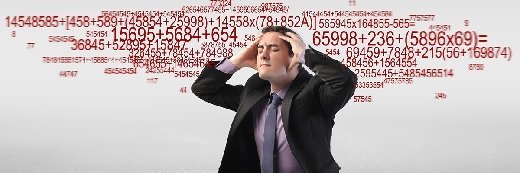
olly - Fotolia
Mathematician warns against weapons of 'math' destruction
In this SearchCIO Q&A, author and data scientist Cathy O'Neil talks about the danger of trusting algorithms too much.
Companies and customers don't spend much time thinking about the negative side effects of data science, but they may want to start. In her new book, Weapons of Math Destruction, Cathy O'Neil explains that just because an algorithm exists, it doesn't mean it's a good algorithm. Many of the algorithms that control our lives are flawed and need to be debugged, like any software.
O'Neil, a data scientist, isn't talking about the algorithms that are designed to trick people, such as the Volkswagen emissions algorithm. Instead, she's talking about algorithms that may lead businesses and governments to draw erroneous, biased and even harmful conclusions about customers and constituents.
O'Neil, who will be speaking at the upcoming Real Business Intelligence Conference in Cambridge, Mass., sat down with SearchCIO to talk about why algorithms go bad. This Q&A has been edited for brevity and clarity.
You've called algorithms that have the potential do real harm 'weapons of math destruction.' How do you define WMDs?
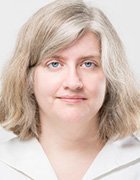
O'Neil: They're defined by their characteristics. There are three characteristics for WMDs: They're very important; they're widespread and are used on a lot of people for important decisions. They're secret; people don't understand how they're being scored, and sometimes they don't even understand that they're being scored. They're destructive on the individual level.
So, people are scored in important ways, in secret ways and they're unfairly being prevented from having options in their life.
What's an example of a WMD that's out there in the wild?
O'Neil: There's a couple of examples -- one in Michigan and one in Australia -- of automated systems used to hand out disability checks. But the system's fraud detection, which tried to detect whether somebody was claiming a disability fraudulently, was way too sensitive.
So, it was denying people their disability checks and actually accusing them of fraud and, essentially, sending them bills. This happened to tons of people before it was discovered and corrected.
How do companies get to a point where they can be confident the algorithms they're using aren't biased to such a degree?
O'Neil: For some reason, people separate out mathematical algorithms from other kinds of processes. So, in other words, if you were working in the unemployment office in Michigan and you were told, 'Hey, we have a new system that's going to be turned on tomorrow, and nobody understands it,' you would think everybody would keep a close eye on it to make sure it works. But for whatever reason, when it's algorithmic, people think, 'Oh, well, really smart people built this, so it's got to work.'
So, I don't really have a very good explanation of it, except that people just trust mathematical algorithms too much. One of my major goals is a call for science and a warning away from blind trust. When I say science, what I mean is that I want evidence this works; I want evidence this is fair; I want evidence that the people who you're claiming are trying to defraud the system are actually trying to defraud the system, that you have a good accuracy rate, you have a low false positive rate, you have a low false negative rate. And, when you do have false positives, I want to make sure that they're not all falling on certain populations unfairly.
Another example is the COMPAS [Correctional Offender Management Profiling for Alternative Sanctions] recidivism model. The recidivism risk algorithms are used by judges in sentencing, and, depending on how high the risk for recidivism, which is to say the risk of returning to prison, a judge will tend to sentence somebody longer if they have a high risk of recidivism.
What was found by ProPublica when they looked into a specific recidivism risk algorithm being used in Florida was that the false positive rate for the African-Americans was twice the false positive rate for the whites, which is to say you would have more African-American defendants going to prison for longer than whites. And that's a problem. There was not enough evidence that that algorithm was working before it started getting used.
Is that due to a bad algorithm or bad data?
Cathy O'Neil's 2016 Talks at
Google on her book 'Weapons of
Math Destruction.'
O'Neil: I would argue that, in this case, the data is bad. And the data's bad for a very human reason, which is that we tend to find crimes committed by African-Americans more often than we find crimes committed by white people. ... When you have data coming in that's telling you that the criminals are much more likely to be African-American -- if it's true or not -- the algorithm isn't going to know that this is a [biased] police practice record rather than a criminality statistic.
Are machine learning algorithms -- or algorithms that teach themselves -- more or less likely to be weapons of math destruction?
O'Neil: They're more likely because we don't really understand them. When you say they 'teach themselves,' it means we don't explicitly tell the algorithm how to interpret the data it's seeing. So, it interprets it in some kind of opaque way that we don't understand and can't explain.
That's already a bad sign. And the other thing that's bad is that people trust deep learning, neural network stuff even more than they trust other kinds of statistical methods. It's, again, the blind trust problem.
And, I should add, the people who build the models -- mathematicians, statisticians, computer scientists -- they also blindly trust the algorithms they build. They are not asked to understand what can go wrong, and they don't consider it very much. I don't think it's a coincidence that the people who built them are typically not the populations that suffer from them. There's a real disconnect between the machine learning community and the people who actually have the misfortune to be incorrectly scored.
In the second part of this two-part Q&A, O'Neil suggests it might be time for a national safety board for algorithms.