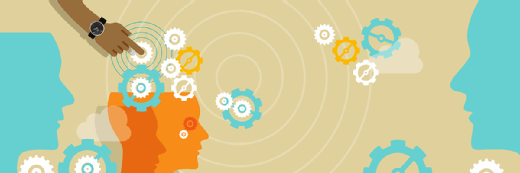
A glimpse into the future of AI enterprise applications
Hands-on experience building bots on the Azure cloud and briefings from Microsoft experts show that the future of AI is here, and it's more user-friendly than you might expect.
Microsoft's 2019 data and AI tech immersion workshop demonstrated the vendor's strategy to democratize AI by providing a small group of about 30 journalists, industry analysts and other tech industry experts with hands-on experience in programming AI bots using the cognitive services in the Microsoft Azure public cloud platform. It provided meaningful glimpses of the future of AI in enterprise applications, from prebuilt AI models in Azure and machine teaching efforts of today to a future quantum coprocessor that will one day function as Azure's sidekick in a hybrid computing model.
The immersion approach of the workshop, which I attended, mimics the real-world experience of AI users who aren't data scientists. Most attendees did not own or have access to the massive data sets needed to complete the exercises on a variety of real-life AI use cases. The software giant overcame that obstacle by providing an open remote desktop connection app on our individual workstations, giving us access to the immersion environment and preloaded data sets in Azure.
The vendor also provided the credentials we needed to access the data and complete the exercises, which means the sign-on process was different from that of a typical user, but we were required to jump through a few more hoops along the way. Even though some attendees grumbled about the extra steps, they helped to make clear that using AI was becoming considerably more user-friendly.
Why AI's budding user-friendliness matters to enterprises
Data democratization has been the key to reconfiguring companies to become data-driven enterprises. Democratizing AI will likewise become essential to unlocking every byte of data in ever-expanding data sets fast enough for responsive action to take place in real time.
Thus, AI "is in virtually every existing technology, and creating entirely new categories," according to the report, "Gartner Top 10 Strategic Technology Trends for 2019." Furthermore, the AI megatrend is far from peaking, despite a shortage of data scientists. According to a LinkedIn study, U.S.-based businesses have been hard pressed to fill more than 150,000 data scientist jobs. The study concluded that demand for data scientists will be off the charts for the foreseeable future.
The software industry is banking on more AI as the answer to its growing skills gap. In its 2019 trends report, Gartner also said smart automation and AI-driven development will resolve many talent shortage issues with technologies and best practices for embedding AI into applications and using AI-powered tools in the development process.
Gartner predicted that, "by 2020, more than 40% of data science tasks will be automated, resulting in increased productivity and broader use by citizen data scientists. Between citizen data scientists and augmented analytics, data insights will be more broadly available across the business, including analysts, decision-makers and operational workers."
The immersion experience at the Microsoft workshop, which took place in early spring, served to underscore these predictions. I was able to build an AI-based bot with the Virtual Assistant accelerator in a matter of minutes. The real-world scenario of an auto manufacturer seeking to make a bot to respond to driver voice commands and visual feedbacks made the exercise more meaningful.
It was only one of the day's four lab exercises to be completed in two hours. Considering I hadn't worked with the technology before, the fact that I could successfully complete the exercises in that short a time drove home how realistic the goal of AI democratization truly is.
The day before, the workshops centered on data, the key component in training and using AI. I was far more familiar with Azure SQL Data Warehouse, Azure Databricks, Azure Data Factory, and Microsoft Power BI. It was obvious that these technologies, too, are getting progressively easier for users with diverse skill sets to master.
The future of AI
While it's clear that prebuilt and pretrained AI models are on the upswing as an essential element in AI democratization, that's not all that is in store for the future of AI. Here are some of the most interesting concepts presented at the workshop.
Machine learning is enhanced by new machine training techniques. Classic machine learning refers to systems that can learn from data, identify patterns and make decisions with little to no human intervention. Analytical model building is thus automated. But that way of learning involves a great deal of hit or miss, and it is highly subject to the quality of the data. Limitations in the data can skew outcomes.
For example, if the data is solely about crashed airplanes, then the machine learning's universe is crashed planes, and it can't learn or consider things that kept planes from crashing. This can be problematic if the intent is to teach the machine how to spot things that will cause or prevent crashes. Human bias is often unintentionally introduced to machine learning this way. Thus, ensuring the quality of data means more than just making sure spreadsheet fields are uniform or customer information is current.
New machine training (or machine teaching) techniques will make AI smarter by enhancing the information and experiences it learns from. Machine teaching provides the abstraction and tooling for developers, data scientists and subject matter experts to program domain-specific intelligence into a system, according to Microsoft.
"Machine teaching plans are independent of underlying [machine learning] algorithms," said Gurdeep Pall, corporate vice president of business AI at Microsoft. "It also makes use of reusable code."
Another form of machine training that will become increasingly prominent is reinforcement learning, which enables a system to learn how to make decisions in complex, unpredictable environments based on external feedback.
For yet another approach to machine training, take a look at Project Brainwave, a Microsoft deep learning platform for AI in the cloud that is used in the Microsoft Bing search engine. A few early applications -- of which ResNet-50, a neural network that can classify images, is the first -- are publicly available in Azure Machine Learning Hardware Accelerated Models.
Simulation training for machine learning will be used where data is sparse or difficult to obtain. Using simulations for machine training is important because it isn't practical to operate a physical device in all scenarios.
"Examples include autonomous wind turbines. Humans can't easily gather data from them because that is dangerous and requires shutting them off. Sensors may not get all the data you need because of the harsh environment, and drones might get damaged or destroyed trying to get close enough to gather data from one running turbine in a field of running wind turbines," Pall explained. "We can build simulators using 3D modeling, machine teaching, photorealistic data and physics data, among other data, to accurately train the system."
There's another huge plus to using simulators to train machines. "We can overclock the real world in simulators, which enables a machine to learn a million times faster than in the physical world," he said.
Quantum computing could enable artificial general intelligence. Yes, scientists have been promising quantum computing will be real in five years for decades now. But progress is being made.
For example, the Microsoft Quantum Development Kit was released in December 2017 to aid developers in building applications for a quantum computer. The March 2019 update added support for cross-platform Python host applications to make it easier to simulate operations and functions of Q# (Q-sharp), a programming language for quantum computing. It also brings Q# programming to Jupyter Notebooks, an online collaborative development and data science environment.
While several vendors are working simultaneously to bring quantum computing fully into reality, they aren't all approaching the problems in the same way. That's because quantum bits, called qubits, are very fragile and highly susceptible to even miniscule environmental interferences.
"All qubits are not created equal," said Krysta Svore, general manager of quantum software at Microsoft. "Our qubit is more scalable and more stable."
Microsoft's qubit is a topological qubit, which achieves at least partial protection from interference by splitting an electron, creating the effect of data redundancy. This is known as electron fractionalization.
Svore said Microsoft's idea is to first make a quantum computer that works as "a coprocessor with classical computing -- a hybrid." And, yes, she too predicted there will be a functioning quantum computer within five years.
Whenever quantum computing does arrive in force, AI will spring light-years ahead, according to Svore. "It moves us closer to artificial general intelligence," she said. Unfortunately, it also means traditional cybersecurity will turn to dust. "But we're prepared for that and planning now for new quantum-based cybersecurity applications," she said.