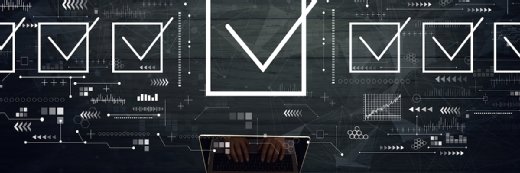
Getty Images/iStockphoto
How to audit AI systems for transparency and compliance
AI audits help businesses ensure functionality, transparency and compliance when deploying AI systems. Learn how to conduct audits to build trust and meet regulatory requirements.
AI audits are an essential part of the AI strategy for any organization, whether an end-user business or an AI vendor.
Drawing on both traditional auditing methodologies and AI governance frameworks, AI audits make sure that these systems meet standards for functionality, transparency, ethics and compliance. While there's no one-size-fits-all approach, best practices like defining scope, conducting thorough assessments and documenting findings can set an organization up for success.
What is an AI audit?
An AI audit -- sometimes referred to as a bias or algorithm audit -- is a structured process for evaluating aspects of an AI system, including its design, algorithms, data, development and operation. The goal is to confirm that the system satisfies performance criteria and complies with regulatory requirements.
An AI audit is a holistic assessment. For example, AI bias stems not only from training data, but also from design choices, algorithmic architectures, system development and deployment practices, and error handling and safety mechanisms. By looking at every aspect of an AI system, audits can identify and mitigate such risks.
The key objectives in AI audits are to verify that the system exhibits the following traits:
- Functionality. The AI system is accurate and reliable for its intended tasks.
- Transparency. There is sufficient understanding of how the system works, including its algorithms, inputs and outputs. This is often evaluated through interpretability or explainability.
- Ethical behavior. The system minimizes bias, harm and discriminatory outcomes for individuals or groups.
- Compliance. The AI system adheres to any applicable standards and regulations.
Why are AI audits conducted?
Organizations conduct AI audits in a variety of contexts:
- Predeployment, to identify and fix issues before systems go to production.
- Postdeployment, to monitor real-world performance.
- As part of operational reviews or as mandated by law.
- As part of root cause analysis following an incident.
- As part of due diligence during mergers and acquisitions.
- By an insurance firm when underwriting risk.
The audit's scope and criteria are tailored to its purpose.
AI audit methodologies and frameworks
AI audit methodologies draw from established auditing practices across technology, risk management and security. Examples include the following:
- Control Objectives for Information and Related Technologies, or COBIT.
- COSO Enterprise Risk Management Framework.
- Institute of Internal Auditors standards.
- NIST frameworks.
- U.S. Government Accountability Office guidelines.
Specialized frameworks and tools are also available for compliance with specific regulations. For example, Oxford University's CapAI tool assesses AI systems in accordance with the European Union AI Act.
AI audits and governance
AI audits are integral to AI governance, including compliance with emerging regulations. For instance, the newly enacted EU AI Act mandates audits as a nonoptional element.
In the U.S., regulations for AI systems exist at local, state and federal levels. Some regulations, such as New York City Local Law 144 on the use of AI in employment matters, require an independent third-party audit. Other regulations that affect AI systems include the following -- though note that federal guidance could change under the Trump administration:
- Colorado SB21-169 and SB24-205, focused on preventing algorithmic discrimination in insurance and consumer protection.
- The U.S. Department of Justice's Evaluation of Corporate Compliance Programs, which incorporates guidance on AI risk.
- The Equal Employment Opportunity Commission's initiative to ensure AI use in hiring is compliant with federal civil rights laws.
- The Federal Trade Commission's rules on AI and consumer harm.
AI audits can also assess whether organizations follow responsible AI practices, adopt explainable AI methods, implement AI governance tools, and adhere to machine learning operations and security best practices. Voluntary AI risk management standards, such as the NIST AI Risk Management Framework and ISO/IEC 42001, provide additional guidance for these types of audits.
Audits for generative AI or large language models involve additional considerations. These audits require assessing potential intellectual property rights concerns, managing hallucinations, making disclosures about AI-generated content, and ensuring data privacy and security.
Internal AI audits
Businesses can manage AI audits by establishing an internal AI auditor or audit team. This team should collaborate with AI, IT, risk, legal and business units to identify and address shortcomings in AI systems. The role of an internal AI audit team is comparable to that of a finance department's internal auditors, who conduct in-house reviews and help their organization prepare for external audits.
AI vendors also benefit from completing internal AI audits for their products, as it demonstrates a commitment to responsible AI practices. Increasingly, such audit reports are requested by both public sector agencies and private companies when procuring AI products.
7 key steps for conducting an AI audit
These seven steps can help businesses get started with an AI audit:
- Define the audit's scope. Establish the audit's main purpose, the AI systems or applications to evaluate, and the risks and impacts to be assessed.
- Gather documentation. Collect detailed information about the AI system's functionality, intended use, and internal and external users.
- Assess data quality and preprocessing. Identify the sources and provenance of training data, including the right to use that data to train AI models. Check for privacy violations and analyze whether the data composition could lead to biases and errors.
- Evaluate development and deployment processes. Examine the types of algorithms used and their transparency. Assess whether protected categories such as gender or race are being used indirectly via correlated variables, and determine whether standard processes for deployment and postdeployment performance monitoring are in place.
- Analyze user impact. Check whether there have been any user complaints and confirm that end users are being informed about the organization's AI use. Depending on the use case, check for bias based on relevant fairness metrics, and test data privacy and application security.
- Check compliance. Applicable regulations vary by industry, country and AI system, so follow regulation-specific guidance and use tailored checklists where applicable.
- Document findings and develop an action plan. Create a report detailing issues discovered, supporting evidence and recommendations. Discuss the findings with key stakeholders and develop roadmaps for improvement, led by the internal auditor or audit team if applicable.
Kashyap Kompella is an industry analyst, author, educator and AI adviser to leading companies and startups across the U.S., Europe and the Asia-Pacific region. Currently, he is the CEO of RPA2AI Research, a global technology industry analyst firm.