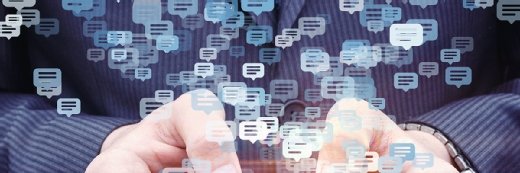
Getty Images/iStockphoto
How AI personalization creates customized user experiences
Businesses can drive revenue and boost user engagement with AI personalization techniques -- if they also ensure transparency and data privacy.
Businesses are no longer designing content for the average customer. Instead, they can customize content for individuals with unique habits, motivations and contexts.
AI personalization is the capability to customize products, services or experiences to the needs and preferences of individual users. Using AI and data analytics, businesses can create personalized experiences like targeted ads in e-commerce or recommendation engines on streaming platforms.
Personalization reduces wasted resources by focusing on the highest-impact content for each user. It also increases user engagement; if customers see recommendations that match their tastes, they're more likely to spend time on a platform, recommend it to others and develop loyalty. However, the practice requires careful engineering, continuous monitoring and ethical data use.
AI personalization techniques
The technologies behind AI personalization include collaborative filtering, reinforcement learning and deep learning algorithms that identify user patterns at scale.
An AI model tracks user clicks, feedback forms and dwell times, which quantify how long a user stays on a page. This data helps build profiles of user preferences and behaviors. The AI model then applies collaborative filtering and predictive analytics algorithms to predict what's most relevant to a user. That might be as simple as showing a user their favorite brand of sneakers when they visit an online store or as complex as predicting their emotional state and adjusting content accordingly.
Personalized AI has many forms, including recommendation engines, personalized pricing, chatbots and predictive personalization.
Recommendation engines
Recommendation engines use collaborative filtering, which looks at behavior patterns across many users with similar interests or profiles. For example, if a user liked items A and B, and a large group of similar users also liked item C, then the system recommends item C to the user. More advanced methods incorporate nuanced information such as holiday periods or time of day.
Personalized pricing
In personalized pricing, predictive models can gauge a user's willingness to pay based on past purchases, browsing history or other characteristics. Dynamic pricing, a similar pricing model that adjusts based on broader market factors, can also help moderate user purchases. For example, Uber uses dynamic pricing to change ride prices based on location, time of day and demand.
Personalized pricing models are sometimes controversial because consumers might pay different prices for the same product. Transparency is crucial in personalized pricing: People often feel uncomfortable if they realize they're being charged differently for reasons they don't understand.
Chatbots
Personalized AI chatbots built on large language models (LLMs) can provide conversational customer support or product guidance. They can also remember user context: maybe a user prefers a succinct explanation or has previously asked about specific products. A personalized chatbot can adapt its tone, length and content to those preferences.
Predictive personalization
Predictive personalization models react to user behaviors and anticipate needs. For instance, an airline application could prompt a user about flight check-in earlier than usual if they historically forget or delay check-in. Or a streaming service might queue up a new documentary that aligns with a user's past interests before they search for something to watch.
Balancing personalized experiences with data ethics
Many forms of AI personalization require extensive user data, which brings up the crucial need for privacy and consent.
Users can feel uncomfortable when they know businesses are tracking or profiling them. The possibility of AI bias also means algorithms could inadvertently stereotype certain groups, making personalization feel exclusionary rather than inclusive.
These best practices can help safeguard data for AI personalization:
- Handle any user data for personalized experiences with consent and store it securely.
- Carefully curate training data to avoid bias and facilitate fair personalization.
- Explain how models use customer data to create recommendations. This helps users develop trust, provide better feedback and make informed decisions.
- Consider regulations that dictate how to store and use personal data. Laws regarding data privacy, like the GDPR in Europe or the CCPA in California, can serve as guardrails to guide ethical design.
AI personalization best practices
AI personalization isn't effective unless it addresses users' distinct needs. To get started, follow these best practices:
- Define user segments. Recognize that not all users want or need the same level of personalization.
- Assemble data infrastructure. To collect and process user data responsibly, define data infrastructure parameters.
- Develop a transparent data collection policy. Make sure people know what data is collected, how it's used and where it's stored.
- Prototype. Create a minimal viable personalization feature, such as a simple recommender system, and test it with a small user group.
- Gather computational resources. LLMs or advanced recommendation systems need computational resources to handle potentially large user bases. Plan for scalability.
- Gather feedback. Try small pilots, gather user feedback and be ready to pivot personalization strategies based on real-world reactions.
- Iterate. Personalization systems degrade over time if the data processing system does not keep up with newly collected information. Regularly retrain models and incorporate user feedback loops.
AI personalization can also create echo chambers where people see only what they already like and aren't shown new products or experiences. Balance tailored content with novel recommendations to ensure personalized AI doesn't narrow users' engagement with the business.
AI personalization industry verticals
Many industries use personalized AI to help tailor services and engage with customers or users. AI personalization has use cases in healthcare, education, travel and financial services.
Healthcare
In healthcare, personalized AI might involve AI systems that look at medical histories, lifestyle data and genetic information to offer treatment suggestions or diagnostics tailored to patients. For example, a virtual health assistant trained on medical information can give guidance about preventive care and lifestyle changes, such as meal plans and exercise routines that suit the patient's schedule and personal tastes.
Education
Adaptive learning platforms can deliver reading materials and practice tests tailored to students' difficulty levels. Platforms might also adapt to learning styles; for instance, a platform could offer visual experiences for some students and textual explanations for others.
Travel
In travel, AI models can create personalized itineraries that combine details like user preferences, real-time flight data and weather forecasts. For instance, if a user usually books budget accommodations and enjoys outdoor activities, the AI model might recommend low-cost flights and scenic hiking destinations.
Personalized concierge-like chatbots that respond to inquiries about flight changes or local recommendations are also increasingly sophisticated. They use natural language processing to parse user queries and respond with human-like dialogue.
Financial services
Personalized AI tools can offer targeted financial products based on customer profiles. By learning unique user habits, personalized AI tools can also detect unusual spending patterns to prevent fraud.
Donald Farmer is a data strategist with 30-plus years of experience, including as a product team leader at Microsoft and Qlik. He advises global clients on data, analytics, AI and innovation strategy, with expertise spanning from tech giants to startups.