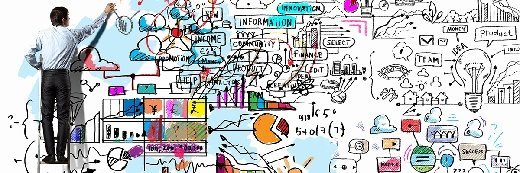
Sergey Nivens - Fotolia
Avoid bias in algorithms for best AI results
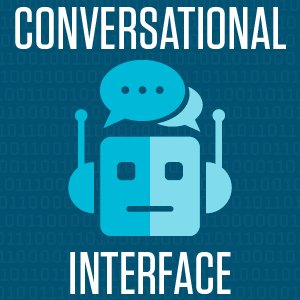
In this podcast, we examine leading thoughts on the problem of AI bias and how to mitigate some of the most common sources of unfair treatment of users of AI applications.
Enterprises are waking up to the potential risks of bias in algorithms and how it can impact AI applications -- and for good reason.
Several prominent companies suffered black eyes when their AI tools went astray, including Microsoft, whose chatbot, Tay, quickly learned sexism and antisemitism, and Google, whose image classification tool once categorized a photo of two black people as gorillas.
But while enterprises are seeing the potential risks of implementing biased AI tools, the steps to avoid these kinds of biases in algorithms are less clear. In this edition of the Conversational Interface podcast, we look at some emerging thoughts on the topic of bias in AI applications.
Several speakers at the O'Reilly Media Artificial Intelligence Conference in New York took on the topic.
Lindsey Zuloaga, director of data science at AI-powered recruiting service HireVue, said a lot of what we call bias in AI applications is really just the application reflecting societal biases. To address these types of inequities, it's not enough to look at an application's recommendations and judge them as fair or biased. Instead, developers need to think about the desired outcomes and optimize for those conditions.
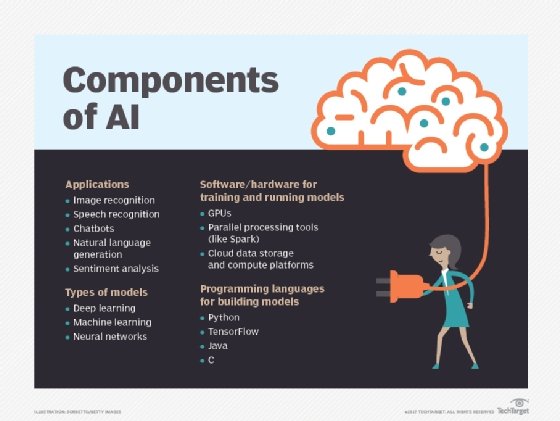
In the realm of image recognition tools, Stephanie Kim, developer evangelist with AI platform Algorithmia, said representative training data is the key to keeping applications balanced. Too often, developers train models on their own faces, which becomes problematic when teams aren't diverse.
Listen to the podcast to hear more recommendations on how to recognize and remedy bias in algorithms for AI applications.
Bridget Botelho, editorial director for the business applications and information management group, contributed to this podcast.