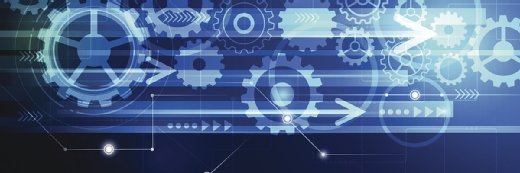
Getty Images/iStockphoto
Google touts AI supercomputer; Nvidia tops MLPerf 3.0 tests
Google added a little more heat to the AI supercomputing wars, revealing the latest details of its upcoming system positioned against that of market leader Nvidia.
The war of words among AI supercomputer vendors escalated this week with Google claiming that its TPU-based system is faster and more efficient than Nvidia's A100-based entry, according to its own testing. Nvidia countered that its H100 system is faster based on testing conducted by the independent MLCommons using MLPerf 3.0.
Google researchers reported that its Tensor Processing Unit-based supercomputer v4 is 1.2 to 1.7 times faster than Nvidia's 3-year-old A100 system and uses between 1.3 to 1.9 times less power. The MLPerf 3.0 benchmarks measured Nvidia's newer H100 against systems entered by 25 organizations, but Google's TPU-based v4 system was not one of them.
A direct system-to-system comparison of the two companies' latest systems would have to be conducted by an independent organization running a variety of AI-based workloads for any benchmarks to be definitive, analysts said.
"This is not so much a story about testing as it is a marketing story," said Dan Newman, chief analyst at Futurum Research and CEO of The Futurum Group. "We're at an inflection point where AI competitors believe if they have it, then flaunt it. But what [Google] especially is doing is reminding users what they have so they aren't ruled out of any AI market early."
Another analyst agreed that each company is using its respective testing results to gain mind share among users for what is shaping up as a battle royal among not just Nvidia and Google, but also Microsoft and AWS in the coming years.
"This move by Google is an attempt to assure users they are not going to rush something to market or do something stupid that makes them look bad," said Jack Gold, president and principal analyst at J.Gold Associates. "Is Google behind, schedule-wise, compared with Nvidia? Yes. Are they behind technology-wise? It's hard to say until their stuff gets delivered and tested."
Google's AI supercomputer
Earlier this week, Google published a scientific paper detailing that it has built a system with more than 4,000 TPUs that are tied together along with custom components capable of running and training AI models. The system has been in use internally since 2020 and has been used to train Google's Palm model, an offering that competes against OpenAI's ChatGPT model. Google has used TPUs for more than 90% of its work on AI training, the company said.
In the paper, the company said custom components included its own optical switches capable of connecting individual machines. These connections figure to play a key role among competitors in the AI supercomputer market because the large language models that fuel technologies such as ChatGPT and Google's Bard are too large to be stored on a single chip, the paper stated.
What further clouds the objectivity of Google's test results is the proprietary nature of TPUs that are specifically enhanced to run Google's AI software, including Bard.
"Google is designing these chips to meet its own needs; it's not so much a general-purpose device," Gold said. "But it's advantageous for them to do so because they save a bunch of money, get better profit margins and increase sales of other offerings to people using their cloud services. Microsoft and AWS are doing the same thing."
Nvidia tops MLPerf testing
Nvidia pointed out that it ran all the MLPerf benchmarks, including the latest networked models that fed the model data to the servers, over a network rather than having the parameters already loaded into the system. The MLPerf results showed that the company's H100 Tensor Core GPUs had the highest performance in every test involving AI inference. The GPUs delivered up to 54% performance gains since last September thanks to a number of new software optimizations, the company said.
In a blog post, Nvidia CEO Jensen Huang said that three years ago, when the company delivered A100, the AI world was dominated only by computer vision, but now "generative AI has arrived."
"This is exactly why we built Hopper, specifically optimized for GPT with the Transformer Engine. Today's MLPerf 3.0 highlights Hopper delivering 4x more performance than A100. The next level of Generative AI requires new AI infrastructure to train large language models with great energy efficiency," Huang said in the blog.
As Editor at Large in TechTarget Editorial's News Group, Ed Scannell is responsible for writing and reporting breaking news, news analysis and features focused on technology issues and trends affecting corporate IT professionals.