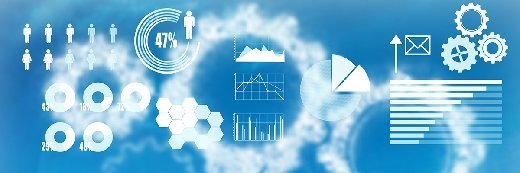
WavebreakmediaMicro - Fotolia
A deep dive into Oracle Adaptive Intelligent Apps
Oracle Vice President Melissa Boxer provides details on how Oracle Adaptive Intelligent Applications take 'repetitive and mundane' ERP and supply chain tasks off people's hands.
Oracle has been investing heavily in making it easier to weave AI and machine learning capabilities into a variety of applications, especially its Oracle Adaptive Intelligent Applications. In theory, this should facilitate automating many aspects of ERP, supply chain and revenue-management processes. But as with most cutting-edge technologies, the devil is in the details.
Melissa Boxer, vice president of adaptive intelligence at Oracle, explained what these capabilities mean in practice. The vendor considers its Oracle Adaptive Intelligent Apps a strategic initiative to develop next-generation smart applications using big data on top of the Oracle Cloud. Boxer responded to questions via email.
What are some of the key ways of embedding machine learning into the Oracle Cloud Applications, particularly ones tied into ERP and supply chain apps?
Melissa Boxer: AI and machine learning can be embedded within Oracle Cloud Applications in many ways. For example, the automation of repetitive and mundane tasks, such as expense approvals, invoice matching or categorization of data for suppliers, or recommendations for next user actions are low hanging fruit and issues that can be addressed with AI/[machine learning] within cloud applications today.
Machine learning can address specific use cases within common business processes, such as accounts payable and procurement. We then build out a capability with the end user in mind that leverages Oracle's machine learning algorithms to make the process more efficient. This capability is natively connected to the Oracle Cloud Applications. Many of these use cases require data from multiple sources -- service and supply chain, finance and HR. The machine learning works with all first-party data in a customer's applications and then adds third-party data from Oracle Data Cloud and Oracle DataFox to provide more context and enable smarter recommendations.
One use case that pulls data from both ERP and [Supply Chain Management] is Intelligent Payments, which enables customers to take advantage of in-the-moment supplier profile and risk data to generate vendor-specific offers in exchange for an early payment. Another is Intelligent Supplier [Management], which leverages AI-sourced and managed company profiles in Oracle DataFox to help identify which suppliers might be candidates for the Intelligent Payment Discounts, a dynamic discounting program.
What do the pre-tuned data models you are incorporating into the Oracle tools mean in practice? In what way are they pre-tuned, and how does this make life simpler for companies?
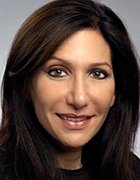
Boxer: Machine learning requires accurate, precise and relevant data to deliver optimum results. One thing is for sure, if you feed poor-quality data into machine learning models, you'll get poor-quality results. That's why we're particularly attentive to the data that feeds Oracle Adaptive Intelligent Apps models and use a combination of first-party and third-party data.
Tuning requires the appropriate data records to be fed into the Oracle machine learning models, using training data and machine learning algorithms specifically selected for a particular use case, such as a process or outcome that we are optimizing.
Pre-tuning provides our customers the benefit of immediate value when the application is implemented, avoiding the cold start problem. Over time, the models are continuously refined using the customer's unique data so that the outcomes continuously improve.
We know that not all organizations can afford to hire a team of data scientists and retain them. The idea behind pre-tuned data models means no data scientists are required.
In what ways will enterprises further need to tune or optimize these models for their use cases?
Boxer: No two businesses are the same, so we wouldn't expect the AI we've developed for common use cases to work perfectly straight away. The system essentially needs to learn the customer's business, which requires learning from the customer's response to its recommendations. Every time the system makes a recommendation, it learns from the customer's response to that recommendation, such as if it's accepted or ignored. This feedback helps the machine learning algorithms tweak and tune themselves to be more intelligent and effective.
Can you give some examples of how this works in the Oracle Cloud Applications? How is this kind of capability surfaced to users?
Boxer: The AI is embedded in the applications that customers are already using, so they don't have to leave a familiar interface. Often the recommendations manifest in slide outs or notifications within the application interface itself. But sometimes they require new interfaces -- for example, with intelligent expense audit and the expenses chatbot. Much of the user experience on the manager's side remains unchanged, but they have less work to do because the machine learning is auto-approving expenses within the normal parameters and only flagging the ones that are anomalies.
However, on the employee side, the chatbot engagement is a new interface, designed to provide a better mobile experience and enable employees to submit expenses on the fly by answering a few simple questions that help properly categorize and report the expense. This simple addition can save hundreds or thousands of hours for employees and managers who have better things to do than expense reports. The AI use case also improves accuracy of reporting and mitigates risk.
How does Oracle make it easier to use third-party data in these kinds AI-infused applications?
Boxer: Third-party data is activated at runtime as part of our Customer Experience B2C offerings and natively connected with Oracle Engagement Cloud for company-level data. As a result, data is continuously updated and is always fresh and accurate.
In Oracle DataFox, we have more than 3.5 million business profiles enriched with 68 dynamic signals. The Oracle DataFox AI engine applies natural language processing to more than 70,000 news articles daily to look for signals such as 'lost C-exec,' 'signs of growth,' 'file for bankruptcy.' In addition, Oracle Data Cloud is the world's largest pool of third-party data. This large pool of contextual data enables Oracle to deliver account prioritization in Oracle Engagement Cloud, next best offers and product recommendations in Oracle Marketing Cloud and intelligent supplier categorization in Oracle ERP Cloud.
What are the business arrangements that Oracle sets up to subscribe to this data? Is there an app store for data or do businesses have to negotiate with each data provider?
Boxer: We include access to Oracle DataFox and Oracle Data Cloud data for Oracle Cloud Applications customers who are leveraging our suite of AI capabilities. To promote time to value, we include the appropriate data as part of each application and remove the complexity for our customers. There are no additional subscriptions to data services required.
Can you give examples of how this could be used, or has been used, in practice to make better decisions?
Boxer: Better data equals better decisions. You can have the best machine learning algorithms, but it will all be for nothing if the data that they are processing is stale, inaccurate or limited in scope. Third-party data, added from Oracle DataFox and Oracle Data Cloud, enables us to add more context, which results in better recommendations.
For example, a North American retailer had a corporate mandate to deliver personalized marketing communications for each of their 60 million customers. To accomplish this, they need to coordinate customer information across channels and systems, communicate with one voice to the customer across channels, deliver personalized customer journeys through intelligent automation and drive and optimize campaign execution with artificial intelligence.
The customer used Oracle AI Apps to generate product recommendations based on first-party customer data and Oracle Data Cloud anonymized data in their Responsys marketing emails. After an initial pilot of 500,000 emails, AI-driven campaigns showed a plus 4% to 5% lift. They're now planning to ramp up their volumes by 10 times, and the company's goal is to have AI driving the execution of 50% of their marketing campaigns by the end of 2019.