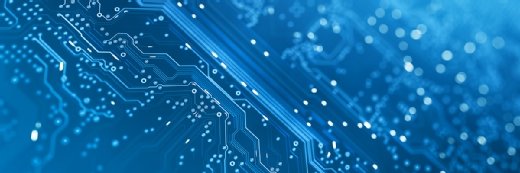
Getty Images/iStockphoto
Algorithm Harmonizes EHR Data into ‘Macrovisits’ for Clinical Research
Researchers said the algorithm is a foundational step in quantifying EHR data heterogeneity and harmonizing data for clinical research.
Encounters are a heterogeneous component of EHR data, limiting the full potential of leveraging real-world data for clinical research, according to a study published in JAMIA.
Researchers demonstrated the successful use of an algorithm, in addition to common data model (CDM) harmonization, to aggregate and classify EHR visits generated from varied, site-specific operational rules into “macrovisits.”
The study leveraged encounter data from 75 partner sites harmonized to a common data model (CDM) as part of the NIH Researching COVID to Enhance Recovery Initiative, a project of the National Covid Cohort Collaborative (N3C).
The study found that atomic inpatient encounters data were widely disparate between sites regarding length of stay (LOS) and the number of CDM measurements per encounter.
After aggregating encounters to macrovisits, LOS and measurement variance declined. A subsequent algorithm to identify hospitalized macrovisits reduced data variability further.
The authors emphasized that a “macrovisit” differs from pre-existing clinical service aggregation methods such as bundles and care episodes.
Care bundles usually refer to linking care services for the same medical situation over various periods to support bundled payment models.
The researchers said care episodes may be short and discrete, like care following minor trauma, or long such as the long-term range of services for chronic conditions.
“In contrast to bundles and episodes, macrovisits are intended for the much more focused purpose of linking encounters together to fully represent the services experienced during a discrete hospitalization, very similarly to the intrinsic linking of encounters inside many EHR systems for actions such as facility billing,” they explained.
While the rule-based algorithms used in the study lack the “apparent dynamism of a machine learning-based approach,” they are key steps in quantifying EHR data heterogeneity and creating solutions to harmonize data, the authors said.
They noted that there is speculation around the need for this type of work due to the perception that these issues will be resolved through harmonization pipelines of CDMs or new interoperability paradigms, such as HL7 FHIR.
“FHIR accounts for the possibility of aggregating encounters with the partOf element in the Encounter resource,” the authors wrote. “However, because partOf is not a required field in FHIR, it remains to be seen what proportion of FHIR-ready sites will choose to use this element and how much variation will be seen in its use.”
“Similarly, the experience of working across N3C, the largest harmonized CDM repository in the country, has demonstrated that the CDM harmonization mechanisms currently in place are not sufficient to harmonize encounter data,” they added.
The authors explained that assessing encounter heterogeneity and methods to aggregate encounters into larger hospitalizations is important because leveraging raw visit data misleads many analyses.
For instance, using raw inpatient visits to identify hospitalizations in N3C data led to an undercounting of severe cases of COVID-19.
While it may be tempting to attempt to solve this issue at the source (the EHR), the authors said combining visits into macrovisits post hoc instead allows for more definitional flexibility for projects and research questions with different needs.
“Using a post hoc method, the ‘raw’ transactional visits are always available in the source data instead of destroyed in a transformation that may be upstream and opaque to the end user,” they wrote. “This also leaves room for multiple shared macrovisit-like algorithms to serve different use cases, which, for example, may wish to preserve differences between inpatient stays and extended holds in the emergency department.”
They also suggested it would be worthwhile to consider CDM schema extensions to facilitate loading hospitalization and hospital facility data and groupings that already exist in EHR platforms, such as the “account” concept in the Epic EHR.
“While these concepts are unlikely complete solutions to the visit issues described and would likely have their own heterogeneity both within and between sites, they offer a significantly more evolved and refined mechanism for dealing with hospitalizations from the EHR,” the study authors said.