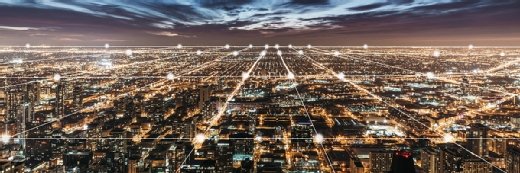
Getty Images/iStockphoto
Clinical Layout is Key to Patient Interaction, EHR Screen Gazing
Widespread EHR adoption has negatively impacted patient interaction, resulting in increased EHR screen gazing during appointments.
Health systems can integrate an automated EHR screen gaze and dialogue tool to track provider-patient interactions, according to a study published in JMIR Publications. Clinical layout is vital to provider-patient interactions and providers tend to screen gaze at a higher rate when the EHR is out of peripheral vision.
Provider-patient communication is critical to driving patient safety, satisfaction, and outcomes. However, according to numerous studies, communication is impacted by EHR adoption and usability. EHR prevalence can have a negative impact on eye contact, decrease active listening, and provide interruption during an appointment.
Researchers integrated an automated tool and utilized the computers’ camera and microphone to detect and classify screen gaze and provider-patient dialogue during medical appointments. Recent advancements in machine learning allowed researchers to evaluate pose and voice activity, researchers said.
In both semi-inclusive and fully inclusive layouts, the tool detected the clinician gazing at the EHR while having a patient conversation, the clinician conversing with the patient while looking away from the EHR, and the doctor gazing at the EHR without conversing with the patient. Any other form of dialogue or gazing was considered out of scope, the study authors said.
Researchers compared the tool’s performance to a separate human coder that could go through the video once. This evaluation aimed to simulate a real-world scenario of video coding assigned to an external coder, the study authors explained.
This research confirmed the impact of clinical layout on provider-patient-EHR interactions, the study authors noted. The fully inclusive videos contained 5 percent more dialogue and screen gaze interactions and less screen gaze plus dialogue interactions than semi-inclusive videos.
The fully inclusive layout made the clinician choose between computer or patient, while the semi-inclusive layout allowed the clinician to look at both the patient and computer screen.
Research revealed the automated tool was just as accurate as the human coder. The tool is unobtrusive and inexpensive compared to the human coder because it does not require additional setup and it only requires the computer’s internal camera and microphone. It also improves patient privacy and security because the video is locally processed.
Both the tool and the coder were more accurate in the fully inclusive layout, compared to the semi-inclusive layout because in the fully inclusive layout, the physician had to rotate her head 90 degrees away from the EHR to gaze at the patient. In the semi-inclusive layout, the head rotation is not as drastic, so it is easier for the tool and the coder to make a distinction between interactions in the fully inclusive layout, the study authors explained.
Both the tool and coder confused both screen gaze plus dialogue interactions with strictly dialogue interactions. Nearly 17 percent of the tool’s errors and 22 percent of the coder’s errors occurred near transitions between interactions.
However, the tool made a common mistake when detecting moments of silence between dialogue, whereas the human coder did not.
“This kind of information may be useful to detect conversational dimensions such as hesitant speech,” wrote the study authors. “However, for our purposes, this leads the classifier to overestimate the lack of verbal interaction. To counteract this, further rules are needed to identify which moments of silence are part of a conversation and which are not.”
Future studies should take into consideration about the language and conversation content, other activities that both the provider or patient are engaging in, and nonverbal behavior such as nodding, the study authors noted.
“Our evaluation showed that the classifier's performance was similar to that of a human coder when classifying 3 combinations of screen gaze and dialogue in doctor-patient-computer interactions,” the study authors concluded.