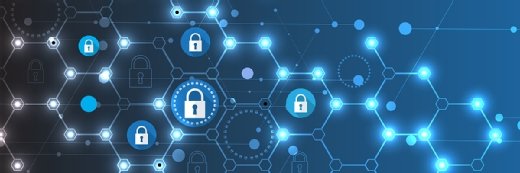
kras99 - stock.adobe.com
How to manage proprietary enterprise data in AI deployments
Explore strategies for managing sensitive data in enterprise AI deployments, from establishing clear data governance to securing tools and building a responsible AI culture.
Across industries, teams and individual workers are adopting AI technologies, particularly generative AI. Although some use these tools strategically, with careful attention to administration and IT governance, many instead use them as self-service utilities for tasks such as content generation, coding, data analysis or design.
In the latter scenario, data governance is often a secondary consideration. As a result, the rapid adoption of AI has established the conditions for both innovation and new data security risks, especially when proprietary enterprise data is shared with generative AI systems. To effectively integrate AI into their daily work, users must balance a desire for innovation with the need for security and confidentiality.
The value and risk of proprietary data
Some proprietary data sets are clearly commercially sensitive: customer records, finance and strategy documents, product designs and specifications. But other types of data have value too:
- Task-specific data. In business, AI is often used for process mining -- analyzing patterns in existing behavior and then identifying ways to improve performance. For example, a business might train the AI on examples of completed but hard-to-perform tasks, such as order-to-cash workflows or supply chain optimizations. This data, which captures what does and doesn't work in business processes, might be a real competitive differentiator in industries such as manufacturing, healthcare or financial services. Consequently, it should be treated as confidential.
- Nonpublic trends. In domains like financial services, proprietary data often includes complex, high-value predictions, such as those used for managing fraud, credit or risk. Even in retail, companies often closely guard information about consumer trends or supply chain insights.
Where generative AI fits in
Generative AI models are highly effective at analyzing and learning from this type of data, but if they run on a vendor's cloud service, the training process risks exposing that information, even if inadvertently.
These risks arise from common characteristics of AI systems:
- Data retention. Generative AI models are typically trained and retrained on large data sets -- including user input, which might contain proprietary information. If that data becomes part of the model's knowledge base during training, it could reappear in future outputs when the model responds to users' prompts. Ideally, the AI system should process sensitive information only once, without storing it for future use.
- Pattern recognition and reproduction. Generative AI models, such as chatbots and image generators, work by recognizing patterns and producing content that resembles those previously learned sequences. As a result, generative models such as chatbots can inadvertently expose proprietary data by reproducing similar patterns, even if they do not explicitly repeat the original input data.
- Data storage practices. To improve their models, many AI providers store user interactions, such as conversations or prompts, and use them to retrain and update the models. This behavior is often the default unless a user explicitly opts out.
- No selective deletion. Once data is shared with a generative AI system, it cannot be undone -- the model cannot "unlearn" the information. As a result, sensitive data, or patterns derived from it, might persist even if a user later realizes the data should have been protected.
- Data harvesting. Third parties can sometimes infer proprietary information and even train their own AI models using other models' outputs; a generative AI system's users can gain small amounts of insight from what that model produces, especially in the case of an automated system or a chatbot. When extracted, that data can serve as training data for another model. For example, a bank might use a third-party AI tool to assess credit risk and then gradually reverse-engineer insights from collected risk scores, in effect developing its own model from a vendor's application.
Mitigating risks and protecting data
Organizations need an array of strategies to manage how company data is used in AI deployments, encompassing both general data management best practices and AI-specific measures.
As with any data management effort, establish and communicate clear policies. What types of data can be used with AI tools? What permissions do users need? How will administrators audit usage? In general, don't let AI adoption displace traditional data management best practices, but rather extend or adapt existing processes for these new use cases.
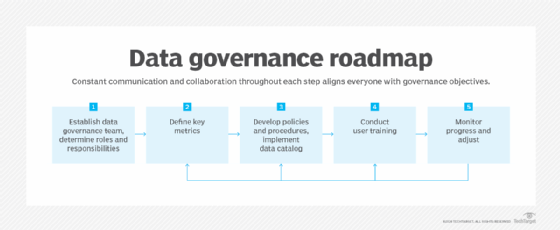
One restrictive approach is to apply strict data controls, limiting AI access to authorized personnel. However, many users adopt AI assistants casually, without IT's sanction. And with AI increasingly built into enterprise and desktop applications, strict controls might only address a few use cases.
Wherever possible, use enterprise-grade AI applications with strong security and compliance features. Configure them to use minimal company data, and disable data retention wherever possible. Many AI chatbots and tools have settings to prevent conversation history from being saved, reducing the risk of proprietary data retention. If IT can't automate those settings, ensure users are aware that they must activate them manually.
In addition, when sharing specific data with AI systems, it is best practice to anonymize and remove identifying information. It's also important to monitor how the AI is used and what data is shared. For highly sensitive use cases, consider deploying AI models on private infrastructure rather than in a public cloud.
People problems
Although technical considerations often take center stage in AI discussions, the human element matters too.
With generative AI tools, most user interactions take place in the form of prompts and conversations. Safeguarding proprietary data therefore requires not only technological solutions, but also a risk-aware organizational culture backed by clear user guidelines.
Implement training that covers practical scenarios and the latest AI technologies to ensure employees handle data responsibly. This training should emphasize the importance of data security, privacy and AI risks, including nonsecurity issues like hallucinations and bias. It helps, of course, to have some experience with AI systems when developing these training programs; organizations new to AI might need to contract with external training providers.
Establish guidelines for responsible AI use, tackling topics such as bias awareness, data minimization and privacy by design. These guidelines might feel restrictive for users accustomed to self-service tools, such as data visualization applications or business intelligence. But it's important that these users understand they are sharing data with third parties that can use that data for their own purposes. As AI becomes increasingly integrated into the company -- and industry -- these guidelines will be an established part of onboarding and best practices, so they should be clear and concise.
Finally, leadership plays a key role in fostering a culture of responsible AI use. Executives can reinforce support for responsible AI and data protection as part of the organization's ethos and culture, and should complete at least some training on AI's strategic implications and risks. Their commitment to security and data protection ensures these issues remain a priority throughout the organization.
Organizations that fail to develop data protection strategies for AI are likely to find themselves at a competitive disadvantage and face substantial risk. But by making thoughtful choices about enterprise AI technologies, practicing careful data governance and building a culture of responsible use, businesses can enjoy AI's innovative advantages while minimizing the risk to their sensitive data.
Donald Farmer is the principal of TreeHive Strategy, which advises software vendors, enterprises and investors on data and advanced analytics strategy. He has worked on some of the leading data technologies in the market and in award-winning startups. He previously led design and innovation teams at Microsoft and Qlik.