How to effectively manage AI projects in 12 steps
AI is a high priority for companies but results often fall short of expectations. These 12 steps will help you successfully manage AI projects and deliver business value.
Executives today enthusiastically embrace artificial intelligence, with the vast majority saying AI is a top priority for their organizations.
Multiple CEO surveys confirm this assessment. For example, a report from Boston Consulting Group, "From Potential to Profit with GenAI," found that 89% of executives ranked AI and generative AI as a Top 3 tech priority for 2024.
Yet most companies are struggling to turn their AI projects into wins. The same BCG report found that "66% of leaders are ambivalent or dissatisfied with their progress on AI and GenAI."
Meanwhile, a report from nonprofit research institute RAND on root causes of failure in AI projects stated that an estimated 80% of AI projects fail -- a failure rate that's double that of conventional IT projects.
AI project failures might mean the investment in the tech exceeded actual returns, the AI tool had a higher rate of inaccuracies than the organization can tolerate, or the tool sits unused by workers either because they don't know how to use it or they don't trust it, said Kathleen Walch, global head and general manager of Project Management Institute (PMI) Cognilytica, which provides AI and data best practices training and certification.
This article is part of
What is enterprise AI? A complete guide for businesses
Whatever the reasons behind the failures, that high failure rate has created disillusionment with AI, particularly generative AI, as executives are finding that the AI hype isn't living up to their expectations.
Moreover, Walch said, those high failure rates indicate a need for organizations to fine-tune their strategies for managing AI projects to ensure success.
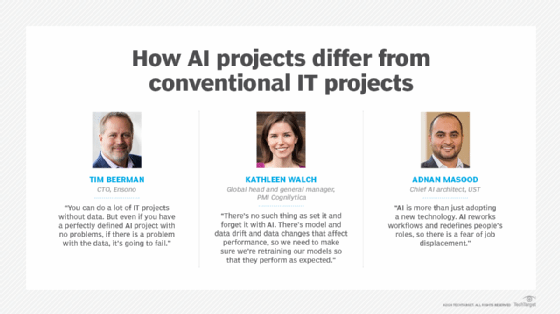
How are AI projects different from traditional projects?
AI projects have many similarities to other IT initiatives. Primarily, they both use a technology to improve existing business capabilities, functions or processes or enable entirely new ones.
However, an AI project is different from projects that feature conventional technologies, and those differences stem from AI's unique characteristics.
- Complex. To start, AI projects involve not just the AI algorithms but also other tech components. That typically adds more complexity than many other traditional tech implementations, said Christine Livingston, global AI leader with management consultancy Protiviti. "It's not just AI that delivers value; it's AI working in concert with other technologies. It's AI plus other technologies that will bring the solution to life," she explained.
- Data-centric. AI projects also are data projects -- another characteristic that sets them apart from many, though not all, traditional projects, said Tim Beerman, CTO of Ensono, an IT consultancy. "You can do a lot of IT projects without data. But even if you have a perfectly defined AI project with no problems, if there is a problem with the data, it's going to fail," he said.
- Iterative. Another difference: AI projects require continuous monitoring for accuracy with ongoing training, testing and tuning. AI projects are iterative in nature, which is not the case in many other technology implementations, Livingston said.
- Impactful. As significant as those differences may be, the most significant difference between AI and more conventional tech projects is in the potential impact that the AI initiatives can have, Beerman said. "With IT projects, we often aim to make things more efficient. But with AI, you can approach a problem completely differently," he said. In other words, traditional projects typically focus on improving a process, whereas AI can blow up the process entirely.
Challenges of AI project management
As is the case with any initiative, AI projects typically come with a host of challenges. Although each project will have a unique set of obstacles, many AI projects face these common issues:
- Inflated and unrealistic expectations. "People have their own preconceived notions and expectations about AI, and oftentimes they're very inflated," Livingston says. Too often, people think they can "just throw AI at a problem and it will all work out."
- Not enough quality data. To operate accurately, AI needs to be trained on enough quality data. The volume and quality of data required depends on how the AI will be applied, explained Adnan Masood, chief AI architect at UST, a digital transformation solutions company. For example, high-risk AI systems, where the systems impact life or safety, require extremely high amounts of high-quality data to ensure the highest levels of accuracy. AI used for less impactful reasons has lower thresholds for data quality and quantity because there's more tolerance for inaccuracies.
- AI implementations at scale. Organizations can quickly roll out an AI use case prototype, especially a GenAI prototype, because such proof of concepts are small and easily managed, Livingston said. But as organizations apply those new AI tools at scale, they often struggle with building the required infrastructure as well as monitoring large-scale AI deployments for accuracy, unintended biases and ethical use. They also struggle to control infrastructure-related costs, particularly their spending on cloud computing. "They can get into hundreds of thousands [of dollars] in extra cloud spend if they're not thinking through a strategy for doing this at scale," Livingston added. Research confirms the observation. For example, Gartner has predicted that 30% of GenAI projects will be abandoned after proof of concept by the end of 2025.
- No project end date. "There's no such thing as set it and forget it with AI," Walch said. "There's model and data drift and data changes that affect performance, so we need to make sure we're retraining our models so that they perform as expected."
- User adoption. Many organizations struggle to get users to adopt, let alone embrace, the use of a new technology to complete tasks. But they can encounter even greater hurdles when implementing AI because "it's more than just adopting a new technology," Masood said. "AI reworks workflows and redefines people's roles, so there is a fear of job displacement."
- Lack of available skills. Some 65% of respondents surveyed by software company Confluent for its "2024 Data Streaming Report" cited a lack of AI-related skills as a major issue or frequent challenge.
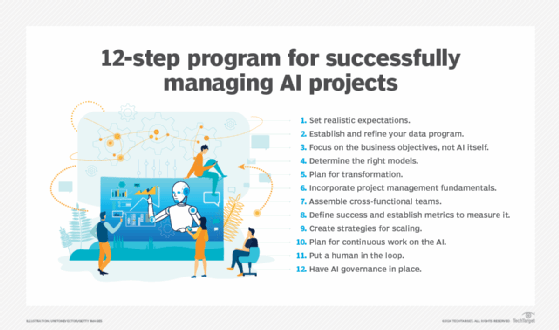
12 steps to effectively manage AI projects
Although there has been much interest in using AI to aid project management, project managers and AI experts have stressed the need to bring tried-and-true project management tools and best practices as well as AI-specific strategies to AI initiatives. They include the following 15 steps.
1. Set realistic expectations
Project leaders should start with understanding "what AI can and can't do," Livingston said. They then should educate other stakeholders so that they have realistic expectations on what benefits AI can deliver and how it does so for their organizations as well as what kind of resources and time is required to deliver those returns.
2. Establish and refine your data program
Many organizations have yet to create a mature data program that has the right infrastructure, practices, processes and governance to ensure that the right data in the right amounts at the right quality level is available at the right time. Some 35% of respondents to S&P Global Market Intelligence's "2024 Global AI Trends Report" cited storage and data management as their primary infrastructure issues hindering AI deployments.
Given that AI doesn't work at all without enough quality data, Walch said she advises project leaders to "make sure you understand your data requirements, including data sources, how to access that data and the state of that data."
3. Focus on the business objectives, not the AI itself
The hype around AI has had some executives focusing more on the technology than on what the technology allows them to do, Masood said. He cautioned against falling into that trap, saying that organizations instead must start by identifying either the problems to be solved or the opportunities to be pursued and then determining whether and how AI will address them.
"Approach it from a business perspective. Think [about]where [you are] inefficient or where … you can do something better. That's the opportunity gap that AI will improve," Masood advised. In other words, be sure that the goals of the AI project align with the organization's objectives.
4. Determine the right models
The term AI often encompasses all types of intelligent technologies, including classical artificial intelligence, GenAI, machine learning, deep learning, neural networks and more. While many might use AI as shorthand for any and all of these, the various types of AI are not identical nor interchangeable.
AI project leaders must determine which approach is best suited to solve for the business need that's being addressed and consider where the AI model is being used -- for example, on the edge versus in the cloud -- Walch said. "That matters for making sure it works as expected," she added.
Luv Tulsidas, author of Failing Fast?: The 10 Secrets to Succeed Faster (Innovation Done Right) and founder of Techolution, which builds AI solutions for enterprises, said most organizations should opt for a specific-purpose AI that is ready to address their specific business needs and only requires fine-tuning. He said this is better than trying to take a general-purpose AI and craft it to their own needs -- something that will be "a long, expensive journey that requires a lot of skills and where the probability of success is still very low."
5. Plan for transformation
As Beerman noted, AI is a transformative technology, not simply an efficiency enabler. As a result, he said organizations should enable stakeholders and project team members with the skills and resources they need to identify opportunities to fully transform processes and products.
Organizations also need to ready workers for AI-enabled workplaces, where there are not just changes in workflows but entirely new ones that require workers to have new skills and perform new tasks.
"It's not just change management. It's restructuring. And you need to train associates to use these AI tools, to act differently, to change their processes," Beerman said. "I don't think AI project leaders spend enough time on enabling the end users to think differently because they [incorrectly] treat AI like any other technology."
6. Incorporate project management fundamentals
Although AI initiatives do differ in some ways from those involving only conventional IT, AI projects still benefit from traditional project management best practices, Livingston said. "All the things we've learned over the decades of technical project management still apply," she said, noting that Agile delivery principles typical fit well with AI projects, whereas the Waterfall delivery approach does not.
7. Assemble cross-functional teams
Beerman cited the need for cross-functional collaboration as particularly important for AI project success. Project data, business, technical and other stakeholders must collaborate to ensure all components required for the AI initiative are included.
8. Define success and establish metrics to measure it
AI projects, like all other kinds of projects, should include what organizations deem as a successful outcome as well as the metrics and KPIs to measure AI success, Walch said. She acknowledged that defining success for an AI project is often more challenging than it is for other types of projects due to AI's nature. For example, a probabilistic AI model will never be 100% accurate, so the organization needs to determine how accurate the model should be with each specific use case before it can be declared a win.
Livingston acknowledged that setting metrics to measure success for some AI projects, particularly those involving GenAI, might be challenging because of the newness of the use cases. To help with such challenges, Masood said he advises executives to focus on the business objectives tied to each AI project and develop metrics tied to the business case.
9. Create strategies for scaling
Given the challenges that organizations have with scaling AI, Livingston said AI projects must plan for how they'll move from proof of concept to pilot to deployment and then expanded use of the AI tool, identifying and funding any new IT architecture, data infrastructure and talent that will be needed to support AI at scale.
10. Plan for continuous work on the AI
According to Masood, AI projects require "bold strokes and long marches." As such, organizations need to think of AI projects more like products that require iterative support improvements. More specifically, AI requires ongoing monitoring to ensure it operates within the performance parameters set by the organization. It also requires ongoing training with new data as that data becomes available. It needs users who are continuously learning how to work with the AI and use it to transform.
Additionally, Walch said project leaders must work with executive stakeholders to ensure they allocate the resources required to make all that happen.
11. Put a human in the loop
The word "intelligence" in its name notwithstanding, AI still needs human oversight throughout its lifecycle, from its development and training to its operational performance and fine-tuning, Tulsidas said. As such, AI project plans must include a human in the loop -- more importantly, Tulsidas stressed, the right human with the right expertise and skills. "Without that, there will be no success with AI solutions," he added.
12. Have AI governance in place
AI governance is a framework that sets the ethical and legal standards for the intelligent systems used within an organization. "A framework for ethical and responsible use of AI is one of the best practice items you must have," Masood said.
The case for an AI factory
A new term, AI factory, has bubbled up as AI has gone mainstream. Definitions vary, but it's essentially a framework and function within the enterprise that embodies processes that can be applied to effectively execute AI projects, Masood said.
Masood said applying a well-articulated approach to AI project management is foundational to such frameworks.
Frameworks are valuable, he added, because they bring repeatable processes, particularly around data, to AI project development and execution within the enterprise. That allows organizations to more effectively, efficiently and consistently pursue as well as scale their AI strategies.
Mary K. Pratt is an award-winning freelance journalist with a focus on covering enterprise IT and cybersecurity management.