Successful generative AI examples and tools worth noting
Industries are using generative AI in various ways to generate new content. Learn about successful examples of this technology and notable tools in use.
Generative AI technology, as the name implies, generates outputs based on some kind of input -- often a prompt supplied by a person. Some GenAI tools work in one medium, such as turning text inputs into text outputs, for example. Others transform among media: turning a text prompt into graphical output, perhaps, or a sound prompt into a textual output.
The most headline-grabbing uses of generative AI tools involve text generation. With the public release of ChatGPT in late November 2022, the world at large was introduced to an AI app capable of creating text that sounded more authentic and less artificial than any previous generation of computer-crafted text. Humans eagerly adopted it, making ChatGPT the fastest-growing app in history while using it for seemingly endless text-generating purposes, from writing term papers and taking a state bar exam to writing the scripts of the news stories about ChatGPT's capabilities.
GenAI's transformative role in various types of content
Text is not the only area where generative AI is pushing boundaries. Here are some examples of its broad impact.
Text-to-speech. Whether its own words or someone else's, generative AI is advancing speech synthesis, improving the quality of artificial readers for e-books, synthetic presenters for news clips and advertising -- including clickbait posts on social media -- synthetic characters in video games and literal chatbots that answer phone calls. Intonation, cadence and volume variations are all becoming more realistic, subtle and flexible. The improvement in quality is also increasing the threat of deepfake audio.
Image synthesis. OpenAI's Dall-E 2 and other products (see "GenAI tools" section below) use AI to create pictures based on text descriptions. If you tell one to create a ridiculous picture of 14 lemmings and a talking cantaloupe wearing a trenchcoat and pretending to be a private investigator, it will do so. Dall-E and its many competitors have taken a huge leap forward, in both their image quality and their ability to translate arbitrary text into images. Such systems are finding their way into advertising, product design, set design, film and other industries. But they are also showing potential as engines of misinformation and disinformation, as they can generate deepfake images of events that never happened or alter images of events that did happen.
This article is part of
What is Gen AI? Generative AI explained
Space synthesis. As is the case with images, this kind of synthesis can occur with 3D spaces and objects, both real and digital. On the real-world side, applications such as Autodesk or Spacemaker can help design buildings and the spaces in them or urban landscapes incorporating built and natural elements. In these situations, AI supplements human designers' work by filling in missing details or proposing solutions to fit specific code requirements or space and material constraints. Many companies -- most notably Meta and all the major game creators -- are also developing applications to generate virtual spaces for game designs. These AI systems can constantly generate new spaces and possibly even make them infinitely expandable.
Real-world examples of GenAI
The use of GenAI is continuing to emerge and expand within organizations. According to a recent Gartner poll of over 1,400 executive leaders, 43% indicated that they are in the process of piloting generative AI products and tools.
The instances that drive the use of GenAI in this setting can vary widely. The Gartner survey indicated that 47% of respondents invested in GenAI for customer-facing functions, such as sales, marketing and customer service. IT functions were also a common area where generative AI investments take place, which includes software development, infrastructure and operations.
Industries experimenting with generative AI tools
Pharmaceuticals. Pharmaceutical companies -- including Amgen, Insilico Medicine and others -- and academic researchers are working with generative AI in areas such as designing proteins for medicines. Predicting the folding of proteins has been an enormous challenge for geneticists and pharmaceutical developers for decades. Deep learning models, such as generative adversarial networks (GANs), are increasing researchers' abilities to understand and use protein synthesis.
Genetics research. Although its emergence in genetics research is not particularly rapid, generative AI is contributing to this field. A common barrier associated with the use of this technology for genetics research is limited access to genetic databases, largely due to privacy concerns. A recent study, however, consisted of training GANs and restricted Boltzmann machines to learn distributions of real genomic datasets. This would then enable them to create artificial genomes.
Manufacturing. In manufacturing, Autodesk, Creo and other products use generative AI to design physical objects. In some cases, they also create those objects through 3D printing or computer-controlled machining and additive manufacturing. Generative AI can create machine parts and subassemblies of larger objects, for example, and can sometimes optimize designs for the following aspects of the manufacturing process: materials efficiency (minimizing waste), simplicity (fewest parts) and speed of production.
Entertainment. ChatGPT, Dall-E and other tools are already employed in generating conceptual art to guide scenario and environment development and are expected to be used to generate full environments in the future. Generative AI tools are also taking up background music generation for games. It's worth noting that artists and corporations are filing a flurry of lawsuits based on copyright infringement and intellectual property theft, arguing that the use of their protected IP in the training data, coupled with the ability to request output in a particular person's style, equate to unfair use and violations of copyright. This kind of legal challenge is slowing the use of generative tools in some contexts.
Cautionary note on applying generative AI
It's important to underscore the downside of generative AI tools. As good as the text outputs are, for example, they still won't always meet the professional standards in the field in question. Professors often can tell immediately that the paper was not written by the student, just as they generally could when students bought papers from term-paper mills -- and instructors themselves can now deploy AI tools to detect papers that are produced by AI. Judges and other lawyers can pick apart briefs cobbled together by generalist AI because they are incorrectly reasoned or inadequately supported.
And so, the tragic flaw (in the Greek tragedy sense) of generative AI systems has come clearly into view: The characteristics that make the current GenAI tools so very powerful in creating real-sounding text and other content also make it possible for them to fabulate. That is, they can generate answers that are at odds with reality. To wit: the social media images of Pope Francis in a big, puffy white coat; Elon Musk hobnobbing with Alexandria Ocasio-Cortez; and Donald Trump being dragged away by police.
Compounding the problem is the ability of the tools to make up supporting materials when pushed to provide support for something they generated. Pushed to support a point in a term paper, for example, a GenAI tool might fabricate a quote in support, for a real person or a person made up for the purpose. When asked to provide legal citations in support of a brief, an AI tool might make up court cases and even entire courts.
While the potential uses for GenAI tools are basically any use case in which a human would generate output in any medium, the tools are not yet ready to do everything well enough -- or cheaply enough -- to be used everywhere. Organizations seeking to implement GenAI tools have to be selective and proceed with significant provisions in mind.
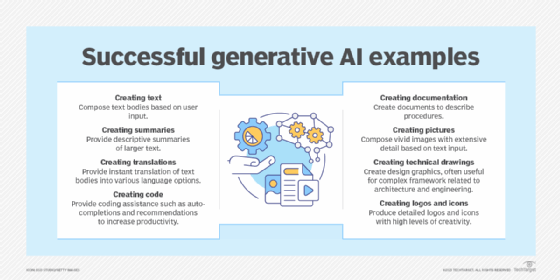
Examples of generative AI tools
With all that in mind, GenAI tools are still hugely useful aids to professionals working with text. ChatGPT is the gold standard for general-purpose text synthesis, though similar tools such as Bloom can do about as well.
In addition to the problems cited above, in this context it's very important to keep in mind that a GenAI tool is perfectly capable of spitting out a block of text that is, in whole or in part, one of its training texts. In some cases, that text will have copyright attached to it, but the GenAI tool will not "know" that -- or, if it knows, will not tell you about it. So, using text that a GenAI tool spits out in any public-facing use case, such as an article for publication or ad copy, without considering this possibility and doing some due diligence is dangerous. And, of course, right now the output of such tools is not itself copyrightable, so publishing it is in effect giving it away as public-domain text.
Creating summaries
Some tools have been trained specifically for summarizing text, sometimes in specific fields. Writing assistance site Grammarly has a general-purpose summarizer, as do Jasper, QuillBot and TLDR This. Tools such as Iris.ai and SciSummary focus on academic papers, LegalMind and Legalyze on legal documents, and other specific publication spaces are seeing similar tools evolve to address them.
Creating translations
Translation is a special case of text-to-text transformation, and Google Translate is perhaps the best known and most widely used tool in the space. People also use Alexa Translations, Bing Translator and Meta's SeamlessM4T, as well as other general-purpose tools like ChatGPT. In areas of specific technical specialization, such as medicine or law, professional translators sometimes employ AI tools to assist them; currently, however, there are few AI tools themselves specialized by discipline.
Creating code
GenAI tools are extremely well suited to dealing with what are called domain-specific languages, which include networking protocols and programming languages. DSLs have strict and small vocabularies, along with well-defined and orderly syntaxes. General-purpose tools like ChatGPT can help; there are many specialized tools too, such as GitHub Copilot, Tabnine, CodeWP and Amazon CodeWhisperer, some of which can plug directly into a coder's chosen integrated development environment.
Creating documentation
Just as they can be used to generate code, AI tools can be used to document code. They can provide explanations of what a procedure does, for example, or what kinds of data an object holds and manipulates. They can sometimes even supply the explanation of what a procedure or module is used for in the framework of a larger program. While these tools might not always provide the level of explanation of an algorithm a human can, they can do an enormous amount of the tedious work of fully documenting a codebase.
Using GenAI to create images
GenAI got a huge and surprisingly positive PR bounce with the advent of ChatGPT. This distracted from -- and even, to some extent, washed away -- the previous round of much more negative commentary centered on AI image creation. Basically, discussion of image GenAI had focused largely on the destructive potential of deepfake images: fictional constructions intended to look like real photos of actual events and people.
The rise of Dall-E and Stable Diffusion around the time of GPT-3.5's release also helped broaden the discussion. Stories and examples focused on whimsy: "Draw me a picture of a yellow dragon on a green motorcycle driving through Brown's Valley, Minnesota." All the negative potentials associated with deepfakes persist, though, and other problems have become apparent as well.
Copyright considerations apply here, too, just as they do with text: Outputs might include protected inputs, and outputs cannot be copyrighted.
Creating pictures
Stable Diffusion, Midjourney and Dall-E -- now Dall-E 2 -- continue to be important. As indications of the breadth of the space, consider also the free photo restoration tool GFPGAN and Lumen5, a commercial product with a free "community" tier, which generates video content rather than still images. Hunting through the spaces at Hugging Face, a community built around free AI models such as Llama and Stable Diffusion, one can find specialized tools for other kinds of image generation or manipulation -- e.g., for comic creation or image upscaling.
Creating technical drawings
Generative design applies GenAI to the problems of computer-aided design and manufacturing (CAD/CAM) by generating design models and images. Generative design has been applied in areas as diverse as architecture and urban planning, civil engineering, mechanical engineering and biomedical device design. Autodesk Fusion 360 and Dassault CATIA Generative Design Engineering, longtime CAD/CAM vendors, are prominent generative design tools.
Creating logos and icons
Any image generator can generate a logo, but there are specialist AIs focused on this activity specifically, including Logo Diffusion, Designs.ai Logomaker and Looka Logo Maker.
Future generative AI examples
Although there's no way to predict which generative AI examples and use cases show the most promise for the future, there are some -- such as image generation and speech synthesis -- that have shown enormous progress in the last few years. Other areas, such as medicine and manufacturing, have also proven enormously promising and show the wide range of fields that AI might contribute to. Progress in physical use cases appears slower, which makes sense given the inherent limits imposed by manipulating matter instead of data.
Such progress builds on itself, a dynamic on full display in 2022 and 2023. As the base tools become cheaper, more widely available and easier to use, the pool of people harnessing those tools broadens. This increases the number and type of situations those tools get trained to deal with, further accelerating the pace of change.
Incorporating generative AI into other AI-powered tool suites can turn them into a more powerful gestalt. For example, current code- and documentation-generation systems aren't great, but as they improve and are combined with other kinds of AI systems already in place -- for detecting coding errors, common security flaws and the use of licensed code in unlicensed ways, for example -- the developer tool set will become more powerful and productive.
What's important to remember is that there are antisocial and dangerous applications of AI that will also become easier in the same ways.