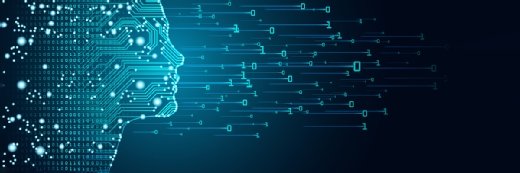
Getty Images
Unlocking the potential of white box machine learning algorithms
Transparent, explainable machine learning algorithms have demonstrated benefits and use cases. Although white box AI is nascent and largely unknown, it's worth exploring further.
The notion of white box AI indicates AI systems with algorithmic transparency and human-comprehensibility. While glass box AI and transparent AI may be more accurate and understandable monikers, the concept is what's most important. This approach to AI and machine learning assures others that algorithms are acting without bias and trustworthy.
More opaque ML algorithms (also called the black box approach) can produce useful results that organizations and even governments embrace. Yet they don't ensure that humans understand why they produced these outputs or decisions.
Black box AI is doing well thanks to the successes of Stable Diffusion and GPT-3 as well as programs based on them, such as Dall-E. There are also a lot of these tools available in the marketplace for businesses to use in developing their own applications. Because they are built on neural networks or other opaque architectures, the inner workings of these systems -- the processes by which an output is generated based on an input -- are not explainable. The full potential of transparent AI is still in the works and hasn't been fully unlocked.
As AI gets introduced to more contexts with direct and important impacts on humans -- and as decisions come to be more obviously and visibly life or death or life altering -- the desirability of explainable, accountable and understandable AI is growing. When humans see why an algorithm acts as it does, they can take the following actions:
- They can explain to those affected by a decision how that decision was made, thus making explainable AI and accountable AI two new buzzwords in the ever-growing AI lexicon.
- They can verify the AI is not making decisions based on incorrect data.
- They can ensure the AI is not ignoring relevant information available to it.
- They can find accidental and undesirable biases in algorithms.
- They can identify maliciously introduced biases in algorithms.
Whitebox AI Use Cases
Any AI use case can theoretically be a white box AI use case. There is no reason an AI system must be opaque or unexplainable. However, interest in AI that is transparent and explainable is highest in environments closely linked to human wellbeing. These include the following uses of AI in decision-making and in direct control of the physical world:
- making government-level decisions (e.g., about whether to fund a new stadium);
- making law enforcement and criminal justice decisions;
- making medical decisions, (e.g., who should be allowed to take an experimental drug);
- making urban planning decisions;
- making major financial decisions;
- controlling moving vehicles (especially self-driving cars); and
- controlling medical devices.
In these and many other situations, employees in government, banking, medicine, law enforcement and justice organizations want to answer questions about how and why decisions were made and defend them as reasonable decisions. People living with the consequences of those decisions want to get comprehensible answers when they ask.
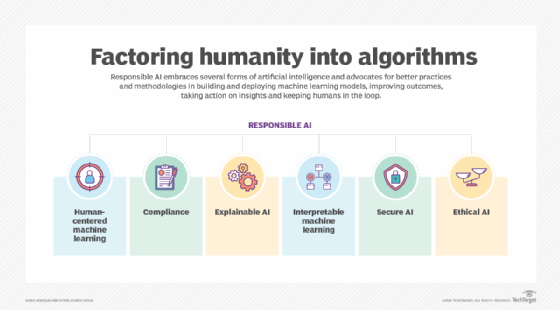
The future of white box AI
Because there are fewer commercially available white box AI systems to use in building a solution, organizations requiring one must build their own for the foreseeable future. However, many academic programs that teach AI, including programs at Johns Hopkins and Michigan State, include courses covering the ethics of AI and courses teaching white box techniques. Some schools have research institutes or programs built specifically around white box principles, such as Columbia's Data Science Institute or Stanford's Institute for Human-centered AI.
The scope of white box AI's role in the future depends on two factors. First, it depends on the accumulation of expertise and evolution of underlying platforms. As we have seen in the black box space recently, once there are enough skilled people, mature projects and focused startups in a space, progress accelerates sharply. Second, it depends upon the progress of laws and regulations governing AI in commercial and governmental spaces.
The implications of existing legal frameworks, like the GDPR, for how AI can and must function are still being worked out. New laws and agency rules will follow in jurisdictions around the world. If they incline toward requiring transparent, explainable and accountable AI, the white box approach will dominate the future landscape.