digital twin
What is a digital twin?
A digital twin is a virtual representation of a real-world entity or process.
It is composed of the following three elements:
- A physical entity in real space.
- The digital twin in software form.
- Data that links the first two elements together.
A digital twin functions as a proxy for the current state of the thing it represents. It also is unique to the thing represented, not simply generic to the category.
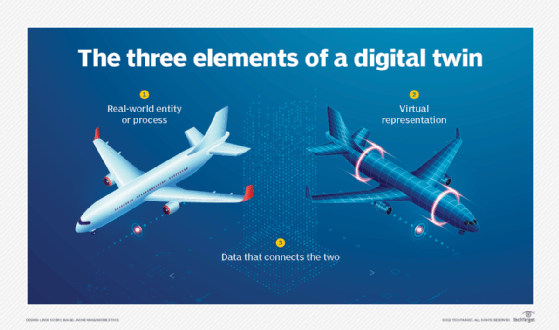
While many digital twins have a 2D or 3D computer-aided design (CAD) image associated with them, visual representation is not a prerequisite. For example, the digital representation, or digital model, could consist of a database, a set of equations or a spreadsheet.
The data link, often but not necessarily two-way, is what differentiates digital twins from similar concepts. This link makes it possible for users to investigate the state of the object or process by querying the data, and for actions communicated through the digital twin to take effect in its physical counterpart.
The Digital Twin Consortium, an industry association working to build the market and recommend standards, adds an important phrase to the basic definition: "synchronized at a specified frequency and fidelity."
These qualifiers refer to three key aspects of the technology:
- Synchronization ensures the digital twin and the represented entity mirror each other as closely as possible.
- The frequency, or speed, at which data gets updated in a digital twin can vary enormously, from seconds to weeks to on demand, depending on the purpose.
- Fidelity is the degree of precision and accuracy of the virtual representation and the synchronization mechanism.
Digital twins are created using the same CAD and modeling software that designers and engineers use in the early stages of product development. The difference with a digital twin is that the model is retained and updated for later stages of the product's lifecycle, such as inspection and maintenance, and often managed in product lifecycle management (PLM) software.
The physical connections between the entity and its twin are most often internet of things (IoT) sensors, and a mature IoT implementation is often a prerequisite for digital twins. It's also been said that IoT equally needs digital twins because they bring structure, analytics and usability to IoT data that is otherwise disorganized and hard to interpret.
Finally, analytics, often bolstered by artificial intelligence (AI) and machine learning, is essential in processing and analyzing digital twin data.
Types of digital twins
Several ways of categorizing digital twins exist, but the following four categories, organized in a hierarchy, are the most common:
- Component twins (also referred to as part twins): The most basic level; it's not for simple parts like screws but for things like mechanical subassemblies.
- Asset twins (product twins): Two or more components whose interaction is represented in the digital twin.
- System twins (unit twins): Assets assembled into a complete, functioning unit.
- Process twins: Systems working together to serve a larger goal.
Some observers employ less abstract categories for digital twins and name them by the type of physical object they represent. Examples are infrastructure twins for highways and buildings, and network twins for processes such as supply chains that involve entities operating in a network.
Benefits of digital twins
Because they're virtual, digital twins can reduce the cost and risk of having to work on the physical things they represent. Further benefits include the following:
- Improved operational efficiency from having more timely data and faster, more effective production.
- More effective and less expensive R&D and reduced time to market because physical prototypes, which can be expensive and hard to modify, are replaced with virtual prototypes, which are more flexible and produce more data.
- Better product quality as digital twins help identify defects earlier in the production process.
- Longer equipment uptime from predictive maintenance enabled by analyzing individual digital twins instead of having to shut down all the equipment to isolate a problem.
- More accurate and efficient remote monitoring of facilities and equipment through integration with their digital twins.
- Improved product end-of-life processes, such as refurbishment and recycling, thanks to more accurate information about the age and contents of a product.
Digital twin interoperability
To be useful, digital twins must work in a variety of enterprise applications.
While they usually start in CAD and PLM, some digital twins are also managed in ERP and material requirements planning (MRP) software.
ERP and MRP store the bill of materials (BOM), a comprehensive inventory of the materials and parts needed to make a product and typically a major contributor of digital twin data. ERP and MRP together also run many of the supply chain and production processes that go into manufacturing a product; along the way, they collect much of the data that goes into the digital twin.
Another common source of digital twin data is the manufacturing execution system (MES) that many companies use to monitor, control and optimize production systems on the factory floor.
Enterprise asset management software, which is increasingly the preferred system companies use to manage the purchasing, monitoring and maintenance of their most valuable equipment, also has to be integrated with digital twins.
In addition, public cloud providers that offer digital twin services, such as Amazon Web Services and Microsoft Azure, integrate with enterprise applications. It's even possible for digital twins that reside in different levels of the hierarchy -- asset twins and process twins, for example -- to be spread across different cloud services.
Challenges of digital twins
Organizations looking to develop digital twins face other daunting hurdles. Here are six of the biggest digital twin challenges:
Data management. Data cleansing is often needed to make data from a CAD model or IoT sensor usable in a digital twin. A data lake might need to be established to manage the digital twin data and perform analytics on it. Deciding who owns the data is another problem.
Data security. Digital twin data is timely and mission critical, but it also travels through several networks and software applications, which makes securing it at every stage challenging.
IoT development. As the preferred data source for most of the real-time and historical data about an entity or process, IoT sensors are usually a basic requirement of digital twins. Implementing IoT presents its own challenges in network infrastructure and storage capacity, device and data security, and device management.
System integration. Digital twins often begin life in CAD software but get more use in PLM, where they're used in post-sale services, such as performance monitoring and equipment maintenance. Numerous CAD and PLM software vendors have one-to-one integrations, but it isn't always adequate and smaller vendors might have no built-in integration.
Supplier collaboration. The numerous participants in a supply chain must be willing to share information from their own production processes to ensure that the information in a digital twin is complete.
Complexity. The data collected in the different software applications used by a manufacturer and its suppliers is not only voluminous, it changes often. Last-minute design changes, for example, must make it into the final version of the twin so the customer and manufacturer have the most current information.
Digital twin effect on environmental sustainability
Manufacturers use digital twins to improve the efficiency of production processes and conserve resources such as water and energy, as well as reduce pollution and waste in factories. Some are starting to modify digital twins explicitly for sustainability objectives, such as optimizing energy management and reducing carbon emissions.
Digital twins are also used to improve the sustainability of products by improving supply chain traceability and enabling better control of the entire product lifecycle, from raw materials to production, use and disposal.
On the other hand, there is an increasing awareness that digital transformation initiatives that involve digital twins, AI or blockchain require powerful new data centers that draw substantial resources, including land, energy and water.
_mobile.jpg)
Use cases and examples of digital twins
The initial deployments of digital twins were mostly directed at the design, production and maintenance of extremely high-value, physically large equipment, such as airplanes, buildings, bridges and power-generation plants where mechanical failure can be life threatening or cause financial losses that exceed the significant expense and effort of developing a digital twin.
The following industries are seeing the most activity in planning or deploying digital twins:
Manufacturing. The industrial world is widely acknowledged as the pioneer in the use of digital twins and has seen the broadest deployment. For several years, manufacturers have been making digital twins of parts, products and systems and are beginning to deploy process twins that model production processes and sometimes entire factories.
Utilities and energy. Electric companies are using digital twins to design, monitor and maintain power plants, electric grids, transmission and consumption. The technology could also help improve the efficiency of renewable energy systems, such as solar installations and wind farms, whose production is less predictable than fossil fuel-burning plants. Process digital twins could someday mirror entire electric grids.
Healthcare. Digital twins built on electronic health records, medical images, genome sequencing and other medical information could make it easier for providers to diagnose illnesses and recommend treatments by comparing a patient's digital twin to that of other patients with similar profiles. Medical testing could be done more efficiently by avoiding the risks of using real patients. Researchers are already running simulations on anonymized digital twin data to identify the best therapy options.
Urban planning and construction. Digital twins are being used in the design of large buildings and offshore oil rigs. Some users are vastly expanding construction twins to encompass neighborhoods and cities, with a focus on infrastructure. The UK even tried to develop a national digital twin but ended the five-year initiative in 2022. Digital twins also have a role in smart city initiatives, which aim to digitally connect infrastructure, often through IoT, and apply AI and analytics to the data, making transportation more efficient and conserving energy, among many goals.
Automotive. Digital twins play their usual role in the vehicle product-design stage, but also in later stages of the vehicle lifecycle, such as service. Automakers are also using digital twins to make assembly plants more efficient. Digital twins are expected to reduce massive recalls by allowing each vehicle's unique twin to be analyzed for defects. Digital twins of customers enable virtual test drives of vehicles.
Retail and e-commerce. Retailers use digital twins to model product placement, the customer's journey through a store and the impact of new store layouts. Some companies have started using the technology to build online twins of their stores to boost interest in their e-commerce sites. Digital twins are also helping to improve the realism of 3D product images.
Digital twins in the metaverse
Digital twins are one of the building blocks of the metaverse, a broad concept that incorporates technologies such as virtual reality that enable immersive and highly interactive digital worlds. Proponents are working to re-create in the metaverse many of the elements that people interact with in the real world. For example, in an enterprise metaverse, digital twins of various aspects of an organization are integrated to simulate operations and improve decision making.
Augmented reality, another foundational technology of the metaverse, can overlay a digital twin on the object it represents to provide field technicians with more detailed maintenance data. Digital twins could also provide some of the data for images in virtual reality.
Both the metaverse and digital twins demand herculean efforts to capture, then digitally mirror, things in the world. For digital twins, the process is called reality capture. It's usually done with a laser scanner that directs a laser beam at an object and the surrounding space to capture measurements. Laser scan files are then combined into a point cloud, a set of points in 3D space that outline the geometry of the scanned object. Color is often added and the point cloud is imported into CAD software for further enhancement.
Reality capture technology has expanded beyond laser scanners to include smartphone software and drones, among others, which should help expand its use in digital twin development. In addition, advances in AI promise innovation in how 3D representations are created and stored. For example, neural radiance fields (NeRFs) use advanced machine learning to generate 3D representations from 2D images and compress them down to a fraction of the size of traditional images. A related approach called Gaussian splatting promises higher-quality, more interactive images and could accelerate the building of the industrial metaverse, in which digital twins play an important role.
For more on the metaverse, read the following articles:
Metaverse pros and cons: Top benefits and challenges
Metaverse interoperability challenges and impact
Metaverse privacy concerns and how to address them
The Metaverse Standards Forum: What you need to know
Metaverse vs. multiverse vs. omniverse: Key differences
Digital twins vs. simulations
While simulations are sometimes executed on digital twins, they aren't a defining feature of digital twins. Conversely, simulations aren't automatically digital twins, because they usually lack the strong network connections to the represented entity and a data model that organizes the information. Furthermore, most simulations focus on a single process, while a digital twin is usually more complex and contains enough data to accommodate several simulations.
Marketing hype often confuses the two, but the differences are easy to keep straight.
Simulations are usually done in CAD software during the design phase of a product or process. Engineers create 2D or 3D models, introduce variables and observe how they affect outcomes.
In contrast, digital twins exist throughout the entire product lifecycle. Over time, they acquire substantial quantities of real-time data that allow them to evolve and represent more accurately the current state of their physical counterparts. Simulations done on digital twins are more active and involve many variables, while those done in CAD are static and typically use a small number of variables. CAD simulations are theoretical, while digital twin simulations are connected to the real world.
How an organization can get started in digital twin technology
McKinsey & Company advises organizations to get their data house in order before deploying digital twins. That means building and maintaining high-quality data infrastructure that provides reliable data.
According to McKinsey, there are three main steps in developing and scaling digital twins:
- Create a blueprint. Define the types of twins to be built, how their capabilities will evolve and the order that will maximize their value and reusability. Establish ownership and governance structures.
- Build the base digital twin. Assemble the core data product, which enables developing visualizations and the first use cases.
- Expand and improve the digital twins. Add data layers, more advanced analytics, AI and simulations.
Organizations can build on the initial, reusable data set and digital twins to continue expanding use cases, with the twins evolving based on real-world feedback and acquiring more predictive capabilities. Connecting the twins to form process twins then makes it possible to simulate the relationships between entities. For example, integrating the digital twins of customers with the twins of retail stores, e-commerce sites and supply chain infrastructure enables a company to analyze and simulate business strategies and eventually build an enterprise metaverse.
History of digital twins
Many experts trace the beginning of the digital twin concept (though not the name) to 1969, when NASA augmented training simulators with telemetry data from the damaged Apollo 13 spacecraft to diagnose and solve problems. The concept of digital twins was first expounded upon in 2002 by NASA consultant Michael Grieves, an academic who is influential in PLM, and significantly expanded on in subsequent years. In 2010, Grieves collaborator John Vickers, manager of advanced manufacturing at NASA, began applying the term digital twin to the concept.
In the ensuing two decades, beginning with manufacturing, digital twins have expanded to other industries, become a standard feature of CAD and PLM software, risen to the top of vendor and market-research hype cycles and begun to take root in the popular imagination.
The future of digital twins
In the near term, developers are likely to expand the use of digital twins to a broader range of entities, from body parts to people, from smart cities to global supply chains.
As the price of digital twin technology comes down, companies will no longer limit its use to their most expensive, mission-critical equipment. The continued growth of digital twin development options, IoT infrastructure and related concepts like the metaverse should help popularize digital twins and make them easier to deploy.
Most market researchers expect growth to skyrocket. For example, Global Market Insights expects a compound annual growth rate of 25% that will take the digital twin market to $90 billion by 2032.
Major advances in digital twin technology are on the horizon. For example, researchers have been discussing cognitive digital twins that would have generative AI and machine learning capabilities that allow them to function as intelligent companions to their real-world counterparts. Software developers are working on alternative database schemas, including semantic networks, that can unify the myriad data sources that feed into digital twins and make them more suitable for training generative AI.
Digital twin optimists imagine a day when intelligent digital twins will become ubiquitous at every level of complexity, from household objects to the global environment. Whether this level of digital transformation is realistic, let alone desirable, is open to debate, but few would dispute that digital twins have secured a role in that future.