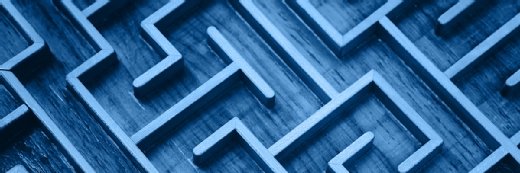
Getty Images/iStockphoto
Why and how to develop a set of responsible AI principles
Enterprise AI use raises a range of pressing ethical issues. Learn why responsible AI principles matter and explore best practices for enterprises developing an AI framework.
Nearly every industry has an opportunity to benefit from the transformative possibilities of AI. However, its full value can only be realized if common-sense principles and responsible use guidelines are an integral part of the organization's value strategy.
With responsible AI principles, businesses can ensure their implementation and use of AI is legally compliant, has a governance foundation, and proactively takes into account possible impacts on the organization's employees, customers and society as a whole.
Because AI implementations are context-specific based on the domain or industry in which an organization operates, responsible AI will look different across different organizations. For instance, a healthcare organization will emphasize a different set of considerations than a law firm. That said, responsible AI principles serve to create a universal set of priorities and a common language across technology, business and compliance.
Establishing this "North Star" encourages different functions and departments to rally around common goals and dive deeper into the implications of applying those principles across the business. For example, a principle focused on identifying and mitigating AI bias could lead to broader questions on model development and output, types and sources of training data, and the need for diverse and meaningful human oversight.
On the flip side, the absence of shared principles could mean that some of these questions are asked in silos or that answers lack crucial insight from colleagues with relevant expertise. It could also lead to conflicting business priorities or decisions, and duplication of work. This could have more serious implications, such as negligent use or increased exposure to cybersecurity risks.
These risks will only increase with the rampant adoption of generative AI technologies. Even as generative AI tools such as ChatGPT have taken the world by storm, questions and doubts are becoming more numerous and prominent and cannot be ignored. Some of the most common concerns about generative AI revolve around the following:
- Unreliable outputs, such as plausible-sounding "hallucinations."
- Lack of transparency, particularly around model training, explainable outputs and related risks such as bias.
- Potential misuse, such as copyright infringement, deepfakes and cyber attacks.
While we're far from a scenario where "rogue AI" takes over the world, failing to implement responsible AI principles and good tech governance has serious implications. Already there are several examples that highlight the severe consequences of inappropriate uses of AI.
Recently, in the legal industry, ChatGPT completely fabricated the case law and citations included in a court filing -- and the chatbot's responses convinced the unsuspecting lawyer that its output was 100% accurate and factual. Other notable examples include the recidivism prediction tool COMPAS, which an investigation found to be racially biased, and an Amazon AI-powered recruitment tool that learned to discriminate against women.
No technology is perfect, but the rapid evolution and adoption of generative AI forces businesses to make decisions about a technology whose capabilities they might not yet fully comprehend. Implementing a set of AI principles is a healthy starting point. However, these principles will only deliver value if a company is committed to putting them into practice.
Tips for developing a responsible AI framework
Responsible AI is a journey. Here are some pointers to help businesses develop and operationalize their principles.
1. Do your research
Read up on what is generally considered responsible practice across international organizations, and follow industry developments and regulatory trends. The field is continuously evolving, but you are likely to come across recurring themes that merit consideration in addition to domain-specific concerns. Bias and transparency are prominent current examples; the environmental impact of training AI models is another.
2. Don't reinvent the wheel
Start with your existing corporate values, responsibility commitments and policies, and build from there. What positive impact do you want AI to create for employees, customers and society? Are there any risks you want to address? Everything should flow from your stated purpose.
3. Collaborate and co-create
Focus on principles that affect your teams first, ensuring the wording and spirit resonate with those who must adopt them into their working practices. Collect insights from colleagues across different parts of the business -- including management, IT, product and legal -- through conversations, reviews and workshops.
Iterate principles development to include feedback from these key stakeholders. Ask questions such as whether the proposed principles make sense, whether they enhance or protect the organization, how they could be applied, what could go wrong and what additional guardrails make sense. This will help identify opportunities and risks and can clarify what is and isn't acceptable to the business. Incorporating different perspectives and diverse life experiences into these conversations will also deliver more insightful feedback.
4. Embed and operationalize
The next -- and hardest -- step is to embed these principles into daily decision-making and execution. This will take time and some experimentation, but the key is to identify what working practices and governance mechanisms can be used -- and potentially improved along the way -- instead of creating parallel workstreams.
Map out and prioritize focus areas, such as transparency or eliminating bias. Instead of "boiling the ocean," establish a key priority that provides an anchor for other responsible AI principles. Focus on tangible practices and outcomes. That might sometimes mean small steps, such as refining existing documentation practices and iterating as you accumulate experience. The goal is continuous improvement and momentum.
Partnering, both internally and with vendors and customers, is crucial to success. Collaboration enables you to take advantage of relevant areas of expertise, avoid duplication and innovate through diversity of thought. Along the way, you'll build relationships, become more resilient in the face of change and get everyone on board. For example, bring privacy and security colleagues into the conversation early, and share responsible AI challenges with relevant vendors.
Finally, don't wait until you have the perfect process in place before taking action. Be prepared to review and iterate on your approach until you find the right fit, then review and iterate again.
Find your North Star
Responsible AI principles are not the answer to all your AI technology challenges. But when such principles are established thoughtfully, they act as a North Star for business decision-making. They can guide decisions on why, where and how to develop or implement AI tools; how to govern their use; and how to best apply them to improve the business, the lives of employees and customers, and perhaps society as a whole.
About the author
Emili Budell-Rhodes is a purpose-driven innovator passionate about creating inclusive, responsible tech, specializing in analytics and AI. As lead evangelist for LexisNexis Engineering Culture, she promotes culture change where technology drives the rule of law around the world, focusing on promoting and refining the enterprise-level technology strategy as an enabler for empowered and autonomous teams globally. Budell-Rhodes led the development of the RELX Responsible AI Principles.