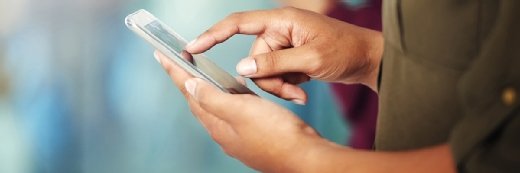
Getty Images
Microsoft's new Phi-3-mini AI language model runs on iPhone
Microsoft researchers contend the Phi-3-mini's performance is on par with the much larger ChatGPT 3.5 model and can run on an iPhone 14 powered by an A16 Bionic chip.
Microsoft's latest small language model shows how the technology is advancing as enterprises evaluate running generative AI models in-house to drive efficiencies in business operations.
This week, Microsoft launched Phi-3-mini, the first of three small language models (SLM) coming from the company's research arm. The new model is the smallest of the trio, at 3.8 billion parameters. The upcoming SLMs are Phi-3-small (7 billion parameters) and Phi-3-medium (14 billion parameters).
Phi-3-mini is available on Microsoft's Azure AI Studio model catalog and on the AI developer site Hugging Face. Microsoft plans to add the other two models to the catalog soon.
The expense of using large language models (LLM) of hundreds of billions of parameters on cloud providers AWS, Google and Microsoft has many enterprises evaluating SLMs as a cheaper alternative. Microsoft's Phi project reflects the company's belief that enterprise customers will eventually want many model choices.
"Some customers may only need small models, some will need big models, and many are going to want to combine both in a variety of ways," Luis Vargas, vice president of AI at Microsoft, said in an article posted on the company's website.
Microsoft lists several SLM advancements with the Phi-3-mini. In a technical report, researchers claim its quality "seems on par" with Mistral AI's Mixtral 8x7B, with 45 billion parameters, and OpenAI's ChatGPT 3.5, with about 22 billion parameters.
Also, researchers reported running the Phi-3-mini on an Apple iPhone 14 powered by an A16 Bionic chip. The model used 1.8 GB memory.
Researchers attributed Phi-3-mini's performance to their training methodology. They trained the model on heavily filtered web data from open internet sources and LLM-generated synthetic data. The former infused the model with general knowledge, and the latter trained it for logical reasoning and various niche skills.
Phi-3-mini's uses include providing summaries of long documents or trends within market research reports. Also, marketing and sales departments could use it to write product descriptions or social media posts. Phi-3-mini could also underpin a customer chatbot to answer basic questions about products and services.
Though the Phi-3-mini model achieves a similar level of language understanding as larger models, it is limited in that it lacks the capacity to store as much information as LLMs. In addition, this small model is restricted to English, according to the technical report.
SLMs in the data center
Microsoft and other model providers recognize that LLMs are overkill for many AI tasks that enterprises can run in-house on an AI server in the data center, experts said.
"Model companies are trying to strike the right balance between the performance and size of the models relative to the cost of running them," Gartner analyst Arun Chandrasekaran said.
Ultimately, enterprises will choose from various types of models, including open source and proprietary LLMs and SLMs, Chandrasekaran said. However, choosing the model is only the first step when running AI in-house.
Other steps include picking the tools for monitoring and fine-tuning model output and preventing models from leaking sensitive data. Also, there's the infrastructure cost, including GPU servers and their underlying storage and networking.
"There's a lot of work that you need to do," Chandrasekaran said.
Enterprises running cloud-based models will have the option of using the provider's tools. For example, Microsoft recently introduced GenAI developer tools in Azure AI Studio that detect erroneous model outputs and monitor user inputs and model responses.
Whether the model is in the cloud or data center, enterprises must establish a framework for evaluating the return on investment, experts said.
Antone Gonsalves is an editor at large for TechTarget Editorial, reporting on industry trends critical to enterprise tech buyers. He has worked in tech journalism for 25 years and is based in San Francisco.