almost as if it was the provider, by the time the provider actually looks at the message, we've already done a lot of the leg work that we would expect the provider to do. Of course, the provider can say, "Nope, I want to take it a different route," in which case, great, you can. But for many examples, we think that's going to help simplify a lot of the work in the system. And so we're pretty excited. I think that's one example of an agent, but we've been working on quite a few agents over the last year and finding different areas where they do well in the healthcare software that we're building.
Waddill: Awesome. Is there anything that we didn't touch on that you think is really important to touch on in this space of AI integration into EHRs or anything that we did touch on that you want to dive in a little bit deeper on before we go off?
McGunigal: No, I would just say that the more you know the better when it comes to AI. So if you're not using AI, I would say check it out, try to use it, use ChatGPT, familiarize yourself with what these tools do, and it'll demystify a lot of the fears around AI. The more we know, the better we can actually integrate these into the software. So definitely recommend everyone's trying to be as AI literate as possible. And especially if you're a health system today, definitely be thinking about governance, AI governance. It is going to become prerequisite for a lot of what we see as the future of healthcare delivery.
Waddill: Excellent.
McGunigal: Yeah.
Waddill: Yeah. Well, thank you and I hope you have a great rest of your time at HIMSS.
McGunigal: Thank you.
Waddill: Listeners, thank you for joining us on Healthcare Strategies: Industry Perspectives. When you get a chance, subscribe to our channels on Spotify and Apple and leave us a review to let us know what you think of this series. More industry perspectives are on the way, so stay tuned.
This is an Informa TechTarget production.
Hannah Nelson has been covering news related to health information technology and health data interoperability since 2020.