Moving beyond NLP to make chatbots smarter
Machine reasoning could help chatbots better understand context, which is crucial to understanding human emotions and formulating emotionally relevant responses.
Organizations have used chatbots for decades to address a wide range of needs, from customer inquiries to providing automated interactions of all sorts. These conversational assistants have proven their value by enabling people to interact with machines in their natural language rather than navigating a website or waiting on hold in customer call centers.
Yet, people are demanding more from their chatbot interactions. They want chatbots to answer more complex questions and complete more complicated interactions that aren't easy to script or plan. Those enhanced capabilities may be possible through advancements in natural language processing (NLP).
NLP encompasses natural language understanding (NLU), the ability for computers to understand human conversational language, and natural language generation (NLG), the ability for machines to respond in kind back to their human interactions. Together, NLG and NLU give machines the ability to interpret natural language data and respond in natural language.
However, NLP is still limited in terms of what the computer can understand, and smarter systems require more development in critical areas.
Computers still have a hard time understanding emotion, reasoning and logic, regional speech patterns, slang, colloquialism, industry-specific terminology, and a wide range of expressions and speech patterns that require common sense or implicit knowledge. For chatbots to obtain this level of understanding, they need to adopt more advanced forms of NLP that take advantage of the recent surge in research and funding in AI and machine learning.
What makes chatbots useful in the enterprise?
The internet has opened the door to connect customers and enterprises while also challenging traditional business concepts, such as hours of operations or locality.
No matter where they are, customers can connect with an enterprise's autonomous conversational agents at any hour of the day. Chatbots can converse with users, keep a consistently positive tone and effectively handle a wide range of user needs. By using conversational agents, businesses can offer chat on their websites without growing their customer service teams or dramatically increasing costs.
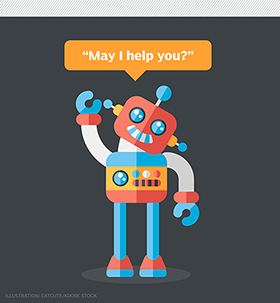
Chatbots can also increase customer satisfaction by providing customers with low-friction channels as their point of contact with the company. In this world of instant everything, people have become less patient with dialing up companies to answer various questions. Customers are often frustrated navigating through an interactive voice response (IVR) system, only to be put on hold for an extended period, before speaking to a human support rep.
According to a Facebook-commissioned study by Nielsen, 56% of respondents would rather message a business than call customer service. Chatbots create an opportunity for companies to have more instant interactions, providing customers with their preferred mode of interaction. This creates less customer friction and higher levels of customer satisfaction.
Enterprises also integrate chatbots with popular messaging platforms, including Facebook and Slack. Businesses understand that customers want to reach them in the same way they reach out to everyone else in their lives. Companies must provide their customers with opportunities to contact them through familiar channels.
Additionally, enterprises can use these integrated chatbots internally to help manage and keep track of conversations, or provide the ability to log work efficiently without having employees go into multiple systems. Integrated chatbots also enable easier collaboration between teams, especially in the current remote and work-from-home environment.
Conversational assistants represent a paradigm shift in how businesses and organizations communicate with their customers and provide tremendous value to enterprises.
Enhancing NLP with emotional intelligence, machine reasoning and common sense
Systems need to understand human emotions to unlock the true potential of conversational AI. While businesses can program and train them to understand the meaning of specific keywords at a high level, the systems can't inherently understand emotion.
A voice-based system might log that a user is crying, for example, but it wouldn't understand if the user is crying because they are sad or happy.
To move up the ladder to human levels of understanding, chatbots and voice assistants will need to understand human emotions and formulate emotionally relevant responses. This is an exceedingly difficult problem to solve, but it's a crucial step in making chatbots more intelligent.
Similarly, current NLP systems have trouble understanding context. They lack the common sense that people take for granted. For example, a person might inherently know that a natural disaster will force businesses in the area to close. A machine, meanwhile, would need to be explicitly programmed to know companies are closed in that situation.
Machines with additional abilities to perform machine reasoning using semantic or knowledge-graph-based approaches can respond to such unusual circumstances without requiring the constant rewriting of conversational intents.
Machine learning is a branch of AI that relies on logical techniques, including deduction and induction, to codify relationships between information. This could enable a chatbot to understand context better.
Likewise, machines that use AI for pattern and anomaly detection, predictive analytics and hyper-personalization can make their conversational systems more intelligent. Rather than relying on an isolated conversational interaction, these systems can pull data from existing customer history or interactions to gain more context for the overall conversation, resulting in more satisfied users who know that they don't have to start each conversation from scratch.