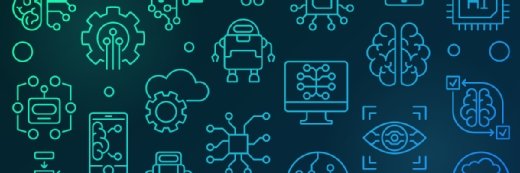
Simplify enterprise AI integration with a centralized AI hub
For enterprises looking to scale their AI projects, centralized AI hubs and governance can simplify integration, streamline operations and ensure consistency.
When transitioning AI proofs of concept or pilot projects into full-scale deployments, enterprises must address an important challenge: effectively integrating AI across business units and technical silos.
Centralized AI hubs offer a pragmatic approach to this challenge, simplifying AI integration while ensuring consistency and improving efficiency. As enterprises scale their AI initiatives, centralized hubs and governance strategies will be pivotal in maintaining consistency while driving innovation.
The challenge of scaling AI deployments
Scaling AI initiatives from pilot projects to real-world applications can be difficult. Any AI deployment will face three main issues: integration complexity, governance consistency and resource management.
Moreover, enterprises that belatedly realize the need for a centralized AI hub might face even more significant challenges in integration and consistency. Without a centralized strategy, attempts to scale AI across the enterprise are likely to fail.
Integration complexity
When different departments run their own pilot projects, the diverse tools and techniques they use might not be compatible or able to communicate easily. Even for organizations with a unified AI stack, data silos can exist between departments, with teams storing data on disparate platforms in varying formats or securing data access in ways that hinder integration. These issues are exacerbated in enterprises running legacy systems with limited interoperability.
Governance consistency
Enterprises, especially those with autonomous business units or international divisions, often struggle to ensure that AI usage adheres to common standards for privacy, regulatory compliance and auditability. Without consistent AI governance, discrepancies in how AI systems handle data can create compliance and security risks.
Resource management
As AI deployments scale, managing resources such as data, models and infrastructure can become cumbersome. The computational demands of AI systems, particularly those involving deep learning, are much higher than those of traditional data analytics programs. This difference not only drives up costs, but also adds complexity when deploying GPUs and other specialized hardware.
Benefits of centralized AI hubs
Centralized AI hubs address scaling challenges by providing a unified platform for AI integration and governance.
For example, in enterprises with disparate data sources or varying data quality across divisions, an AI hub can consolidate data into a single repository prepared specifically for AI use. This unified approach simplifies data management and ensures uniform data quality standards and practices. In addition, data governance policies for regulatory compliance, privacy and security can be centrally managed, fostering a responsible and ethical AI environment.
Similarly, where AI is integrated into business workflows rather than confined to standalone pilot projects, a centralized AI hub can enforce best practices across all use cases, reduce duplication, and enable automation with a single control point for auditing and model retraining. This, in turn, reduces the risk of inconsistent model performance.
Moreover, a well-designed AI hub is built for scalability. As AI projects become more widely adopted, a centralized hub enables organizations to manage increased data volumes, more complex models and higher computational demands.
An additional, albeit less tangible, advantage of a centralized AI hub is improved collaboration and knowledge sharing. The hub provides a platform where employees can easily access and share insights, models and best practices. This shared knowledge base promotes creativity and the formation of cross-functional teams that can work together on AI projects. Centralized AI hubs also facilitate the pooling of skilled AI developers and computational resources, preventing duplication of efforts and redundant infrastructure or licenses.
Examples of centralized AI implementation
Centralized AI hubs have many industry use cases, in fields ranging from manufacturing to financial services to logistics and transportation. Although each sector must consider its specific conditions and circumstances when implementing a centralized AI hub, the common threads are optimization and consistency.
Manufacturing
Modern manufacturing often features a wide variety of equipment, increasingly instrumented with sensors that can collect operational data through the internet of things. AI can be extremely valuable for manufacturing processes such as predictive maintenance, which optimizes schedules for individual machines while also considering the resources the entire facility needs. A centralized AI hub makes this process very efficient, reducing unplanned downtime for machines and ensuring consistent AI application across multiple production facilities.
Financial services
Fraud detection and risk management are critical capabilities in finance, but fraud can be complex, and risk is often spread across business units. A centralized AI hub enables banks and brokerages to standardize risk assessment models while enhancing personalized services by integrating customer data into a unified platform.
Logistics and transportation
By their nature, logistics companies are highly distributed, but often require centralized processes such as real-time route optimization to improve delivery times and reduce fuel consumption. A centralized AI hub can integrate demand forecasting, inventory management and staff scheduling, ensuring better resource allocation and reducing operational costs.
Developing a centralized AI governance strategy
Many organizations can benefit from a centralized AI hub, but also need a robust AI governance strategy to maximize benefits. Every decision made in AI deployment has its consequences, underscoring the need for strong governance.
Focus on the following three key elements:
1. Clear objectives and metrics
Define clear objectives for the AI project in advance. Establish business-focused metrics to measure success, such as operational efficiency, cost savings, risk assessment and customer satisfaction, as well as deployment metrics, such as performance, resource usage and regulatory compliance.
2. Standardized policies and procedures
Develop appropriate standardized policies for data management, model development, deployment and maintenance. Ensure that these policies are compliant with relevant regulatory requirements and industry standards.
3. Cross-functional teams
In a centralized AI hub, there is no natural hierarchy of departments. Instead, collaboration is key, with each team bringing its unique expertise. Establish cross-functional teams to oversee centralized AI governance, including IT, legal and business unit representatives.
Continuously monitor this strategy and adapt it as circumstances change. AI can make a dramatic difference to operations and customer-facing strategies, with more profound implications than adopters might initially expect. As these changes affect the business, update policies alongside models. It's crucial to regularly adjust and add to what has been done, ensuring the AI hub evolves with the business's needs.
Donald Farmer is the principal of TreeHive Strategy, which advises software vendors, enterprises and investors on data and advanced analytics strategy. He has worked on some of the leading data technologies in the market and in award-winning startups. He previously led design and innovation teams at Microsoft and Qlik.