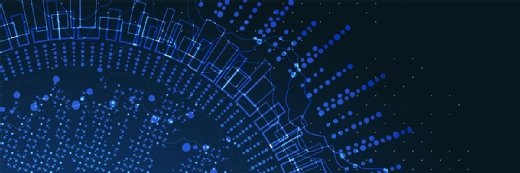
KOHb - Getty Images
The data privacy risks of third-party enterprise AI services
Using off-the-shelf enterprise AI can both increase productivity and expose internal data to third parties. Learn best practices for assessing and mitigating data privacy risk.
Outsourcing AI application development or deployment to a third party is simpler -- and potentially more cost-effective -- than building AI applications in house, making it an attractive option for many organizations.
Vendors offer third-party AI services, such as Microsoft's Azure OpenAI Service, for developing and operating AI models or applications. This capability is attractive to businesses that lack the in-house expertise or infrastructure to build AI apps on their own. Rather than creating AI software from scratch, businesses can turn to preexisting AI models and services to meet their needs.
But using third-party AI tools also raises major data privacy and security concerns. Although these data privacy risks don't always outweigh the benefits, they are an important factor to consider when choosing whether to build an AI app or rely on third-party services. For businesses that opt to outsource some or all of their AI development and deployment, it's essential to have a plan for managing data privacy issues.
The data privacy risks of third-party AI
From a data privacy perspective, third-party AI tools present several challenges, mainly because using a third-party AI service often requires enterprises to expose private data to that service.
For example, imagine that an organization wants to deploy a generative AI application that can produce marketing content tailored to its business products. If the company outsources this work to an external AI service, that service would likely need to be trained on material such as internal product documentation and roadmaps. That data might contain business secrets that the organization doesn't want to share with outsiders.
Using third-party AI tools doesn't always require exposing proprietary information to external providers. If an AI tool doesn't need to make decisions tailored to the specifics of an organization, it won't need to be trained on internal data.
That said, enterprise AI applications capable of more than producing generic recommendations or content typically do require access to an organization's internal data. Parsing that data is the only way these tools can make decisions and take actions tailored to the company's specific needs.
The lack of transparency in AI
But doesn't this issue apply when using any type of external application or service, not just outsourced AI?
That's true -- to a point. Anytime a business deploys a third-party application and allows it to access corporate data, the organization is exposing information to an external provider. Using a SaaS tool for email or customer relations, for instance, involves connecting some internal data to a third-party service.
However, the key difference is that AI apps and services can share data in ways that are not at all clear and transparent. Traditional third-party apps and services typically let users define clear boundaries for how their organization's data can or can't be used. A business can expect, for example, that its SaaS email vendor won't make the company's emails visible to other organizations that use the same email platform.
But the complexity of AI models, combined with the novelty of AI and lack of AI governance standards, makes it much harder to establish transparency surrounding the use of private data in the context of AI. Third-party AI vendors might not be willing -- or able -- to guarantee that the proprietary data that users expose to their models won't be used for future model training.
If those models are later shared with other organizations, business users' data will then be indirectly used beyond the confines of their IT estate. An organization could even end up in a scenario where competitors gain access to insights gleaned from analysis of its data if competitors use the same third-party AI services.
Complicating this challenge is the fact that AI models never really "forget" the insights they learned through training. Even if data ceases to be directly available to a third-party AI app or service, the app or service can continue to make decisions based on that information if it was previously included in training data.
This is where AI differs from other types of tech services; users don't face these sorts of issues when dealing with other third-party software tools. A business can ask a SaaS vendor to delete its data from the vendor's platform, but it can't ask an AI vendor to make its tools forget that data.
Consider open source AI
Open source AI models and tools represent something of a middle ground between in-house and outsourced AI, providing enterprises with the algorithms they need to develop AI tools without writing everything from scratch. Enterprises can deploy open source models themselves by training them on their internal data without exposing that data to a third party. This approach might be attractive to businesses that have some ability to deploy AI apps themselves, but don't want to design and implement the models from the ground up.
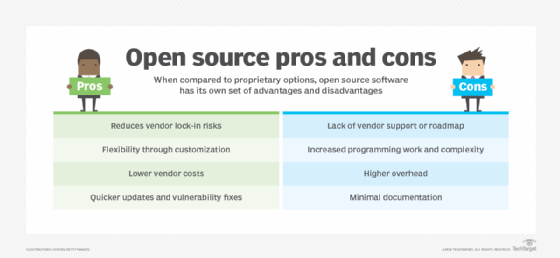
How to mitigate the data privacy risks of third-party enterprise AI
Fortunately, organizations don't have to accept these privacy pitfalls to take advantage of outsourced AI apps and services. The following are a few steps enterprises can take to reduce data privacy risks when using external AI tools.
1. Demand vendor transparency
The first step businesses can take to guarantee data privacy in the context of outsourced AI is to require transparency from AI vendors about how private data is used. For example, an enterprise can ask for contractual guarantees that its data will not be used to train models that are shared with its competitors.
2. Manage data exposed to AI
Another simple but effective means of mitigating data privacy concerns is to establish firm controls over which data the organization shares with third-party AI services.
Doing so requires two key resources:
- Internal data governance practices that enable the organization to separate data intended for exposure to AI models from other data.
- Content filtering support in the chosen AI service, which lets the organization control which types of information the service ingests.
This strategy doesn't eliminate data privacy risks, but it reduces them by enabling organizations to restrict the types of internal data the AI model can access.
3. Desensitize data
Data desensitization is the process of removing sensitive information from a data set. For example, an organization planning to feed a customer database into a third-party AI tool could replace actual customers' names and addresses with made-up placeholders. Doing so could help avoid compliance and governance issues related to the management of personally identifiable information.
However, this strategy comes with a potential pitfall: making the model less effective by inserting fake information into training data. Swapping in made-up customer addresses could cause an AI model to misinterpret the places where actual customers live, for example. Thus, the benefits of this AI data privacy protection method must be weighed against the resulting potential reduction in model effectiveness.