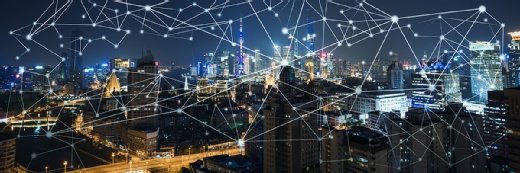
Getty Images/iStockphoto
Machine Learning Model Predicts Physician Departure With EHR Use Metrics
The machine learning model found that physician demographics, EHR use metrics, and patient panel characteristics contributed most to the risk of physician departure.
While reasons for physician departure are multifaceted, machine learning (ML) models that leverage EHR use metrics could aid in identifying those at risk of leaving, according to a study published in PLOS ONE.
Researchers conducted a 34-month longitudinal cohort study of 319 physicians from a large multispecialty ambulatory practice.
While healthcare organizations typically leverage surveys to track clinician burnout and job satisfaction, the study used EHR data, including EHR use time and clinical productivity measures. Researchers also collected data on physician characteristics, including age and length of employment.
The study used portions of the data to train, validate, and test the ML model. When tested, the model was able to predict whether a physician would depart with 97 percent accuracy, the researchers found.
The features with the greatest average contribution to departure risk in the study population came from physician demographics, EHR use metrics, and patient panel characteristics.
Researchers found that high EHR use time among clinicians with high tenures tended to increase departure risk. However, high EHR use rates among those with lower tenures were associated with lower risk.
The study revealed that this pattern is consistent for total EHR time, inbox time, and order time.
However, note time departs from this trend; increased time on EHR notes was associated with departure for the shortest tenure physicians.
“This indicates that both who and how the EHR is being used are important factors in determining risk of departure,” the authors wrote. “Future qualitative research could be aimed to understand how these differences arise across tenure groups and if high EHR use is a marker or causative feature for upcoming departure.”
“Taken together, these findings demonstrate that machine learning algorithms, which can identify complex interaction and non-linear effects can be used to predict physician departure and provide an interpretable summary of what factors contributed to calculated risk of departure for each physician,” the study authors wrote.
The researchers noted that a strength of the ML model is its ability to generate dynamic monthly risk predictions for each physician.
The features with the highest average change in risk contribution were teamwork on inbox, documentation time, panel count, and panel complexity.
“Although this analysis cannot attribute causality to features impacting predicted departure risk, both teamwork on inbox and documentation time are intervenable features for which targeted changes could be designed,” the authors suggested.
“For example, structures for inbox task distribution or the addition of documentation assistants like dictation tools or scribes could be implemented,” they added. “Panel count and complexity are both potential indicators that a practice is winding down, and although not intervenable, could provide early warning about an impending departure.”