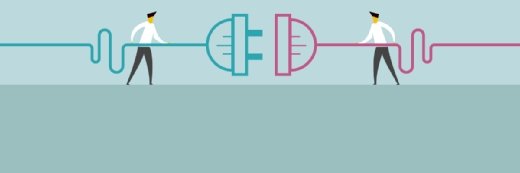
Developing a COVID-19 Mortality Risk Tool for EHR Integration
Researchers leveraged numerous models in one month to create a mortality risk tool prepared for EHR integration.
Health IT professionals developed a COVID-19 mortality tool for EHR integration within a month, according to a recent study published in the Journal of the American Medical Informatics Association (JAMIA).
Researchers created an accurate, real-time, in-hospital COVID-19 mortality risk solution that health IT professionals could optimize based on emerging knowledge about the pandemic.
The quick spread of COVID-19 created a need for an accurate and context-sensitive EHR tool to predict hospitalized patient mortality for patients with and without the illness. But making these tools usually takes years, leaving the developers to turn to other scoring systems used for other, similar illnesses, he study authors wrote.
Researchers developed the mortality score by stacking SOFA, a commonly utilized pneumonia mortality score, and an acute respiratory distress syndrome (ARDS) mortality model, and a comorbidity score. The researchers tested the model on 82,087 patients at University of Colorado Hospital (UCHealth) with and without COVID-19
The research team had four main goals. Those goals were to utilize SOFA as a Crisis of Standards of Care protocols baseline and adding other models with the potential to boost predictive performance and robustness, integrate and test potential COVID-19-specific predictors, launch the solution across a 12-hospital health system, and validate model performance.
Researchers developed, validated, and integrated the model in one month by leveraging EHR data estimates while also building a transition to a prospective data model. The solution generated a mortality risk once every 15 minutes for every inpatient across the 12-hospital health system.
Due to the ever-changing pandemic, the data pipeline could update once new data entered the EHR.
“Rapid development and implementation of a new score in a real-time EHR requires a full clinical and informatics pipeline including skilled data warehousing, data wrangling, machine learning, health system information technology (IT), and clinical and ethics personnel working in sync,” explained the researchers.
“All data flowed to the study team from UCHealth’s Epic instance through Health Data Compass (HDC), the enterprise data warehouse for the University of Colorado Anschutz Medical Campus. HDC is a multi-institutional data warehouse that links inpatient and outpatient electronic medical data, state-level all-payer claims data, and the Colorado Death Registry.”
The solution revealed 5 percent of the encounters were positive COVID-19 cases.An additional 16.5 percent of cases required intensive care, 5.4 percent required mechanical ventilation, and 2.5 percent, or 717 individuals, eventually died.
Patients with COVID-19 were more likely to be male (55.6 percent), be Hispanic (44.4 percent), receive ICU-level care (30 percent vs 15.8 percent), be intubated (17.6 percent vs 4.8 percent), have an increased duration of mechanical ventilation (8.7 days vs 3.0 days), a longer hospital stay (7.6 days to 4.3 days), and die (12.2 vs 2.1 percent). Patients with COVID-19 also had higher SOFA scores.
“Of note, there are many reports in the literature describing development of predictive models using EHR data, but very few reports of the implementation of those models in a live EHR for clinical use,” wrote the study authors.
“In this case, the total elapsed time from including data extraction, model construction, implementation, and deployment within the EHR across the 12 UCHealth hospitals was 1 month, illustrating the potential capacity for novel predictive model development. Now that we have demonstrated a workflow to rapidly develop new informatics tools in our health system, we anticipate that many other tools will follow.”
Researchers said the solution is an example of allocating scarce medical resources and leveraging numerous models to create a mortality score in a short amount of time.
“We rapidly deployed the novel score in our EHR during the COVID-19 pandemic for potential real-time use in making triage decisions,” concluded the study authors.
“We demonstrated that reliability was maintained in a prospective cohort of patients with and without COVID-19. Fortunately, we have not needed to use these scores for triage, but our development process forges a new path for leveraging EHRs, clinical expertise, and machine learning to provide real time, situation-critical clinical decision support”