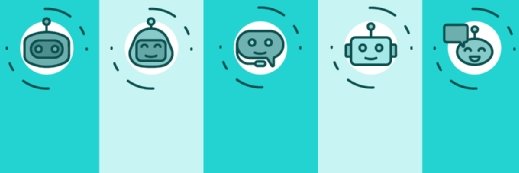
Recent developments show us the future of chatbots
Experts in conversational AI are optimistic about what recent advancements in chatbot technology mean for the future. Despite challenges, these advancements can point the way forward.
In the last five years, chatbots have been widely deployed by enterprises in both customer-facing and employee-facing scenarios to support a range of marketing, customer support and IT use cases. These chatbots come in different flavors: voice-based or text-based and with a web interface or mobile interface. Chatbots have been deployed not just by B2C and B2B enterprises but also by federal, state and local governments and agencies for citizen services.
However, despite high expectations and much promise, chatbots in general have thus far underperformed or delivered mixed results. To be sure, chatbots might have reduced support costs by automating customer support but often at the cost of customer experience (CX). Both enterprises and their customers view current chatbots as less than exemplary.
Chatbots are struggling with context and interactivity
The interactions with many of the chatbots seem stilted or they seem to be following a strict rules-based flow. The chatbots can understand only simple instructions and struggle with complex requests and long questions. To compensate, the developers only allow for a limited set of interactions and chatbots often must route the interactions to a human.
Further, chatbots have been limited in their ability to maintain context of the interaction and this constrains their usage to short requests because long sequences of questions could not be processed effectively. In short, despite advances, the current generation of chatbots struggles with understanding user intent and retaining context.
Broadly speaking, to be useful, a chatbot must be good along two dimensions. First, it must understand the user intent and second, provide the needed information in the form of a resolution based on user intent. Large language models (LLMs) like GPT-3 are trained on vast amounts of text (several orders of magnitude larger than a typical chatbot) and generate text/content based on user prompts. ChatGPT is the most recently hyped LLM tailored for dialogue-based interactions.
LLMs might hold key to chatbot success
In the span of a few weeks, ChatGPT has generated a lot of buzz and represents a significant improvement over the current state. Despite the impressive examples doing the rounds on social media and the web, a closer scrutiny reveals several limitations. In its current avatar, ChatGPT generates glib text that might not be true, does not provide sources/references and can generate potentially offensive or politically incorrect responses. Even the OpenAI team has said that reliance on ChatGPT for factual queries is not such a good idea.
But even among all the "hallucinations" of ChatGPT, one thing stands out: It excels at understanding user intent, maintaining context and keeping up with dialogue while remaining highly interactive.
Now, imagine the following scenario for enterprise chatbots:
- a customer interacts with a chatbot via your website or app;
- the chatbot uses an LLM to understand the customer's question and determine the appropriate response;
- if the customer's question requires querying enterprise systems (e.g., to check the status of an order), the chatbot sends a request to a custom back-end system;
- the back-end system queries the appropriate enterprise systems and retrieves the necessary information;
- the back-end system formats the information and sends it back to the chatbot;
- the chatbot uses the LLM to generate a response to the customer based on the information it received from the back-end system; and
- the chatbot presents the response to the customer in a friendly, interactive way.
In essence, this represents a best-of-breed strategy that combines the strengths of both traditional chatbots and LLM-based tools. While the traditional chatbots are good at integrating with enterprise systems after the user intent has been figured out, the user interaction can be driven by LLM-based tools. By making selective use of LLM capabilities, it avoids challenges with their content veracity and overcomes concerns with LLMs, such as generating offensive text.
LLM technology a strong candidate for many enterprise use cases
As we can see from these examples, it can have different conversational styles and content tones, allowing many possibilities for presenting content to users in a more engaging way. The content can even be customized based on an enterprise style guide or brand personality. It can be personalized based on customer preferences or customer segments. In short, pretrained LLMs can be fine-tuned based on enterprise-specific data and this presents opportunities to elevate CX when using the strengths of LLM technology.
There are several other potential applications of this technology. Such chatbots can provide more engaging and personalized scripts during live interactions by customer support agents. Employee chatbots can also provide useful summaries of documents and knowledge bases, instead of just providing a link to them. It's even possible that the same chatbot can seamlessly support multiple languages, allow for language switching within a conversation or provide translations -- sometimes even in the same interaction.
In 2023, the world of enterprise chatbots will continue incorporating the relevant state-of-the-art user intent understanding and dialogue management capabilities that we've seen play out recently. This represents a leap forward for conversational AI.