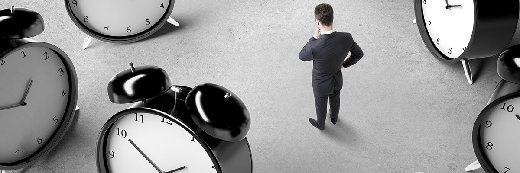
peshkova - Fotolia
Can modern technology solve demand forecasting problems?
Predicting demand has never been easy, but in the age of e-commerce and omnichannel, that difficulty has multiplied. Here is some helpful guidance for organizations.
Demand forecasting problems have always existed, and they've only gotten worse. Technology can help, but there's no silver bullet.
The basis of all forecasting is an analysis and mathematical modeling of past demand, and a number of programs can do this. Comprehensive planning suites include these programs and they are available as specialty add-ons to work with a company's ERP or demand management software. The science of statistical forecasting continues to advance with the evolution of advanced mathematics, machine learning, AI and simulation. In addition, as IoT sensors and other technology more closely tracks sales and material movement throughout the supply chain, organizations can get better source data, which in turn helps create better forecasting.
Supply chain stakeholders who want to address demand forecasting problems should take advantage of the new and improved forecasting tools. A 10-year-old forecasting module that came with the existing ERP system is probably not going to address modern demand forecasting problems, such as those posed by omnichannel. Companies should upgrade or replace an old forecasting module with one that incorporates modern technologies that can make use of IoT-fed data streams.
Shifts in demand forecasting problems
Mathematical forecasts are based on the assumption that past demand can be a useful indicator of future demand. They create a model of past demand and project it into the future. But that basic assumption only holds true in general, and not forever.
Consumer tastes change quickly, markets shift unpredictably, and producers, sellers and distributors react. None of that is considered in the mathematical models. So there has always been a need to apply these so-called extrinsic factors on top of the basic forecast. Even with the new advanced forecasting systems that doesn't change much, although users may be able to input additional data sources such as demographic trends and economic indicators. Even then, a user will likely need to modify the forecast using a best guess as to how the forces of the market and the realities of competition and consumer behavior might change demand in the foreseeable future.
Machines are continually getting smarter and more artificially intelligent, but they are unlikely to be able to replace the human element in demand forecasting anytime soon.