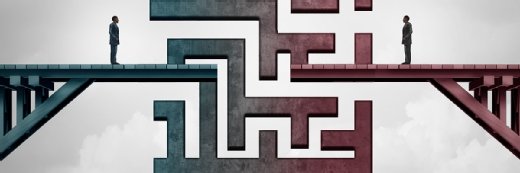
Getty Images/iStockphoto
Using EHR Data to Help Prevent Avoidable Hospital Transfers
Regenstrief research scientists have piloted a tool for nursing homes that uses EHR data to retrospectively flag avoidable hospital transfers to support quality improvement.
Researchers estimate that anywhere between 23 to 60 percent of hospital transfers of nursing home residents are potentially avoidable. A new tool that uses EHR data could help nursing homes prevent these unnecessary transfers and drive quality improvement.
“Around 50 percent or more of people living in nursing homes in our country have dementia,” Jennifer Carnahan, MD, MPH, Regenstrief Institute research scientist, told EHRIntelligence in an interview. “When you have dementia, changing your environment can be really hard on people, which often leads to delirium.”
Those transfers happen when a person gets sick with an infection, Carnahan added. Most potentially avoidable transfers in nursing homes are linked to one of five medical conditions: pneumonia, heart failure, urinary tract infection, dehydration, and asthma.
There are two main ways that stakeholders have identified avoidable transfers in the past for clinical quality measurement and nursing home process change, according to Carnahan. However, these approaches present several challenges.
One way is the use of CMS billing codes. For instance, if a nursing home resident goes to the hospital and is diagnosed with pneumonia, CMS would say that pneumonia is something that should have been prevented. However, that’s not always the case, Carnahan said.
“As a physician, I have a problem with that, because not every pneumonia is preventable,” noted Carnahan, who also serves as an assistant professor of medicine at the Indiana University School of Medicine. “There are times when people are just eating a meal and aspirate food and that causes pneumonia.”
“The question is whether or not we have what's needed to treat that pneumonia in the building,” she said. “A lot of times that depends on the goals of care and the severity of it.”
Organizations have also identified avoidable transfers through in-depth root cause analyses.
“Root cause analyses take highly skilled and trained nurses, though physicians have also been part of the process,” she explained. “They spend sometimes hours evaluating a transfer and deciding whether or not it was avoidable. While that's great, it's not as helpful when it takes hours and hours.”
Recognizing the limitations of traditional approaches, Carnahan and fellow researchers developed the Avoidable Transfer Scale as part of an eight-year CMS-funded demonstration project called OPTIMISTIC (Optimizing Patient Transfers, Impacting Medical quality and Improving Symptoms: Transforming Institutional Care).
For a year and a half, Carnahan and other clinicians worked with nurses from the OPTIMISTIC project to review transfers of nursing home residents who were part of the project.
“We really tried to drill down on what were the big issues in these transfers and when they were avoidable and when they were not,” she said. “Out of those sessions, we developed a list of questions that we eventually turned into the avoidable transfer scale. Then we tested it in some of the nursing homes that were participants in OPTIMISTIC.”
The tool aims to help fuel quality improvement initiatives by allowing nursing homes to retrospectively identify transfers to hospitals that could have been avoided.
For example, by using the scale, a nursing home could learn that three transfers to the hospital could have been potentially avoided because providers didn't have good conversations with the residents and their families about their goals of care.
“If they identify that, then they may initiate a quality improvement program to try to increase or improve the quality of these conversations so that we really know that we're doing what it is that people want,” Carnahan said. “Some people really do want to go to the hospital, and that's okay too. We just want to make sure that the transfers that are avoidable can be avoided.”
Importantly, the tool allows nursing homes to outsource quality improvement work related to potentially avoidable hospitalizations. This may be critical during public health emergencies like COVID-19, or other times when facilities have a lack of onsite staff available to focus on quality improvement initiatives.
“It's been designed so that people can use the electronic health record and remotely review what happened,” Carnahan said. “Someone wouldn't even need to necessarily be onsite. They can look at the electronic health record, see what the events were that led up to the decision to transfer the resident, and then sort of check off the boxes on the tool to see if this was a necessary transfer or not.”
Some of the scale’s prompts include:
- A pertinent nursing assessment was completed (within 12 hours) prior to transfer
- Staff reported warning signs of change in condition were reported to a supervisor
- Goals of care were honored at the time of transfer
- Available diagnostic services were utilized to evaluate/diagnose the change in condition
- At the time of transfer, the resident’s condition could not be safely assessed in the facility
The study of the avoidable transfer pilot was published in Innovation on Aging.
“This was a publication of our first trial run using it,” Carnahan said. “It definitely needs to be refined more, but we think there's a lot of promise in what we have so far. We're hoping that going forward, it will become more enabled to be used in nursing homes across the country.”
Carnahan said that Regenstrief is exploring how machine learning could be used to potentially automate the tool.
“Fully automating it is definitely exploratory, but it would be amazing if we could make this more automated because then it would be accessible in a much broader sense,” she said.
“We have a lot of amazing Regenstrief data scientists who are very well-versed in machine learning, but a lot of the tool relies on the clinical acumen of someone who's been trained clinically,” Carnahan said. “Some of the questions that tested the most strongly were things that I'm not sure machine learning would be appropriate for.”
For instance, the prompt “hospitalization was clearly part of the resident goals of care” requires an understanding of whether the provider was having high quality patient conversations.
“I'm not positive that machine learning will be able to necessarily identify that, although possibly some combination of natural language processing on top of that might be able to look in the electronic health record and say that they have this kind of conversation,” Carnahan said. “So, we're hoping in the future, we can automate things a little bit more, but we're not quite there yet.”