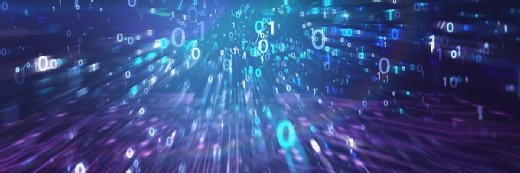
Getty Images
Beyond algorithms: The rise of data-centric AI
Prioritizing data curation, preparation and engineering -- rather than tweaking model architecture -- could significantly improve AI systems' reliability and trustworthiness.
AI is becoming increasingly prevalent in daily life, powering applications from playful voice assistants to medical diagnostic tools. The technology press shows a striking interest in the latest models and algorithms. But what if the key to unlocking AI's potential lies not in the algorithms, but in the data?
In industries that have already adopted AI, a quiet shift in focus is underway: the rise of data-centric AI. This approach sidesteps the assumption that bigger models and better algorithms are the primary drivers of AI progress.
Instead, data-centric AI emphasizes that the quality and relevance of data used to train models is equally if not more important than model architecture. This emphasis on data significantly affects how models are developed, deployed and governed, aiming to make AI more resilient, customizable and widely applicable.
The role of algorithms vs. data in AI
For the past decade, the dominant paradigm in AI has been algorithm-centric, assuming that developing more sophisticated algorithms and models is the key to improving AI systems. While this strategy has led to remarkable breakthroughs -- from GPT-4's language generation and Midjourney's image generation to AlphaFold's protein structure prediction -- its focus on model complexity has overshadowed the critical role of data.
In contrast, data-centric AI recognizes that the performance and reliability of AI systems depend fundamentally on the quality and relevance of the data those systems are trained on. As AI pioneer and computer scientist Andrew Ng put it, data-centric AI is "the discipline of systematically engineering the data needed to build a successful AI system."
This means carefully curating, labeling and structuring training data to ensure it is consistent, unbiased and representative of real-world use cases. Data-centric AI initiatives shift priorities and resource allocation in model development. Instead of concentrating all resources on algorithm development, data-centric AI invests heavily in data engineering, annotation and management.
An important implication of this methodology is that it requires a multidisciplinary team of domain experts, data scientists and AI engineers who collaborate to create high-quality, application-specific data sets. It might also necessitate new tools and platforms for data versioning, validation and monitoring.
Nevertheless, a data-centric approach is critical for domains like healthcare and manufacturing, where data is often limited, messy and expensive to collect. In these contexts, smaller amounts of carefully curated data can yield better results than larger, noisier data sets. By prioritizing data quality over quantity, data-centric AI promises to make AI more practical and effective for a broader range of industries and applications.
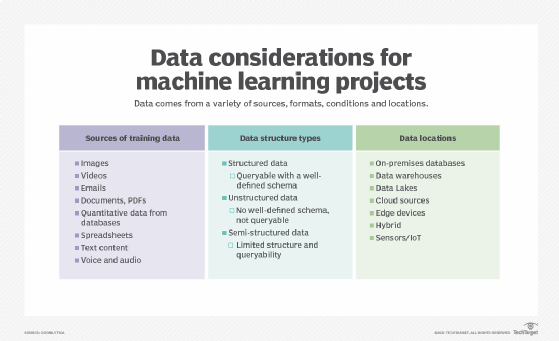
Data-centric AI in practice
As Ng's definition suggests, data-centric AI requires a disciplined and systematic data management and engineering approach. This broader discipline involves several critical practices: data curation, labeling, versioning and validation.
Data curation
Curation is the process of identifying, selecting, cleaning and organizing data to ensure it is relevant, accurate and usable for a specific business purpose. This involves defining clear criteria for data quality, relevance and representativeness, and then systematically filtering, enriching and structuring data from various sources to create a high-quality data set. Effective data curation requires close collaboration among domain experts, data engineers and business stakeholders to ensure the curated data aligns with business goals and use cases.
Data labeling
Labels annotate raw data with meaningful descriptions to teach machine learning models how to make accurate predictions or decisions -- for example, labeling images with object categories, text with sentiment tags or customer feedback with issue types. Correct and consistent data labeling is critical for training effective AI models, but it can be time-consuming and expensive, often requiring human domain experts. Defining clear guidelines, using techniques like consensus labeling and investing in tools to streamline the process can significantly improve label quality and efficiency.
Data versioning
Data versioning involves tracking and managing changes to data sets over time, much like version control for code. It involves systematically cataloging different versions of a data set and metadata about what changed, who made the changes and why they were made. Data versioning enables teams to collaborate effectively on evolving data sets and track model performance across different data versions, including the ability to roll back to previous versions as required. This approach provides transparency and reproducibility, which are essential for building trust in AI systems and debugging data drift or quality issues.
Data validation
Continually assessing and monitoring data quality, consistency and relevance helps train and evaluate AI models. This includes defining and measuring key data quality metrics -- such as accuracy, completeness, timeliness and representativeness -- and setting acceptable thresholds for each metric. Regular data validation helps identify and mitigate data drift, biases or errors that can degrade model performance over time.
By curating high-quality, diverse data, models can learn more predictive and generalizable features. Consistent labeling reduces noise and ambiguity in the training signal. Versioning enables teams to audit and refine data sets iteratively. Finally, validation helps catch data issues before they degrade model performance.
As an example, a data-centric approach in manufacturing might involve carefully collecting and labeling images of product defects, consistently annotating defect types, and validating that the data set covers all relevant product versions and variations. In healthcare, a multidisciplinary team might be involved in curating patient records with consistent codes, ensuring demographic and clinical diversity, and regularly updating the data set as new data becomes available.
Beyond the model
The shift toward data-centric AI has implications beyond just model performance. The aim is to address AI's most pressing challenges today: reliability, transparency and accountability.
Resilient and reliable AI systems are critical for high-stakes applications like healthcare and transportation, where safety is paramount. Data-centric practices like comprehensive data validation and continuous monitoring can help ensure AI systems remain safe and effective even when real-world conditions change.
Data-centric AI promotes transparency and accountability -- and therefore trust -- by making data provenance and processing more explicit and auditable. Moreover, data-centric AI intersects with responsible AI practices and AI governance frameworks. Documented data collection, labeling and validation processes all help demonstrate compliance with privacy regulations and AI ethics frameworks. Engaging diverse stakeholders in data curation can surface and mitigate biases.
However, the shift to data-centric AI also raises challenges and questions. Collecting and annotating high-quality data can be resource-intensive; there are reasonable concerns about scalability and accessibility; and balancing data needs with privacy concerns is an ongoing challenge. Aligning data practices across organizations and domains requires coordination and standardization. Yet these challenges must be met to build more robust, responsible and effective AI systems.
Donald Farmer is the principal of TreeHive Strategy, which advises software vendors, enterprises and investors on data and advanced analytics strategy. He has worked on some of the leading data technologies in the market and in award-winning startups. He previously led design and innovation teams at Microsoft and Qlik.