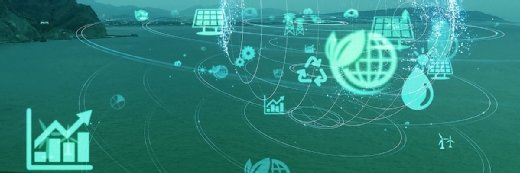
Getty Images/iStockphoto
Best practices for integrating AI and ESG strategies
Integrating environmental, social and governance goals into AI models can help organizations achieve more ethical, responsible and sustainable business outcomes.
Adopting an environmental, social and governance framework can help businesses address the climate and ethical challenges that AI presents.
ESG refers to a framework or set of criteria designed to measure the effects of an organization's actions on the environment, society and its constituents. The ESG approach seeks to address several areas:
- Environmental. How does the organization manage its natural resources, reduce its carbon footprint, mitigate environmental risks and contribute to the fight against climate change?
- Social. How does the organization treat its employees, customers, suppliers and communities? How does it promote diversity, inclusion, human rights and social responsibility?
- Governance. How does the organization conduct its business; ensure accountability, transparency and compliance; and address ethical dilemmas and conflicts of interest?
ESG can also have a significant positive effect on an organization's long-term performance, reputation and value creation. Following an ESG framework can enhance competitive advantage and differentiation in the market by attracting and retaining customers, investors, employees and partners who value sustainability and ethics. In addition, it can improve operational efficiency and innovation by reducing costs, risks and waste, and fostering a culture of collaboration.
Moreover, an ESG framework can strengthen customer and constituent relationships and trust through thoughtful, relevant engagement. It can help organizations solicit feedback, address customer concerns and set expectations. Internal ESG guidelines also assist organizations in fulfilling their social and environmental obligations and commitments. Guidelines can ensure that organizations comply with relevant laws and regulations and that they contribute to global goals and initiatives, such as the United Nations Sustainable Development Goals.
To ensure that ESG delivers more meaningful, relevant, responsible and ethical outcomes, organizations must identify the variables that constitute their ESG aspirations. These variables should be integrated into an AI utility function that guides the actions and decisions of AI models, providing a clear path for achieving ESG goals.
ESG and the AI utility function
An AI utility function consists of the variables, metrics and associated weights that guide an AI model's decisions, map probabilistic outcomes to utility values and measure decision effectiveness, enabling the model to continuously learn and adapt.
The AI utility function is the beating heart of an AI model. The model uses the utility function to guide its decisions and actions by comparing the expected values of different outcomes and choosing the action or decision that maximizes the expected value, based on the variables and their associated weights.
Creating an ESG-friendly AI utility function requires integrating several types of ESG measures into the model's algorithmic architecture:
- Ethical metrics, such as charitable contributions, volunteering hours, pay equality, corporate social responsibility reporting and individual privacy protections.
- Environmental metrics, such as energy efficiency, carbon footprint, water usage, biodiversity impact and renewable energy usage.
- Social metrics, such as workforce diversity, equal employment opportunities, healthcare affordability, workplace safety and human rights adherence.
Challenges of ESG from an AI perspective
Identifying and prioritizing the ESG measures to integrate into the AI utility function is a complex process involving organizational and technical challenges.
A key organizational challenge is creating a culture of data literacy and data-driven decision-making, where the entire organization values, communicates and aligns with ESG measures. Collaborating across multiple stakeholders -- including senior leaders, business units, customers, employees and regulators -- is essential to define and validate the ESG variables and their weights in the AI utility function.
In addition, organizations must establish clear and measurable ESG goals and metrics that align with the business strategy. This includes balancing tradeoffs and conflicts among ESG variables and other criteria, such as profitability, efficiency and quality, as well as ensuring the accountability, transparency and compliance of AI models and their outcomes.
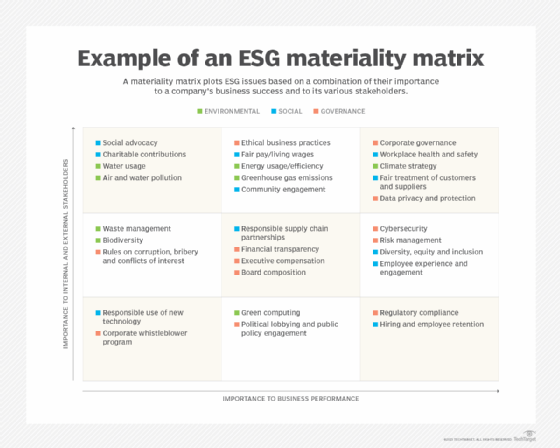
On the technical side, challenges include collecting, preparing, cleaning, aggregating, integrating and analyzing ESG data from a wide variety of internal and external data sources. Developing AI models that incorporate ESG variables and their natural tradeoffs into the utility function is another potential hurdle. Finally, organizations must monitor and evaluate the effectiveness of the AI models on the ESG measures and update the utility function accordingly.
AI use cases in ESG
Using AI to enhance ESG reporting can have beneficial outcomes in a variety of sectors:
- Inventory and supply chain management. Incorporating ESG variables such as greenhouse gas emissions, water consumption and recycling rate into the AI utility function can help organizations minimize their carbon footprint and waste generation.
- Healthcare. Providers can use AI to improve patient care and outcomes while enhancing social responsibility and reputation. ESG variables to incorporate could include patient satisfaction, quality of care and ethical standards.
- Banking and finance. Financial institutions can use AI to enhance risk management and fraud detection, and can use variables such as compliance, accountability and trust to promote governance and transparency.
- Manufacturing. Manufacturers that use AI to optimize product design and development can improve environmental and social impact by incorporating ESG variables such as energy efficiency, material usage and waste reduction.
- Media. Media companies can use AI to personalize content and recommendations while ensuring diversity and inclusion by incorporating ESG variables such as representation, accessibility, quality and relevance.
- Nonprofits. A nonprofit organization that uses AI to enhance its fundraising and advocacy can advance its mission and vision by incorporating ESG variables such as impact, awareness, engagement and trust.
Bill Schmarzo is the former CIO of Hitachi Vantara, where he was recognized for groundbreaking work in data science and automated machine learning. He has also worked at Dell EMC as CTO and as vice president of analytics at Yahoo. He has also written several books on big data.