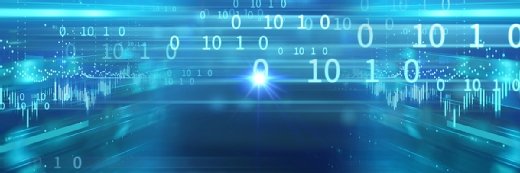
Getty Images/iStockphoto
Using Machine Learning to Help Characterize Clinician EHR Behavior, Workflows
The machine learning approach identified two distinct EHR information-gathering strategies, which could reflect individual variations in clinician EHR behavior.
Annotating EHR audit logs using machine learning could help researchers study clinician EHR behavior at scale, according to a study published in JAMIA.
Raw audit logs capture a trail of clinician activities within the EHR, allowing researchers to study physician behaviors at the microscale. However, raw audit logs lack context for clinical activities.
While manual annotation of audit logs by clinical experts to provide context is possible, the researchers said these efforts are labor-intensive, potentially error-prone, and not easily scalable.
Using a dataset of 15 million raw audit log actions performed by 88 intern physicians across inpatient and outpatient settings, the researchers developed a novel approach to annotate EHR activities using action embeddings, a scalable unsupervised machine learning approach.
“Our embeddings approach provides insights—complementary to clinician expert audit log annotations—into the context in which individual EHR actions occur,” the study authors wrote.
“By situating specific actions within the broader context of their cooccurring activities, our method provides opportunities to generate meaningful hypotheses regarding clinical work activities, clinical workflows, and user interactions with the EHR,” they added.
The researchers identified two distinct EHR information-gathering strategies used during chart biopsy activities, which could reflect individual-level variation in EHR use or setting-specific workflows.
“The identification of distinct workflows for the same purpose creates opportunities for studying workflow efficiency and its contribution to outcomes such as burnout,” the authors wrote.
The approach also found that activities like placing orders, writing notes, and inbox messages tended to occur across diverse contexts, potentially reflecting the disjointed nature of clinician work.
“Because our method treats audit log activities as tokens, it can be applied across any EHR raw audit log source, regardless of vendor or institution,” the study authors pointed out.
“Recent research has utilized raw audit logs to measure physician workload, workflow, teamwork, cognitive burden, and burnout, illustrating the considerable potential of raw audit log data to capture physician behaviors at the macroscale,” they continued.
The approach also enables the use of raw audit log data in machine learning research since it transforms audit log data into numerical vectors.
“Because clinicians spend the majority of their time at work using the EHR, raw audit log activities may reflect not only clinician workload but also behavioral characteristics such as distractibility, which have the potential to be used to predict physician behaviors, errors, and outcomes,” the researchers wrote.
“Traditional machine learning requires painstaking manual engineering of features to represent these complex concepts; in contrast, applying embeddings to raw audit log data enables the use of deep learning, which can directly learn these representations from the raw data,” they added.
The researchers pointed out that the study has several limitations.
“This was a single-center study on a small sample of intern physicians working across diverse clinical settings and specialties,” they said. “Although our work has a strong theoretical foundation from NLP, further research is needed to establish the applicability of embedding representations across other EHR vendor platforms, clinician types, and work settings.”
For instance, the researchers noted that cluster analysis to identify workflows might not be as effective when the clinician population is more homogenous.